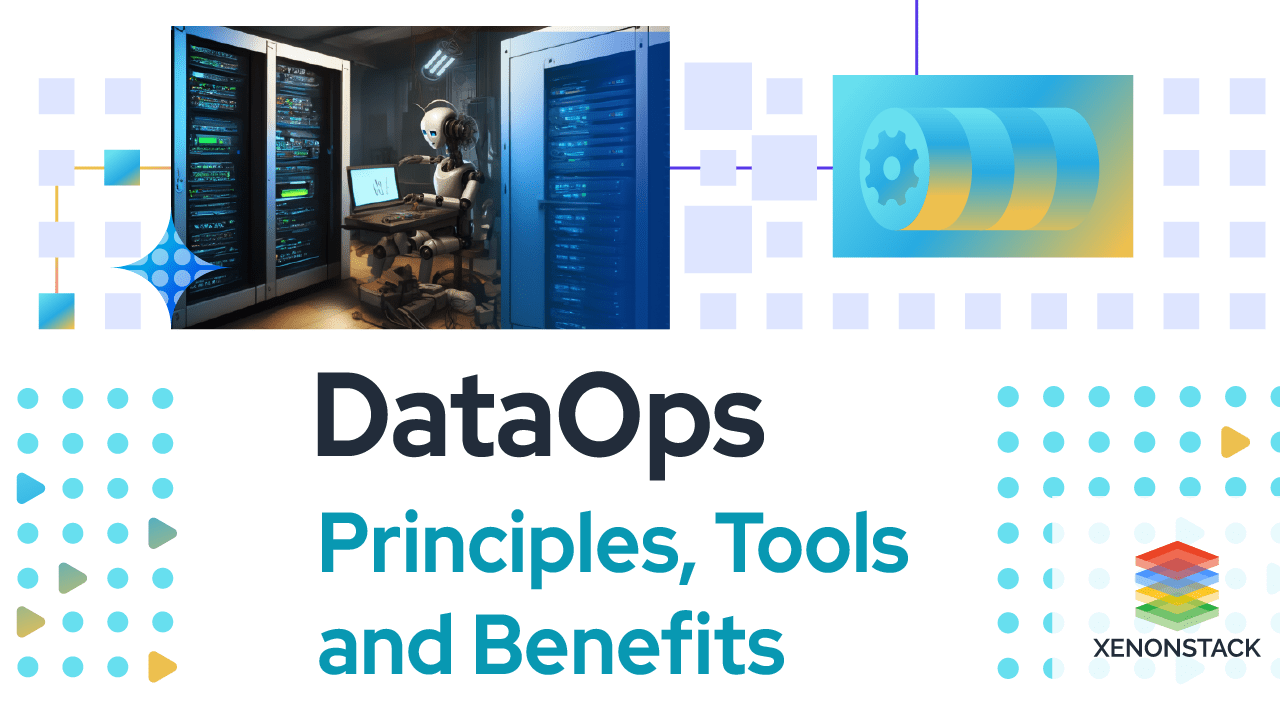
Organisations increasingly rely on seamless data workflows to make informed decisions in today's data-driven world. The ability to manage, process, and monitor vast amounts of data efficiently and accurately has become a critical success factor. This is where DataOps (Data Operations) comes into play—an evolving practice that combines data engineering, quality monitoring, and governance to streamline the entire data lifecycle.
What is DataOps?
DataOps enables organisations to automate and optimise key aspects of their data infrastructure, ensuring data is always accessible, reliable, and aligned with business objectives. Key principles like data quality monitoring, data pipeline orchestration, and data transformation are central to this approach, as they guarantee that data is clean, well-organised, and ready for analysis. Additionally, data governance ensures that your data remains secure, compliant, and auditable across its lifecycle.
As businesses scale, empowering teams with self-service data access becomes increasingly important. DataOps encourages transparency and collaboration by allowing users to access, analyse, and transform data without bottlenecks. At the same time, adopting Continuous Integration and Delivery (CI/CD) principles enables automated, rapid delivery of data updates and changes, fostering innovation while reducing errors and delays. Finally, data observability provides real-time visibility into the health of your data systems, helping you monitor data quality, troubleshoot issues, and improve system performance proactively.
This blog will explore how DataOps can drive efficiency, agility, and quality across your data processes. We'll provide a comprehensive overview of these key practices and how they can be applied in modern data environments.
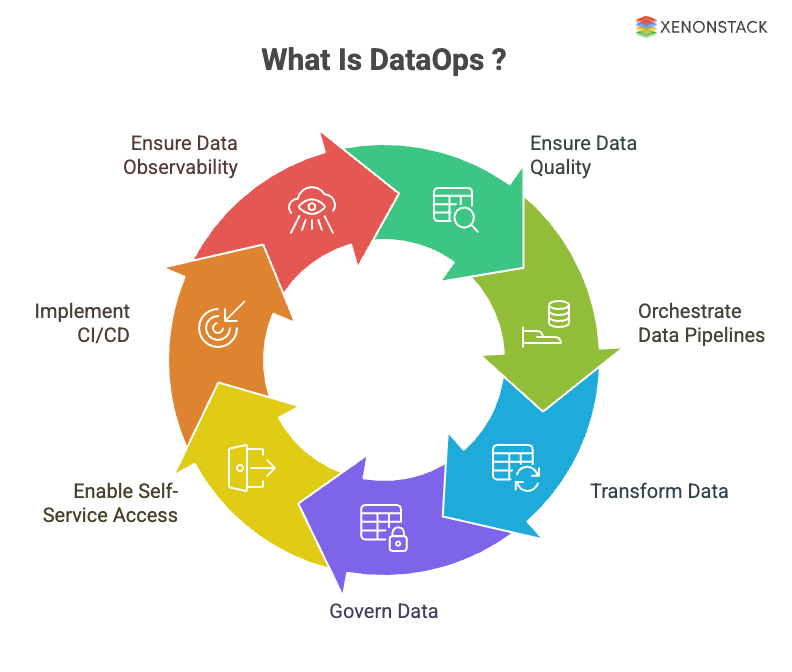
The pillars of DataOps are:
-
Creating Data Products
-
Aligning Cultures
-
Operationalising Analytics and Data Science
-
Plan your Analytics and Data Science
-
Harness Structured Methodologies and Processes
-
Key Principles of DataOps: Enhancing Efficiency, Collaboration, and Security
Predictive Analysis
Predictive analysis uses statistical algorithms and machine learning to forecast future outcomes based on historical data. It helps organisations make data-driven decisions by predicting trends, optimising operations, and enhancing customer experiences.
Error Detection
Error detection in DataOps ensures data accuracy by using automated systems to identify and correct discrepancies. This proactive approach minimises the impact of faulty data on decision-making, improving the reliability of analytics.
Data Democratization
Data democratisation makes data accessible to all employees, enabling non-technical users to explore and use data for decision-making. It promotes innovation, collaboration, and a more data-driven organisational culture.
Automation in DataOps
Automation in DataOps streamlines data management tasks, reducing manual effort and human error. It improves operational efficiency by enabling real-time data processing and accelerating decision-making.
Collaboration in DataOps
Collaboration in DataOps involves cross-functional teams working together to achieve data goals. Effective communication and shared insights foster alignment with business objectives, improving overall data strategies.
Cloud Migration
Cloud migration involves moving data and applications from on-premises to cloud environments. It offers benefits like scalability, cost savings, and enhanced security, enabling advanced analytics and better integration with cloud services.
Data Security Policies
Data security policies protect sensitive data through encryption, access controls, and regular audits. They ensure compliance with regulations and help build trust by safeguarding against breaches and unauthorised access.
Interoperable Systems
Interoperable systems enable seamless integration across different platforms, improving data flow and operational efficiency. In DataOps, they facilitate better collaboration and data sharing, leading to more informed decisions.
Principles of DataOps
1. Raw Source Catalog.2. Movement/Logging/Provenance.
3. Logic Models.
4. Unified Data Hub.
5. Interoperable (Open, Best of Breed, FOSS & Proprietary).
6. Social (BI Directional, Collaborative, Extreme Distributed Curation).
7. Modern (Hybrid, Service-Oriented, Scale-Out Architecture).
Benefits of DataOps
The main aim of it is to make the teams capable enough to manage the main processes, which impact the business, interpret the value of each one of them to expel data silos and centralize them even without giving up the ideas that move the organization as one all. It is a growing concept that seeks to balance innovation and management control of the data pipeline. Besides the benefits, it extends across the enterprise. For example:
-
Supports the entire software development life cycle and increases DevTest speed by providing fast and consistent environments for the development and test teams.
-
Improves quality assurance through the provision of "production-like data" that enables the testing to exercise the test cases effectively before clients encounter errors.
-
It helps organisations move safely to the cloud by simplifying and speeding up data migration to the cloud or other destinations.
-
It supports both data science and machine learning. Any organisation’s data science and artificial intelligence endeavours are as reasonable as the available information, so it also ensures a reliable flow of data for digestion and learning.
-
It helps with compliance and establishes standardised data security policies and controls for smooth data flow without risking your clients.
Why do we need DataOps?
-
It tackles challenges related to accessing, preparing, integrating, and making data available and inefficiencies in dealing with evolving data.
-
It provides better data management and directs better and more available data. More and better data lead to better analysis, fostering better insights, business strategies, and higher productivity and profitability.
-
DataOps seeks to collaborate between data scientists, analysts, engineers, and technologists so that every team works in sync to obtain data more appropriately and in less time.
-
Companies that succeed in making an agile decision and using an intended approach to data science are four times more likely than their less data-driven peers to see growth that exceeds shareholder expectations.
-
Many of the services we think of today — Facebook, Netflix, and others — have already adopted these approaches that fall under the DataOps umbrella.
What do DataOps people do?
So, we know what DataOps is, right? Now, we will learn more about these people, their responsibilities, their actions, and the effect of their actions on other teams.DataOps (also known as modern data engineering and data operation engineering) is a way of rapidly delivering and improving data in the enterprise (just as DevOps is for software development) using best practices.
Aims of DataOps people mentioned below:
-
DataOps aims to streamline and align the process, including designing, developing, and maintaining applications based on data and data analytics.
-
It seeks to improve how data is managed and products are created, and coordinate these improvements with business goals.
-
Like DevOps, DataOps follows an agile methodology. This approach value includes continuous delivery of analytic insights to satisfy the customer.
-
To maximise DataOps(data operation), enterprises must be mature enough in data management strategies to deal with data at scale and respond to real-world events as they happen.
-
In effect, to DataOps, everything starts with and ends with the data consumer because that's who will turn data into business value.
-
Data preparers provide the expository link between data suppliers and data consumers. They include data engineers and ETL professionals empowered by the DataOps agile approach and interoperable, best-of-breed tools.
-
The data supplier is the source owner who provides data. Data suppliers can be any company, organisation, or individual who wants to make decisions based on their data and get insightful information for their data-driven success.
What is the framework of DataOps?
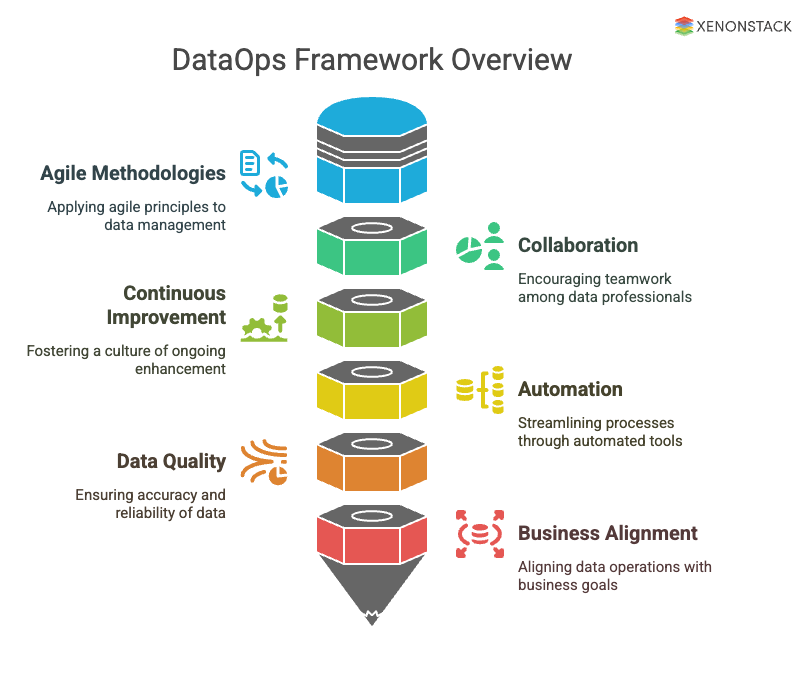
DataOps combines Agile methodologies, DevOps, and lean manufacturing concepts. Let's see how these concepts relate to the DataOps framework.
1. Agile Methodology
This methodology is a commonly used project management principle in software development. Agile development enables data teams to complete analytical tasks in sprints. Applying this principle to his DataOps allows the team to re-evaluate. Their priorities after each sprint align with business needs, thus delivering value much faster. This is especially useful in environments with constantly changing requirements.
2. DevOps
DevOps is a set of practices used in software development to shorten the application development and delivery lifecycle to deliver value faster. This includes collaboration between development and IT operations teams to automate software delivery from code to execution. However, DevOps involves two technical teams, whereas DataOps involves different technical and business teams, making the process more complex.
3. Lean Manufacturing
Another component of the DataOps framework is lean manufacturing, a way to maximise productivity and minimise waste. It is commonly used in manufacturing operations but can also be applied to data pipelines. Lean manufacturing allows data engineers to spend less time troubleshooting pipeline issues.
Best Practices for Effective DataOps Framework
Organisations should embrace the best data pipeline and analytics management practices to make the DataOps architecture effective. This includes:
1. Automation
Automation is a crucial best practice in DataOps. It enables businesses to streamline operations and lower manual errors. Automation aids firms in managing the complexity and amount of big data and scaling their data operations.
2. Implementing continuous integration and delivery procedures
A critical best practice in DataOps, continuous integration and delivery (CI/CD) enables enterprises to test and apply improvements to their data operations rapidly and efficiently. CI/CD enables teams to make data-driven decisions more quickly while also assisting organisations in reducing errors and improving data quality.
3. Managing Data Quality Metrics
Data quality management is another practice in DataOps, as it ensures that data is accurate, complete, and relevant for decision-making and analysis. Data quality management helps organisations to improve data quality, reduce errors, and make more informed data-driven decisions.
4. Data Security
Since extensive data often contains sensitive and private information, data security is an essential best practice in big data operations. Data security enables businesses to safeguard their information against illegal access, modification, and misuse while ensuring that data is maintained and used per organisational, legal, and regulatory standards.
The AWS DataOps Development Kit is an open source development framework for customers that build data workflows and modern data architecture on AWS.
Benefits of DataOps Platform
A DataOps platform is a centralised area where a team can gather, analyse, and use data to make rational business decisions. Some benefits are :
Improved workforce productivity
DataOps revolves around automation and process-oriented methodologies that increase worker productivity. By integrating testing and observation mechanisms into the analytics pipeline, employees can concentrate on strategic tasks rather than wasting time on spreadsheet analysis or other menial tasks.
Quicker access to business intelligence
DataOps facilitates quicker and simpler access to valuable business intelligence. DataOps combines the automation of data ingestion, processing, and analytics with eradicating data errors; this agility is made possible. Additionally, DataOps can instantly deliver insightful information about market trends, customer behaviour patterns, and volatility changes.
An easy migration process to the cloud
A migrating project to the cloud can significantly benefit from a DataOps approach. The DataOps process maximises business agility by combining lean manufacturing, agile development, and DevOps. A data operations platform automates workflows on both your on-premises and cloud workplace. It can assist your enterprise in organising its data by virtually eliminating errors, shortening product lifecycles, and fostering seamless collaboration between data teams and stakeholders.
Use Cases of DataOps
DataOps (Data Operations) isn’t just a methodology—it’s a transformative approach to managing modern data ecosystems with agility, collaboration, and scalability. Below, we explore some of the most impactful DataOps use cases across industries, highlighting how organisations solve complex data challenges with automated, reliable, and continuous data workflows.
1. Real-Time Business Intelligence and Analytics
Organisations today operate in fast-paced environments where up-to-the-minute insights must inform decision-making. DataOps enables the orchestration of real-time data pipelines that ingest, clean, and deliver data from multiple sources—including CRM systems, transactional databases, and external APIS—into analytical platforms such as Tableau, Power BI, or Looker.
Why it matters: Traditional ETL processes can take hours or even days, making insights outdated by the time they're used. With DataOps, data freshness is maintained continuously, empowering teams to act on real-time trends, customer behaviour, and operational metrics.
2. Automated Data Pipelines for Machine Learning Workflows
Due to fragmented data preparation and inconsistent environments, data science teams often face bottlenecks when building and deploying machine learning models. DataOps automates these workflows—ingesting raw data, cleaning and transforming it, generating features, and feeding it into model training pipelines, often using orchestration tools like Apache Airflow, Kubeflow, or MLflow.
Why it matters: By automating and standardising ML data pipelines, DataOps reduces the time spent on repetitive manual tasks, ensures consistency in feature sets, and shortens the cycle between experimentation and deployment.
3. Continuous Data Quality Monitoring
Poor data quality undermines the effectiveness of analytics, reporting, and machine learning models. With DataOps, organisations implement continuous testing frameworks across pipelines to validate data quality metrics at every processing stage, such as completeness, accuracy, consistency, and schema conformance.
Why it matters: Proactively detecting and fixing data issues prevents downstream errors and ensures trust in the data consumed by business users and automated systems.
4. Regulatory Compliance and Governance Automation
Industries like finance, healthcare, and telecom are subject to stringent data privacy and security regulations such as GDPR, HIPAA, and CCPA. DataOps is key in operationalising data governance by automating lineage tracking, metadata management, access controls, and audit logs.
Why it matters: By embedding governance into the pipeline lifecycle, DataOps helps organisations ensure data handling policies are enforced consistently while reducing the risk of non-compliance penalties.
5. Customer 360 Initiatives
Creating a unified, real-time view of the customer is essential for hyper-personalisation and competitive differentiation. DataOps enables the integration of structured and unstructured data across sales, support, marketing, and product touchpoints into a centralised data lake or warehouse.
Why it matters: A seamless Customer 360 view allows organizations to tailor offerings, enhance support, and identify upselling opportunities—leading to improved customer satisfaction and retention.
6. Iot Data Stream Processing
Iot devices generate massive volumes of real-time telemetry in manufacturing, energy, and smart city applications. DataOps frameworks support the ingestion, processing, and storage of high-velocity data using event streaming platforms like Apache Kafka and Spark Streaming.
Why it matters: With DataOps, organizations can implement predictive maintenance, monitor system health, and trigger automated actions with minimal latency—significantly improving operational efficiency and safety.
7. Cloud Data Migration and Integration
As enterprises modernise their infrastructure, migrating legacy systems to cloud-native data platforms (e.g., Snowflake, Databricks, or BigQuery) is a significant initiative. DataOps ensures that migrations are seamless but also repeatable and verifiable, with automated validation checks and rollback mechanisms.
Why it matters: Minimises disruption during migration, ensures data consistency between source and target systems, and accelerates the journey to a scalable, cloud-based data stack.
8. Self-Service Analytics Enablement
Empowering business users with timely access to curated, high-quality data—without IT intervention—is a hallmark of modern data strategy. DataOps facilitates this by creating discoverable data catalogs, governed access controls, and pre-built data products tailored for business needs.
Why it matters: Reduces reliance on engineering teams, promotes data democratisation, and enables data-driven decision-making across all levels of the organisation.
What are the different DataOps Tools?
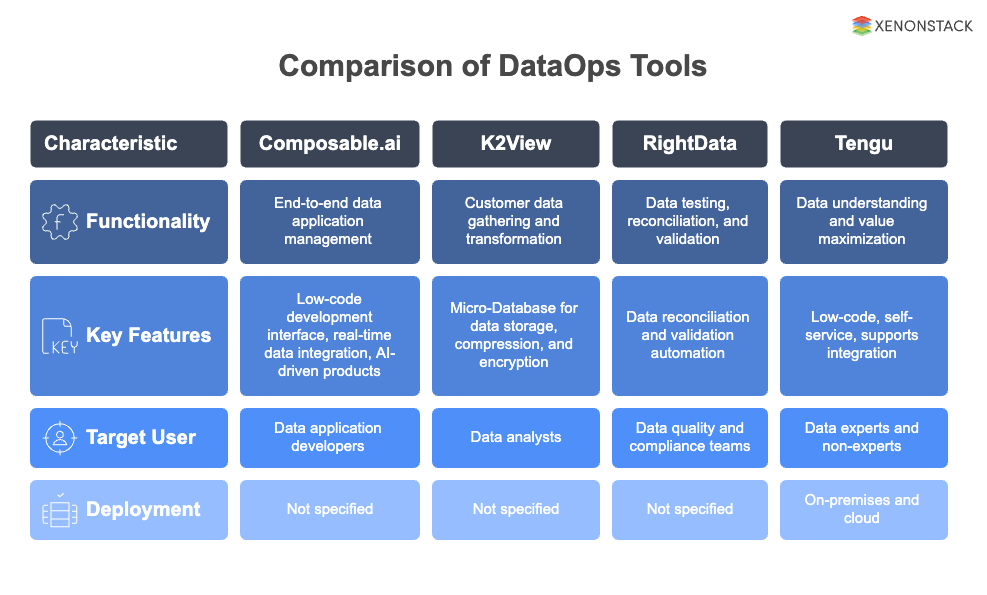
Some of the best DataOps tools are:
1. Composable.ai
DataOps is an Analytics-as-a-Service that offers an end-to-end solution for managing data applications. Its low-code development interface allows users to set up data engineering, integrate data from various sources in real-time, and create data-driven products with AI.
2. K2View
To make the customer data easily accessible for analytics, this DataOps tool gathers it from various systems, transforms it, and stores it in a patented Micro-Database. These Micro-Databases are individually compressed and encrypted to improve performance and data security.
3. RightData
The data testing, reconciliation, and validation services offered by this DataOps tool are practical and scalable. Users can create, implement, and automate data reconciliation and validation processes with little programming knowledge to guarantee data quality, reliability, and consistency and prevent compliance issues. Dextrous and RDt are the two services that RightData uses for its tool.
4. Tengu
A low-code DataOps tool called Tengu is made for data experts and non-experts. The business offers services to assist companies in comprehending and maximising the value of their data. To set up their workflows, Tengu also provides a self-service option for existing data teams. Additionally, users can integrate many tools thanks to its support. Both on-premises and in the cloud are options.
Makes sure data follows both internal and external mandates and data is secure, private, accurate, available, and usable. Taken From Article, Data Governance
How does DataOps help Enterprises build Data-Driven Decisions?
DataOps as a Service combines multi-cloud big-data/data-analytics management and managed services around harnessing and processing the data. Using its components, it provides a scalable, purpose-built big data stack that adheres to best practices in data privacy, security, and governance.
Data Operations as a service means providing real-time data insights. It reduces the cycle time of data science applications and enables better communication and collaboration between teams and team members. Increasing transparency by using data analytics to predict all possible scenarios is necessary. Processes here are built to be reproducible, reuse code whenever possible, and ensure higher data quality. This all leads to the creation of a unified, interoperable data hub.
Enable Deeper Collaborations
As businesses know what data represents, it thus becomes crucial for collaboration between IT and industry. It enables the company to automate the process model operations. Additionally, it adds value to the pipelines by establishing KPIS for the data value chain corresponding to the data pipelines. Thus enabling businesses to develop better strategies for their trained models. It brings the organisation together in different dimensions. It helps bring localised and centralised development together. A large amount of data analytics development occurs in various corners of enterprises that are close to business, using self-service tools like Excel.
The local teams engaged in distributed analytics creation play a vital role in bringing creative innovations to users. However, as said earlier, lack of control pushes you down the pitfall of tribal behaviours; thus, centralising this development under it enables standardised metrics, better data quality, and proper monitoring. Rigidity chokes creativity, but with it, it is easy to move into and for motion between centralised and decentralised development; hence, any concept can be scaled more robustly and efficiently.
Set up Enterprise-level DevOps Ability
Most organisations have completed or are iterating over building Agile and DevOps capabilities. Data Analytics teams should join hands and leverage the enterprise’s Agile and DevOps capabilities to follow the latest trends in DataOps.
-
The transition from a project-centric approach to a product-centric approach (i.e., geared toward analytical outcomes).
-
Establish the Orchestration (from idea to operationalisation) pipeline for analytics.
-
Automated process for Test-Driven Development
-
Enable benchmarks and quality controls at every stage of the data value chain.
Automation and Infra Stack
One of its primary services is to scale your infrastructure in an agile and flexible manner to meet ever-changing requirements at scale. Integrating commercial and open-source tools and hosting environments enables the enterprise to automate the process and scale Data and analytics Stack services. For example, it provides infrastructure automation for:
-
BI Stack
-
Data lakes
-
Machine learning infrastructure
Orchestrate Multi-layered Data Architecture
Modern-day AI-led data stacks are complex and have different needs, so it’s essential to align your data strategy with business objectives to support vast data processing and consumption needs. One of the proven design patterns is to set up a multi-layered architecture (raw, enriched, reporting, analytics, sandbox, etc.), with each layer having its value and meaning, serving a different purpose and increasing the value over time. Registering data assets across various stages is essential to support and bring out data values by enabling enterprise data discovery initiatives. Enhance and maintain data quality at different layers to build assurance and trust. Protect and secure the data with security standards so providers and consumers can access data and insights safely. Scale services across various engagements and reusable services.
Building end-to-end Architecture
Workflow orchestration plays a vital role in binding together the data flow from one layer to another. It helps automate and operationalise the flow. Leverage the modularised capabilities to Key pipelines supported by it are:
-
Data Engineering pipelines for batch and real-time data
-
Standard services such as data quality and data catalog pipeline
-
Machine Learning pipelines for both collection and real-time data
-
Monitoring reports and dashboards for both real-time data and batch data
Monitoring and Alerting Frameworks: Provide building monitoring and alerting frameworks to continuously measure how each pipeline reacts to changes and integrate them with infrastructure to make the right decisions and maintain coding standards.
Future Actions to Utilize DataOps Principles
To fully leverage DataOps principles, organizations should focus on integrating predictive analytics, automating workflows, enhancing collaboration across teams, migrating to the cloud, and implementing strong data security policies, ensuring seamless data flow and informed decision-making for future growth.