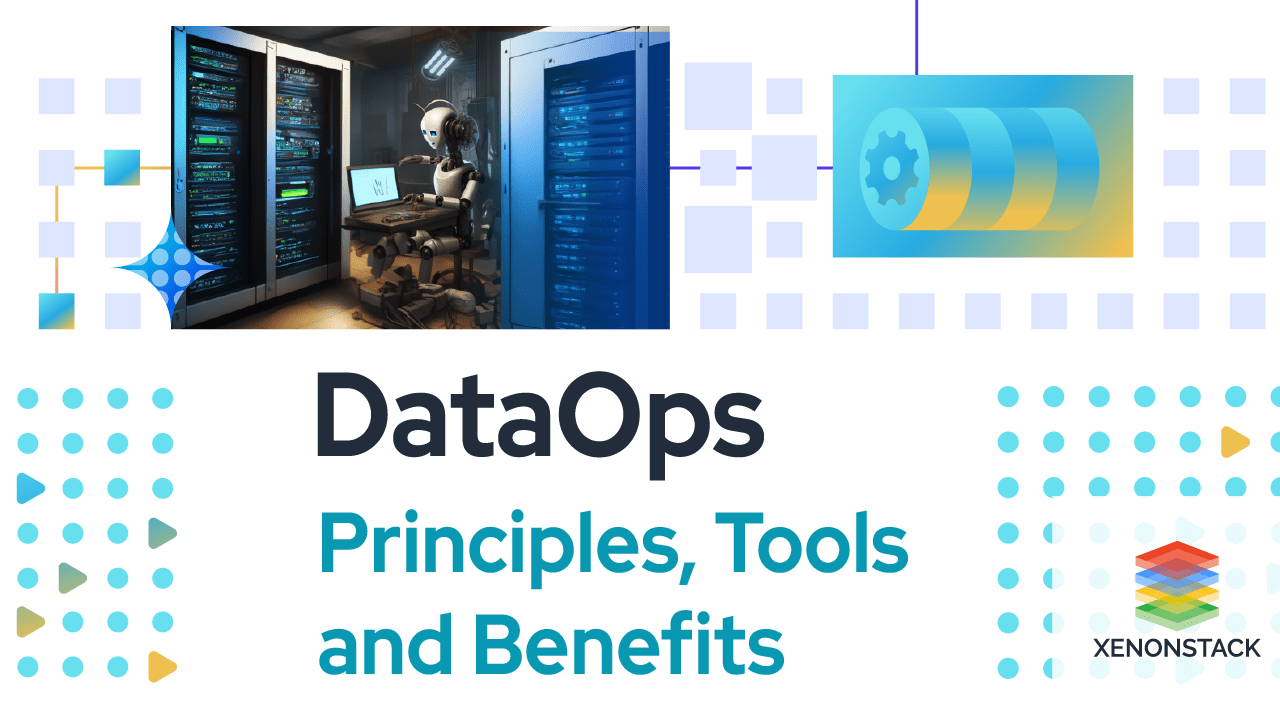
What is DataOps?
DataOps, also known as Data Operations, is a comprehensive system that brings together people, processes, and products to facilitate efficient, automated, and secure management of data. It serves as a vital tool for businesses, as it allows for seamless collaboration and teamwork in various aspects of data handling, including acquisition, storage, processing, monitoring, and delivery. By harnessing the collective skills and expertise of individuals, DataOps contributes to the overall success and growth of an organization.
As a result, the combination of software operations development teams, known as DevOps, is essential. This emerging discipline, comprising engineers and data scientists, emphasizes the collaboration and knowledge-sharing between both groups. By inventing new tools, methodologies, and organizational structures, DevOps aims to enhance the management and protection of the organization. Its primary objective is to optimize the company's IT delivery outcomes by bridging the gap between data consumers and suppliers.
The thought for its concept draws heavily from the source of DevOps, according to which infrastructure and development teams should work together so that projects can be managed efficiently. It focuses on multiple subjects within its field of action, for example, data acquisition and transformation, cleaning, storage, backup scalability, governance, security, predictive analysis, etc.
The pillars of DataOps are:
1. Creating Data Products
2. Aligning Cultures
3. Operationalizing Analytics and Data Science
4. Plan your Analytics and Data Science
5. Harness Structured Methodologies and Processes
What are the Principles of DataOps?
2. Movement/Logging/Provenance.
3. Logica Models.
4. Unified Data Hub.
5. Interoperable (Open, Best of Breed, FOSS & Proprietary).
6. Social (BI Directional, Collaborative, Extreme Distributed Curation).
7. Modern (Hybrid, Service Oriented, Scale-out Architecture).
What are the benefits of DataOps?
The main aim of it is to make the teams capable enough to manage the main processes, which impact the business, interpret the value of each one of them to expel data silos and centralize them even without giving up the ideas that move the organization as one all. It is a growing concept that seeks to balance innovation and management control of the data pipeline. Besides the benefits, it extends across the enterprise. For example:
- Supports the entire software development life cycle and increases DevTest speed by providing fast and consistent environments for the development and test teams.
- Improves quality assurance through the provision of "production-like data" that enables the testing to exercise the test cases effectively before clients encounter errors.
- It helps organizations move safely to the cloud by simplifying and speeding up data migration to the cloud or other destinations.
- Supports both data science and machine learning. Any organization’s data science and artificial intelligence endeavors are as reasonable as the available information. So, it also ensures a reliable flow of the data for digestion and learning.
- Helps with compliance and establishes standardized data security policies and controls for smooth data flow without risking your clients.
Why do we need Dataops?
- It tackles challenges that come while accessing, preparing, integrating, and making data available, also inefficiency while dealing with evolving data.
- It provides better data management and directs better and more available data. More and better data direct better analysis, fostering better insights, business strategies, and higher productivity and profitability.
- DataOps seeks to collaborate between data scientists, data analysts, engineers, and technologists so that every team is working in sync to get data more appropriately and in less time.
- Companies that succeed in taking an agile decision and intended approach to data science are four times more likely than their less data-driven peers to see the growth that exceeds shareholder expectations.
- Many of the services we think of today — Facebook, Netflix, and others — have already adopted these approaches that fall under the DataOps umbrella.
Click to learn Composable Data Processing with a Case study
What DataOps people do?
So, we know what DataOps is, right? Now, we will learn more about what these people do, their responsibility, their actions, and the effect of their actions on other teams.
DataOps (also known as modern data engineering and data operation engineering) is the way of rapid data delivery and improvement in the enterprise (just as DevOps is for the development of the software) by using best practices.
Aims of DataOps people mentioned below:
- DataOps aims to streamline and align the process, including designing, developing, and maintaining applications based on data and data analytics.
- It seeks to improve the way data should be managed and products to be created and coordinate these improvements with the business goals.
- Like DevOps, DataOps follows agile methodology. This approach value includes continuous delivery of analytic insights to satisfy the customer at most.
- To make the most of DataOps(data operation), enterprises must be mature enough in the process of data management strategies to deal with data at scale and in response to real-world events as they happen.
- In effect to DataOps, everything starts with the data consumer and ends with the data consumer because that's who is going to turn data into business value.
- Data preparers, who provide the expository link between data suppliers and data consumers. Data preparers include Data Engineers and ETL professionals empowered with DataOps agile approach and interoperable, best-of-breed tools.
- Data supplier, who is the source owner that provides data. Data suppliers can be any company, organization, individual who wants to make decisions over their data and get insightful information for their data-driven success.
What is the framework of DataOps?
DataOps combines Agile methodologies, DevOps, and lean manufacturing concepts. Let's see how these concepts relate to the DataOps framework.
1. Agile Methodology
This methodology is a commonly used project management principle in software development. Agile development enables data teams to complete analytical tasks in sprints. Applying this principle to his DataOps allows the team to re-evaluate
their priorities after each sprint align with business needs, thus delivering value much faster. This is especially useful in environments with constantly changing requirements.
2. DevOps
DevOps is a set of practices used in software development to shorten the application development and delivery lifecycle to deliver value faster. This includes collaboration between development and IT operations teams to automate software delivery from code to execution. But DevOps involves two technical teams, whereas DataOps involves different technical and business teams, making the process more complex.
3. Lean Manufacturing
Another component of the DataOps framework is lean manufacturing, a way to maximize productivity and minimize waste. It is commonly used in manufacturing operations but can also be applied to data pipelines.
Lean manufacturing allows data engineers to spend less time troubleshooting pipeline issues.
Best Practices for Effective DataOps Framework
Organizations should embrace the best data pipeline and analytics management practices to make the DataOps architecture effective. This includes:
1. Automation
As it enables businesses to streamline operations and lower manual errors, automation is a crucial best practice in DataOps.Automation aids firms in managing the complexity and amount of big data and scaling their data operations.
Get a deep knowledge of automation.
2. Implementing continuous integration and delivery procedures
A critical best practice in DataOps, continuous integration and delivery (CI/CD) enables enterprises to test and apply improvements to their data operations rapidly and efficiently.
CI/CD enables teams to make data-driven decisions more quickly while also assisting organizations in reducing errors and improving data quality.
3. Managing Data Quality Metrics
Data quality management is another practice in DataOps, as it ensures that data is accurate, complete, and relevant for decision-making and analysis. Data quality management helps organizations to improve data quality, reduce errors, and make more informed data-driven decisions.
4. Data Security
Since extensive data often contains sensitive and private information, data security is an essential best practice in big data operations. Data security enables businesses to safeguard their information against illegal access, modification, and misuse while ensuring that data is maintained and used per organizational, legal, and regulatory standards.
Research in-depth about Data Security.
The AWS DataOps Development Kit is an open source development framework for customers that build data workflows and modern data architecture on AWS.
What is the Architecture of DataOps?
DataOps is an approach to data management that emphasizes collaboration, automation, and the continuous delivery of high-quality data to stakeholders. A DataOps architecture typically includes the following components:
1. Data Ingestion
This component involves collecting data from various sources such as databases, APIs, and streaming platforms. Data acquisition can be done by batch processing or real-time streaming.
Get more knowledge on Data Ingestion.
2. Data Processing
After data is ingested, it must be transformed into a form that can be analyzed. This component includes data cleaning, filtering, aggregation, and enrichment.
Analysis in depth on Data Processing.
3. Data Storage
After processing, data should be stored for efficient retrieval and analysis. Data storage can be done using various technologies, such as data warehouses, data lakes, and NoSQL databases.
4. Data Quality
This component includes ensuring data accuracy, completeness, consistency, and timeliness. Data quality can be maintained through profiling, validation, and monitoring.
Learn more about data quality management.
5. Data Governance
Data governance involves establishing policies, procedures, and standards for data management. This component includes data security, privacy, and regulatory compliance.
6. Data Analysis
The final component involves analyzing data to generate insights and support decision-making. This can be done using various analytical tools and techniques such as data visualization, machine learning, and statistical analysis.
Overall, the DataOps architecture emphasizes a collaborative and automated approach to data management that ensures timely and efficient delivery of high-quality data to stakeholders.
Example of Dataops
All these medley-skilled, separately tasked, and individually motivated people must coordinate under a skilled conductor (DataOps/modern data engineering and the CDO) who will earn unified and mission-oriented data to the business at scale and accuracy.
Skills to get started with DataOps
Here we can talk about how you can start and skill that will help for your DataOps success. Understanding this skill set can speed up your journey with DataOps. These are basic and must for starting with DataOps.
-
Basic architecture/data pipelines
From ingestion to storage to presentation layers/production processes/automation of analytics: Involves developing data pipelines and automation of pipeline executions.
-
Database / SQL / Data Marts / Data Models / (also Hadoop / Spark / NoSQL - if those platforms are in the project)/ Data Management system
It involves the storage of your data, accessing data, managing, and handling of data, data governance, and uses of data catalog find the data that they need.
-
ETL / Data APIs / Data-movement / Kafka
It involves moving and transforming data from your sources to your targets and accessing data through APIs for different applications.
-
Cloud Platforms (any of the popular ones) / Networks / Servers / Containers / Docker / Bash / CLI / Amazon Web Services/Redshift (for data warehousing)
It's a lot of fun building models on our laptops, but to build real-world models, most companies are going to need cloud-like power for which one should have a good understanding of these skill sets.
-
Data Manipulation and Preparation / Python, Java, and Scala programming languages
Programming languages understanding must build the pipeline, manipulate, and prepare the data.
-
Understanding the basics of distributed systems / Analytics tools
Hadoop is one of the most used tools for distributed file storage. The Apache Hadoop software library is the framework that makes use of a distributed file system for large data processing across the cluster of computers using a simple programming model, on the other hand, the spark is a unified analytics engine for large-scale data processing.
-
Knowledge of algorithms and data structures
Data engineers mainly focus on data filtering, data preparation, and data optimization. Having a basic knowledge of algorithms can boost your understanding to visualize the big picture of the organization's overall data function and define checkpoints and end goals for the business problem at hand.
-
Versioning tools
A git-like version control system designed to handle everything from small to very large projects with speed and efficiency.
Other important core adroitness includes knowledge of logical and physical data models, data-loading best practices, orchestration of processes and containers.DataOps practices revolve around Python skills. But understanding R, scala,Lambdas, Git, Jenkins, IDE, and other containers would be "a big boost to standardizing and automating the pipeline."
Hence, now you know what DataOps, how they help and speedup data pipelines delivery, best practices that they follow to have better data management and deals with big data in a skillful way, save time and cost, and lead a better data analyst team by saving their time by managing and delivering data to their end.
Benefits of DataOps Platform
A DataOps platform is a centralised area where a team can gather, analyse, and use data to make rational business decisions.Some benefits are :
Improved workforce productivity
DataOps essentially revolves around automation and process-oriented methodologies that increase worker productivity. Employees can concentrate on strategic tasks rather than wasting time on spreadsheet analysis or other menial tasks by integrating testing and observation mechanisms into the analytics pipeline.
Quicker access to business intelligence
DataOps facilitates quicker and simpler access to useful business intelligence. Because DataOps combines the automation of data ingestion, processing, and analytics with eradicating data errors, this agility is made possible. Additionally, DataOps can instantly deliver insightful information about changes in market trends, customer behavior patterns, and volatility.
An easy migration process to the cloud
A migrating project to the cloud can greatly benefit from a DataOps approach. The DataOps process maximizes business agility by combining lean manufacturing, agile development, and DevOps. Workflows on both your on-premises and cloud workplace are automated by a data operations platform. It can assist your enterprise in organizing its data by virtually eliminating errors, shortening product lifecycles, and fostering seamless collaboration between data teams and stakeholders.
What are the Best Practices of DataOps?
1. Versioning2. Self-service
3. Democratize data
4. Platform Approach
5. Be open source
6. Team makeup and Organisation.
7. Unified Stack for all data- historical and Real-Time production.
8. Multi-tenancy and Resource Utilisation.
9. Access Model and Single Security for governance and self-service access.
10. Enterprise-grade for mission-critical applications and Open source tools.
11. Compute on data Stack- leverage data locality.
12. Automation saves time. Thus, automate wherever possible.
What are the latest trends of DataOps?
1. Self-service predictive analytics2. Security and data governance
3. DataOps and Data Mesh
4. Automation and hyper-automation
What are the different DataOps Tools?
Some of the best DataOps tools are:
1. Composable.ai
DataOps is an Analytics-as-a-Service that offers an end-to-end solution for managing data applications. Its low-code development interface allows users to set up data engineering, integrate data in real-time from various sources, and create data-driven products with AI.
2. K2View
To make the customer data easily accessible for analytics, this DataOps tool gathers it from various systems, transforms it, and stores it in a patented Micro-Database. These Micro-Databases are individually compressed and encrypted to improve performance and data security.
3. RightData
The data testing, reconciliation, and validation services offered by this DataOps tool are practical and scalable. Users can create, implement, and automate data reconciliation and validation processes with little programming knowledge to guarantee data quality, reliability, and consistency and prevent compliance issues. Dextrous and RDt are the two services that RightData uses for its tool.
4. Tengu
A low-code DataOps tool called Tengu is made for data experts and non-experts. The business offers services to assist companies in comprehending and maximizing the value of their data. To set up their workflows, Tengu also provides a self-service option for existing data teams. Additionally, users can integrate many tools thanks to its support. Both on-premises and in the cloud are options.
Makes sure data follows both internal and external mandates and data is secure, private, accurate, available, and usable. Taken From Article, Data Governance
How does DataOps help Enterprises build Data-Driven Decisions?
DataOps as a Service combines multi-cloud big-data/data-analytics management and managed services around harnessing and processing the data. Using its components, it provides a scalable, purpose-built big data stack that adheres to best practices in data privacy, security, and governance.
Data Operations as a service means providing real-time data insights. It reduces the cycle time of data science applications and enables better communication and collaboration between teams and team members. Increasing transparency by using data analytics to predict all possible scenarios is necessary. Processes here are built to be reproducible, reuse code whenever possible, and ensure higher data quality. This all leads to the creation of a unified, interoperable data hub.
Enable Deeper Collaborations
As businesses know what data represents, it thus becomes crucial to collaboration between IT and industry. It enables the company to automate the process model operations. Additionally, it adds value to the pipelines by establishing KPIs for the data value chain corresponding to the data pipelines. Thus enabling businesses to form better strategies for their trained models. It brings the organization together in different dimensions. It helps bring localized and centralized development together as a large amount of data analytics development occurs in different corners of enterprises close to business using self-service tools like Excel.
The local teams engaged in distributed analytics creation play a vital role in bringing creative innovations to users, but as said earlier, lack of control pushes you down towards the pitfall of tribal behaviors; thus, centralizing this development under it enables standardized metrics, better data quality, and ensures proper monitoring. Rigidity chokes creativity, but with it, it is easy to move into and for motion between centralized and decentralized development; hence, any concept can be scaled more robustly and efficiently.
Set up Enterprise-level DevOps Ability
Most organizations have completed or are iterating over building Agile and DevOps capabilities. Data Analytics teams should join hands and leverage the enterprise’s Agile and DevOps capabilities to the Latest trends of DataOps.
- The transition from a project-centric approach to a product-centric approach (i.e., geared toward analytical outcomes).
- Establish the Orchestration (from idea to operationalization) pipeline for analytics.
- automated process for Test-Driven Development
- Enable benchmarks and quality controls at every stage of the data value chain.
Automation and Infra Stack
One of its primary services is to scale your infrastructure in an agile and flexible manner to meet ever-changing requirements at scale. Integrating commercial and open-source tools and hosting environments enables the enterprise to automate the process and scale Data and analytics Stack services. For example, it provides infrastructure automation for
- Data warehouses
- BI Stack
- Data lakes
- Data catalogs
- Machine learning infrastructure
Orchestrate Multi-layered Data Architecture
Modern-day AI Led Data stacks are complex with different needs, so it’s essential to align your data strategy with business objectives to support vast data processing and consumption needs. One of the proven design patterns is to set up multi-layered architecture (raw, enriched, reporting, analytics, sandbox, etc.), with each layer having its value and meaning, serving a different purpose and increasing the value over time. Registering data assets across various stages is essential to support and bring out data values by enabling enterprise data discovery initiatives. Enhance and maintain data quality at different layers to build assurance and trust. Protect and secure the data with security standards so providers and consumers can safely access data and insights. Scale services across various engagements and reusable services
Building end-to-end Architecture
Workflow orchestration plays a vital role in binding together the data flow from one layer to another. It helps in the automation and operationalization of the flow. Leverage the modularized capabilities to Key pipelines supported by it are:
- Data Engineering pipelines for batch and real-time data
- Standard services such as data quality and data catalog pipeline
- Machine Learning pipelines for both collection and real-time data
- Monitoring reports and dashboards for both real-time data and batch data
Monitoring and Alerting frameworks Provide provision of building monitoring and alerting frameworks to continuously measure how each pipeline reacts to changes and integrate them with infrastructure to make the right decisions and maintain coding standards.
Know more about DataOps
Companies nowadays invest a lot of money to execute their IT operations better. It is an Agile method that emphasizes interrelated aspects of engineering, integration, and quality of data to speed up the process. Main highlights of this article:- Data Operation focuses on collaborating agility to Machine Learning and Analytics projects.
- Data Operatconnectsnect key data sources right away, unlike Salesforce, customer record data, or what we've identified.
- It simplifies the processes and maintains a continuous delivery of insights.
- DataOps themes: Databases, IoT (Internet of things), Automation, Big data, Machine learning, Smartphones, Blockchain, Cloud, and much more!
Before executing, you are advised to look at its Solutions for Enterprises
- Click here to learn How Data Observability Drives Data Analytics Platforms.
- Explore more about What is a Data Pipeline.
- Importance of Master Data Management in the Banking Sector
- What are the Key Components of DataGovops?