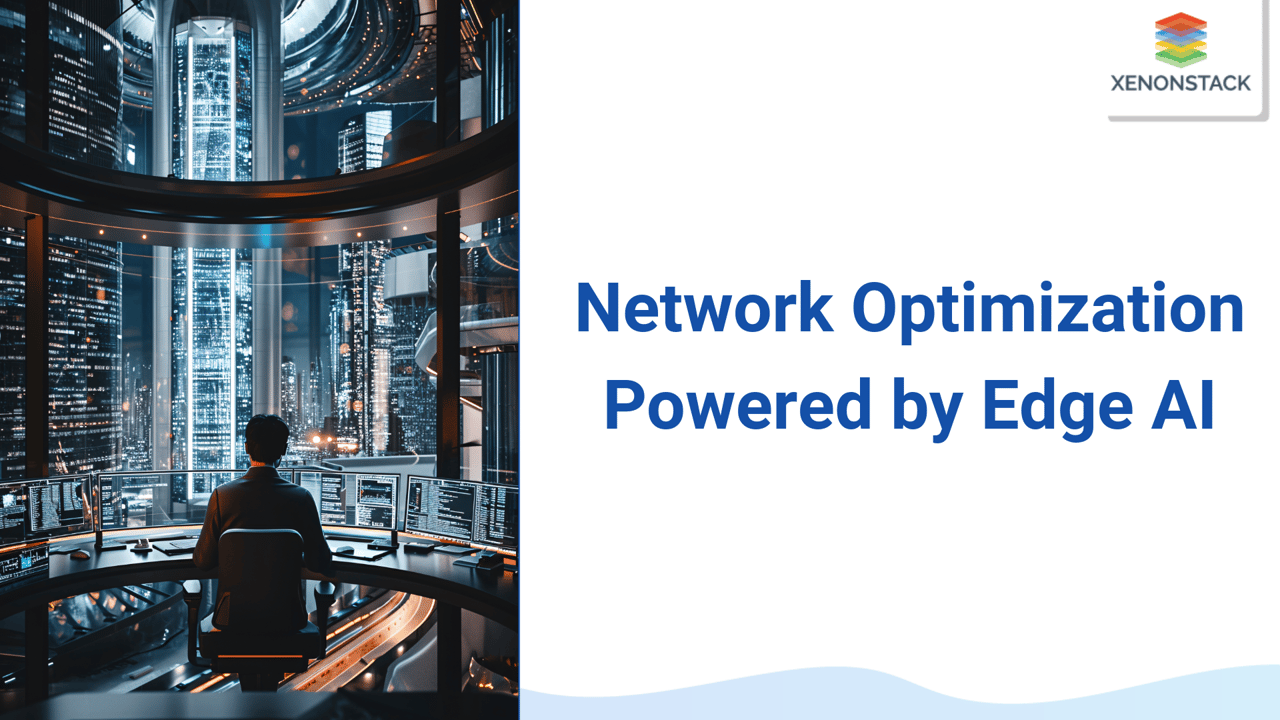
Training and Inference at the Edge AI
AI model development at the edge involves two key processes: training and inference.
Training at the Edge
Leveraging large data sets in training AI models has always been a resource-intensive activity in large server farms. In recent years, however, some training processes have shifted to local computations due to the development of edge computing and model optimisations. These strategies include pruning, quantisation, and low-rank adaptation to optimise the AI model for edge devices.
Inference at the Edge
Inference involves using trained models to make predictions or decisions in real time. Edge devices equipped with AI inference capabilities can:
-
Analyze sensor data for industrial automation.
-
Detect anomalies in network traffic for cybersecurity.
-
Enable real-time diagnostics in healthcare.
Edge inference's low latency and energy efficiency make it invaluable for applications demanding immediate feedback.
The Role of Pluggables in the Network Edge
Pluggable, such as optical transceivers, enable high-performance Edge AI systems. These components ensure seamless, high-speed data transmission between edge devices and central networks.
Contributions to Network Optimization
Enhanced Data Transmission: Facilitate rapid and reliable communication, which is critical for AI-driven insights. Telemetry Integration: Provide real-time monitoring data to central AI systems, enabling dynamic network adjustments. Adaptive Functionality: Support diverse use cases by adapting to varying conditions and requirements.Pluggable bridges the gap between the edge and core network, ensuring optimized performance and scalability.
The Role of Pluggables in the Network Edge
Pluggable, such as optical transceivers, enable high-performance Edge AI systems. These components ensure seamless, high-speed data transmission between edge devices and central networks.
Contributions to Network Optimization
-
Enhanced Data Transmission: Facilitate rapid and reliable communication, critical for AI-driven insights.
-
Telemetry Integration: Provide real-time monitoring data to central AI systems, enabling dynamic network adjustments.
-
Adaptive Functionality: Support diverse use cases by adapting to varying conditions and requirements.
Pluggable bridges the gap between the edge and core network, ensuring optimised performance and scalability.
Advantages of Edge AI in Network Optimisation
Edge AI offers numerous benefits that revolutionise network management:
-
Reduced Latency: Local processing makes Real-time decision-making possible, which is fundamental to use cases such as autonomous vehicles and manufacturing automation.
-
Enhanced Privacy and Security: Retains key data locally on the device, reducing vulnerability to external attacks.
-
Bandwidth Optimisation: Reduces the overall amount of information to be transmitted, thus relieving traffic jams on the Internet and other communication networks and, simultaneously, saving on costs.
-
Offline Capabilities: Aids in operation in such places or when the network is inaccessible.
-
Cost Efficiency: This does not entirely depend on the cloud resources, which are costly, leading to low operational costs.
-
Scalability: Can sustain the IoT device's growth independently and exponentially without affecting the network capabilities.
Applications of Edge AI
Edge AI is transforming industries by enabling innovative applications:
Smart Cities
-
Traffic management systems analyse real-time data to reduce congestion.
-
Energy optimisation systems ensure efficient utility usage.
-
AI-driven waste management improves resource allocation.
Industrial Internet of Things (IIoT)
-
Real-time equipment monitoring enhances operational efficiency.
-
Predictive maintenance minimises downtime and reduces repair costs.
Healthcare
-
Wearables with Edge AI provide real-time health insights, improving patient outcomes.
-
Remote diagnostics enable efficient medical services in underserved areas.
Retail
-
Frictionless checkout systems streamline shopping experiences.
-
AI-driven inventory management ensures stock availability and reduces waste.
Furthermore, Edge AI is a new broadbanding model that helps a network work faster, wiser, and more securely due to computation decentralisation. AI at the edge allows organisations to obtain insights in near real-time while scaling much more efficiently and keeping data more private. Across industrial automation, healthcare, logistics, and transportation, Edge AI holds the opportunity to transform these industries and how networks work.
That is why Edge AI will become the main driving force in developing connections and using artificial intelligence in the future. It is the edge where the path to network optimisation through AI and RAN computing starts.
Learn more about optimizing IT support and operations with Network Optimization using Edge AI.