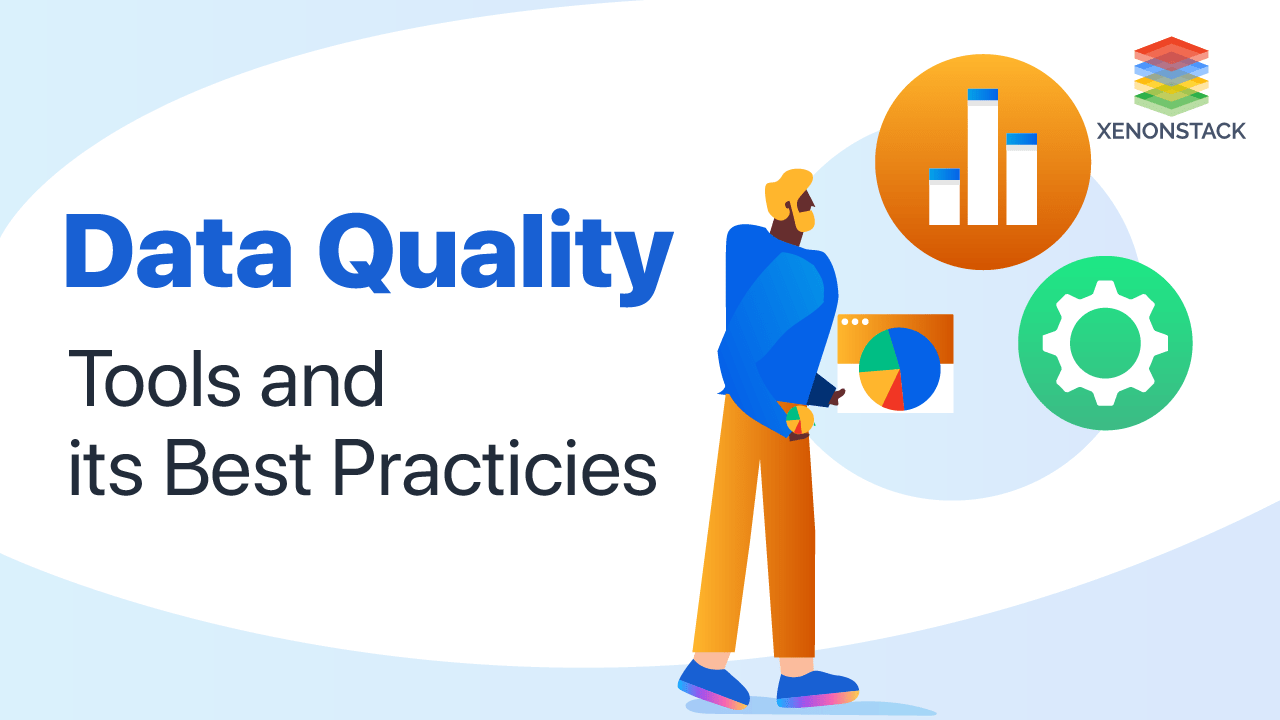
Understanding Data Quality: Definition and Key Concepts
Low-quality data impacts the business process directly. The presence of duplicate data is a severe problem in real-life business. For example, suppose you have it on some logistics-related organization in the dataset. It is connected with products that must be delivered and other associated products that have already been delivered. So if there is the same kind of data present in both datasets, it will trouble to deliver the product that is already delivered.
Late updates to data have a negative effect on data analysis and business processes. In the above example, duplication of it occurs due to not updating it on time.
What is Data Quality?
Data quality is a measurement of the scope of data for the required purpose. It shows the reliability of a given dataset. Its quality of it always impacts the effectiveness and efficiency of any hierarchy. In any order, it acts as a foundation for that. On top of the data, there is information in which it is in context. After that, there is the knowledge that gives the actionable information. And at the top, there comes wisdom.
So if you have low-quality data, you will not have adequate information quality. And if there is no useful information quality, you will be short on the vital information you need for business tasks. High-quality data is always collected and analyzed using a channel of guidelines that ensure the data's accuracy and consistency.
Good quality data is essential because it directly impacts business intuitions. This could be organized information sources like Customer, Supplier, Product, and so forth or unstructured information sources like sensors and logs.
The Importance of Data Quality for Businesses
Without the quality, no one will work with their Business Intelligence Applications because they have data issues, and they will not trust it.
Organizations that successfully render quality and master data management achieve their goals and success as it is the most valuable resource in this world now.
It is significant because, without high quality, you can't understand or remain in contact with your customers. In this data-driven age, it is simpler than any other time to discover key data about current and possible customers. This can enable you to showcase all the more successfully.
High-quality Data is likewise a lot simpler to use than low-quality. Having quality information readily available builds your organization's proficiency too. If your information is incomplete, you need to invest vast amounts of energy in fixing it to make it usable. This removes time from different exercises and means it takes more time to implement the insights that data uncovered.
Quality data also helps keep your company’s various departments on a single page so they can work together more effectively. As in any organization's success, customer relations are crucial, so they provide customer relations to the organization.
Exploring the Architecture of Data Quality Frameworks
The data quality architecture comprises different models, rules, and regulations that supervise which data is collected how the data is stored and, arranged, and used in the organizations. Basically, its role is to make complicated computer database systems that will be useful and secure. It helps define the end-use of a database and then creates a blueprint for testing, developing, and maintaining it. Basically, to implement a simple architecture, there are three main components.
- Workflow manager
- Rule’s Intelligence manager
- Dashboard Manager
The architecture can be implemented with different technologies and tools depending on requirements and what is better for their needs.
Workflow manager
The first component of the architecture is workflow, which is responsible for the data quality workflow and controls the rules and actions that each user has in a process. Even though you can physically develop the workflow, you should prefer tools like Bizagi, which can start up a workflow automatically situated in a BPMN standard. It encourages a great deal since you can import some BPMN modes you made in the process management tool. You can use any tool that goes with a workflow, like Atlassian Jira, which is designed for application
development life cycle management. Jira helps you create and manage customized workflows and has a good cost. But it will be more useful if you use an administration tool like Collibra Data Governance Center; it helps you make and manage the governance operating models in your organization in a scalable and adaptable way.
Rule’s Intelligence manager
Rule’s Intelligence Manager is responsible for changing high-level specifications of data quality rule implemented by Steward in a program code that shows the rule's intelligence kernel. It is also responsible for the generation of data profiling.
You can use the ETL tool if you haven’t any data quality tools. All these tools have a module that is a transformation part that behaves like a tool used the moment you transform the data. This module is responsible for cleansing data before sending it to the destination.
Dashboard manager
The dashboard manager is responsible for creating the dashboard with metrics and KPI, letting you observe the data quality development after some time. And don’t forget to introduce one of the most essential KPI data quality attributes (completeness, consistency, accuracy, duplication, integrity). Create a great dashboard, and nothing better to do with BI and analytical tools.
Strategies to Achieve High Data Quality
Data quality is an important feature whenever we ingest data. Due to the high volume, velocity, and presence of different data kinds, it becomes very challenging to consider it in big data. Quality management system based on machine learning requires hours because they can learn with time and find the anomalies that are not easily discovered. As we have two kinds of it, one is historical data, and the other is real-time. We have different kinds of software to maintain the quality of both types. It is processed using Apache Griffin and AWS Deequ. Apache Griffin is used for Data Quality solutions. It provides a uniform process to predict the quality from different ways to build liable data assets and boost confidence for business requirements.
We have historical data or real-time data. First of all, data scientists define their requirements, such as accuracy, completeness, and much more. After that, it will ingest source data into the Apache Griffin computing cluster and kick off the data quality measurements based on the requirements. Finally, it will remove the reports as measurements of the assigned objective. Then the reports are stored in the open TSDB. From there, we can monitor quality reports using the dashboard.
Core Pillars of Effective Data Quality Management
The quality of data depends upon different attribute the data have, which are as follow:-
- Consistency: Consistency refers to no contradictions in finding the data, no matter where we find it in the database. The number of metrics should be inconsistent.
- Accuracy: The Data you have should be accurate, and the information that contains it should correspond to reality.
- Orderliness: The data should be in the required structure and format. It should follow a proper arrangement order. Imagine having a data set that should be in standard date format.
- Auditability: Data is easily accessible where it is stored in the database, and it is easily possible to trace the changes done.
- Completeness: Data probably consist of different numbers of elements as a part. So the interdependent elements we have in the data have complete information about them so that data can be interpreted correctly.
Overview of Data Quality Management Practices
Data Quality Management generally refers to the business principles that require the combination of the right people, technologies, and processes, all of which have a common goal of improving the measure of data quality that matters most to a hierarchy. The primary purpose of DQM is to enhance the data and achieve business outcomes that directly depend upon high-quality data. The cures used to forestall issues, and inevitable data cleansing includes these orders:-
Data Governance
Data Governance includes what business rules must be stuck to and underpinned by data quality measurement. The information administration system should incorporate the hierarchical structures expected to accomplish the necessary degree of information quality.
Data Profiling
Data profiling is a technique regularly supported by devoted technology used to understand the data resources associated with quality management. It is necessary for people who are engaged to be responsible for quality. Those who are assigned to prevent quality issues and data cleansing have an in-depth knowledge of data.
Master Data Management
Master Data Management and Data Quality Management are firmly coupled with disciplines. MDM and DQM will be a piece of a similar data administration framework and offers the same role as data owners, stewards, and caretakers. Preventing issues in a maintainable manner and not being compelled to dispatch data cleansing activities repeatedly, for most organizations, is an MDM framework that should be set up.
Customer Data Integration
Not at least, customer master data in many organizations are sourced from self-service registration cities, customer relationship management, ERP applications, and perhaps many more. Other than setting up the technical platform for compiling the customer master data from these sources into one source of truth, there is an excellent effort in guaranteeing the data quality of that source of truth. This includes matching and a supportable method of ensuring the correct data completeness, the best data consistency, and satisfactory accuracy.
Product Information Management
As a manufacturer, you just need to adjust your inner information quality with your wholesalers and shippers to make your items stand out so that end clients will choose them as they have a touchpoint in the production network. It ensures the data completeness and other data quality particles inside the item data partnership measures.
Best Practices for Ensuring Data Quality
As low-quality impacts are very severe, it is necessary to learn about inadequate data quality remedies. These are the best way that can improve the quality of data.
Data Quality Always Priority
First of all, it always prioritizes quality. In this step, arrange data quality improvement as a higher priority and ensure every employee understands the difficulties that low-quality data produces. There must be a dashboard to monitor its status of it.
Automatically Entry Of Data
As there is manual entry of data by team members or customers, which is also a reason for low-quality data, organizations should work on automatic entry of the Data that reduces the error that occurs by manual data entry.
Care For Master Data And Metadata
As the master data is essential, but in front of it, you should not forget about the metadata. As the timestamps are revealed by metadata without them, organizations cannot control data versions.
Innovative Data Quality Solutions for Organizations
There are some top-rated solutions, which are as follows:-
- IBM Infosphere Information Server
- Microsoft Data Quality Service
- SAS
IBM Infosphere Information Server
IBM Infosphere Information Server for data quality helps cleanse and maintain quality, helping you turn your data into reliable and trusted data. It provides different tools to understand the data and their relationship, analyze the quality, and maintain data lineage.
Microsoft Data Quality Services
SQL Server Data Quality (DQS) is a very known product that enables you to perform many critical quality operations, including correction, standardization, and data de-duplication. DQS helps perform the data cleansing operation by using cloud-based data services that referred data providers enable.
SAS Data Quality
Whenever the quality of data increases, the value of analytical results increases. SAS data quality software provides improved consistency and integrity to the data. It supports several operations.
Leveraging Deequ for Enhanced Data Quality
Usually, we write unit tests for our code, but we never test our data, and incorrect data always has an enormous impact on the production system. So for the correct Data, amazon uses an open-developed tool Deequ. Deequ provides you with the calculation of quality metrics in a dataset, defining quality constraints. In this, you do not need to implement and verify the algorithms, and you should only focus on describing how your data looks.
Deequ at Amazon
Deequ is used internally at amazon to verify the datasets. Data quality constraints can be added or edited by data set producers. The framework registers information quality measurements consistently (with each new form of a dataset), confirms requirements characterized by dataset makers, and distributes it to customers in the event of progress.
What is Deequ?
Deequ use different kind of components, which are as follow:-
Metrics Computation
Deequ figures information quality measurements that is, insights, for example, completeness, maximum, or correlation. For reading from different sources like amazon s3 and for the computation of metrics with an optimized set of aggregation queries, deequ uses apache spark.
Constraint Verification
As a client, you center around characterizing a bunch of information on quality requirements to be confirmed; Deequ deals with inferring the necessary arrangement of measurements to be registered on the information. Deequ produces an information quality report containing the aftereffect of the required check.
Constraint suggestion
In the deequ, you can decide how to explain your custom information quality requirements or utilize the robotized imperative recommendation strategies that profile the information to surmise practical limitations.
Next Steps Towards Data Quality Management
Talk to our experts about implementing compound AI system, How Industries and different departments use Agentic Workflows and Decision Intelligence to Become Decision Centric. Utilizes AI to automate and optimize IT support and operations, improving efficiency and responsiveness.