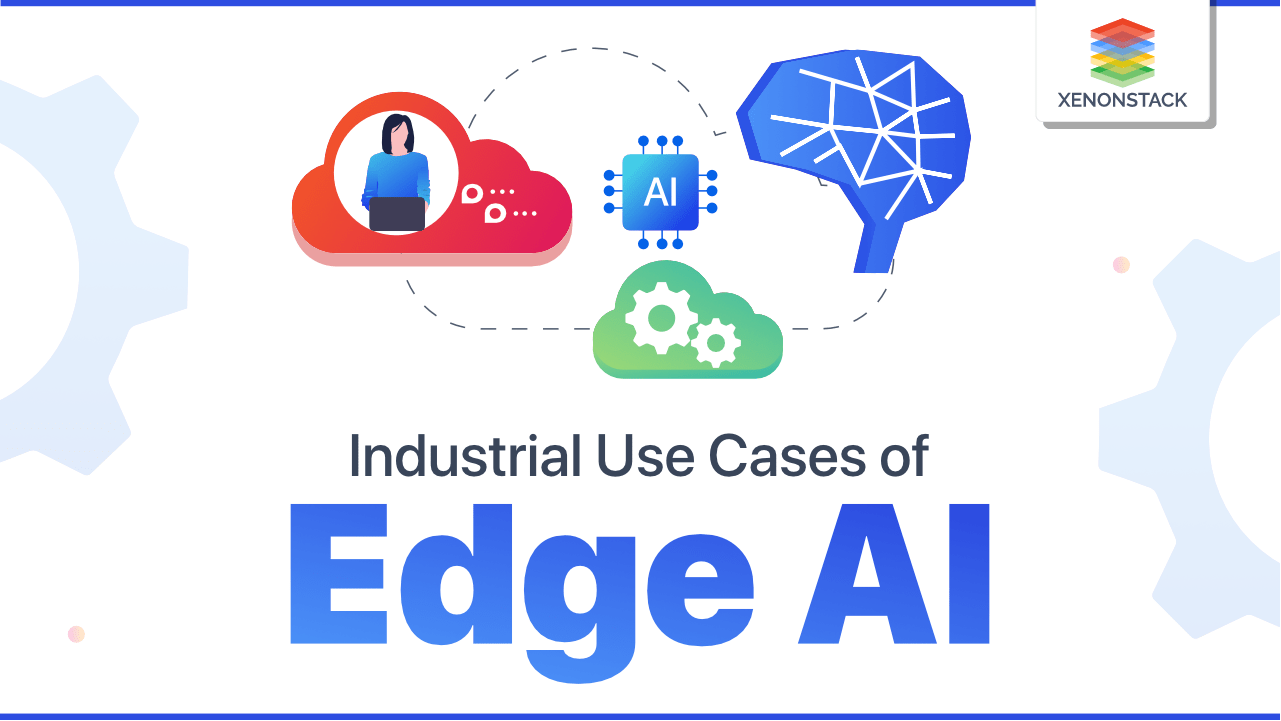
Introduction to Edge AI
Edge AI is a combination of artificial intelligence and edge computing. Locally, algorithms are processed on the machines on the manufacturing line or a nearby server. The algorithms use data collected by various sensors and created by the machines themselves. Machines can make autonomous decisions in milliseconds without connecting to the Internet or the cloud in this way.
With developing technologies such as deep learning and neural networks, which have revolutionary promise but are dependent on cloud computing, their runtimes are hampered, and large power requirements are incurred.
"Latency is becoming a concern as organizations strive to push more data to software that operates in the cloud or in the data center," according to Forrester Research, an international information technology consultancy. As the volume of data grows, it becomes less cost-effective to handle everything centrally.
Edge AI have opened up opportunities to take a fresh and practical approach to data processing and fuel a range of technology-driven solutions. Click to explore our, Edge AI Architecture and its Applications
The pandemic has increased the use of edge computing, which refers to computation and data storage close to the point of use.
- By 2028, the Linux Foundation's State of the Edge report predicts that digital health care, Manufacturing, and retail enterprises will employ edge computing more extensively. This is largely due to the technology's ability to reduce reaction times and bandwidth consumption while allowing for more flexible data analysis.
- In 2025, IoT devices alone will generate more than 175 zettabytes of data.
- Grand View Research estimates that the worldwide computing market will be valued at $61.14 billion by 2028.
How Edge AI Works?
Edge AI involves deploying artificial intelligence (AI) algorithms and models on edge devices, such as smartphones, IoT devices, and other embedded systems, to enable faster and more efficient data processing. Here are the general steps of how Edge AI works:
- Data Acquisition: Edge devices capture data from various sensors or sources, such as cameras, microphones, and environmental sensors.
- Data Pre-processing: The acquired data is pre-processed to clean, filter, and format it to be used by AI algorithms and models.
- Local Inference: AI algorithms and models are deployed on the edge device, enabling real-time data processing and decision-making. The processed data can trigger actions, make decisions, or provide insights.
- Data Transmission: The processed data is sometimes transmitted to remote servers or the cloud for further analysis or storage.
- Model Updates: AI models deployed on edge devices may need to be updated regularly to improve accuracy or to adapt to changing conditions. These updates can be done remotely or through manual intervention.
Overall, Edge AI enables data to be processed and analyzed in real-time on the edge device, reducing the need for data transmission to remote servers or the cloud. This approach enables faster response times, improved data security and privacy, and more efficient use of network resources.
It is necessary to implement data and AI Ethics. AI must be developed and deployed ethically. Taken From Article, Ethical AI Challenges and it's Solutions
Edge AI vs. Cloud AI
When we talk about Edge AI, it is somewhat different from AI. Edge AI is a subset of AI. Apart from Edge AI, AI does have other subsets too. There is also a Cloud AI, which involves a centralized server for data processing, and the ML algorithms are applied there. Cloud AI and Edge AI have advantages and disadvantages, which are applicable based on the requirement and needs.
Edge AI emerges as a better alternative whenever there is a need for real-time prediction and data processing. Since there is latency in the case of Cloud AI, it is not a better model for that. Edge AI and Cloud AI are two approaches to deploying artificial intelligence (AI) algorithms and models, with advantages and disadvantages. Edge AI refers to deploying AI algorithms and models on edge devices, such as smartphones, IoT devices, and other embedded systems. This approach enables faster response times and more efficient use of network resources, as data is processed locally on the device.
Cloud AI, on the other hand, refers to deploying AI algorithms and models on cloud servers. This approach provides more processing power and storage capacity, enabling more complex and sophisticated AI models to be trained and deployed.
Here are some critical differences between Edge AI and Cloud AI:
- Latency: Edge AI offers lower latency as data is processed locally on the device, while Cloud AI sends data to remote servers, resulting in higher latency.
- Data Privacy: Edge AI offers better privacy as data is processed locally on the device. At the same time, Cloud AI involves sending data to remote servers, potentially exposing sensitive data to third-party servers.
- Processing Power: Cloud AI offers more processing power and storage capacity than Edge AI, enabling more complex and sophisticated AI models to be trained and deployed.
- Network Bandwidth: Edge AI requires less bandwidth as data is processed locally on the device, while Cloud AI involves sending data to remote servers, requiring more network bandwidth.
Edge AI in Manufacturing
Manufacturers worldwide have begun to alter their manufacturing processes with AI at the edge. The following use cases of edge computing are helping manufacturers improve their efficiency and productivity.
Predictive Maintenance
Sensor data may be utilized to discover anomalies early and anticipate when a machine will break, referred to as predictive maintenance. Sensors on equipment scan for defects and notify management if a machine needs to be repaired, allowing the problem to be treated quickly and with minimal downtime. The manufacturer can precisely analyze equipment conditions using sensor data, artificial intelligence, and edge computing, avoiding costly unexpected downtime. Sensor-equipped video cameras, for example, are used in chemical facilities to detect corrosion in pipelines and inform workers before any damage is done.
Quality Control
Detecting flaws is an essential aspect of the manufacturing process. Defects must be caught in real-time when running an assembly line that produces millions of units. Edge computing devices can make microsecond choices, detect faults immediately, and warn workers. This feature gives firms a significant edge by reducing waste and increasing manufacturing efficiency.
Equipment Efficiency
Manufacturers are constantly seeking ways to optimize their operations. When combined with sensor data, Edge computing can be used to evaluate overall equipment effectiveness. For example, in the automobile welding process, producers must follow several regulations to ensure that their welding is of the highest quality. Companies can monitor products in real-time using sensor data and edge computing, catching flaws or safety issues before leaving the facility.
Yield Optimization
Knowing the exact quantity and quality of the components used in the manufacturing process is crucial in food processing plants. Machines can rapidly recalibrate if any parameters need to be modified to generate better quality products using sensor data, AI, and edge computing. There is no requirement for manual oversight or data to be sent to a central location for analysis. The sensors on-site are capable of making real-time decisions to boost yields.
Edge AI has the potential to revolutionize Video Analytics and Surveillance System worldwide. Taken From Article, Edge AI in Video Analytics
Edge AI in Healthcare
The use cases of Edge Artificial Intelligence in Healthcare are described below:
Monitoring of Hospital Rooms
In general, the ability to automate processes is one of AI's distinctive selling points. AI algorithms may collect data from various sensors and analyze it to choose the best course of action. This is taken to the next level with Edge AI. It provides autonomous monitoring of hospital rooms and patients using computer vision and data from other sensors.
Fall Detection
Many wearables now include the capacity to detect if a person falls suddenly by utilizing specific technology. These gadgets can be trained to detect falls in real-time and even notify caretakers. In most circumstances, this can save a person's life. The fall detection feature on Apple Watch is an example of this.
Monitoring vital indicators is another area where it can be quite useful. Medical equipment that captures heart rate, temperature, respiration rate, blood pressure, and other parameters can use AI to detect any anomaly in real-time. The devices may then alert hospital staff, who could subsequently take action. This is important for the patient, but it also improves their whole experience.
Radiology
DICOM (Digital Imaging and Communications) images in radiology are quite huge. As a result, sending these photos to the cloud or a central server for processing and receiving machine learning inference can be quite costly and time-consuming. On the other hand, Edge AI allows the analysis to take place locally, resulting in a considerably faster diagnosis.
In the healthcare industry, advanced imaging applications are used. Some of them are:
Abnormalities in the Cardiovascular System
The automation of cardiovascular problems in routine imaging tests such as chest X-rays may result in speedier decision-making and fewer diagnostic errors. Artificial Intelligence applied to the concept of imaging data can also aid in recognizing key issues such as muscle thickening, monitoring changes in total blood flow in the heart, and so on.
Fractures and Other Musculoskeletal Injuries Detection
Using Artificial Intelligence to detect hairline fractures, soft tissue injuries, and dislocations can help surgeons feel more confident about their treatment options. Supporting the Diagnosis of Neurological Disorders, Algorithms can help speed up the diagnosis of neurological diseases by identifying photos with questionable findings and providing risk ratios for whether the images contain signs of PLS or ALS.
Edge AI in Smart Homes
Edge Artificial Intelligence is highly used in making smart homes. Some of the use cases ofEdge Artificial Intelligence in smart homes are:
Security and Privacy
Data is sent to fog nodes established in the home network by smart security devices such as security cameras, video doorbells, and alarms. This information is analyzed locally on the edge node to detect unwanted activity, provide notifications, and raise alarms.
Entertainment
In a smart home concept, entertainment is a significant plus. It's ideal to control music, audio, or video in a smart home setup. The rise of smart televisions has also increased its position in smart home setups.
AI can be used for customized marketing and sales offers, rating based recommendation, location-based recommendations. Click to explore here, Artificial Intelligence in Retail
Edge AI for Video Surveillance System
While several applications for computer vision, few are as time-sensitive as safety and security. When a computer vision system detects a possible risk to your company, you must act quickly and decisively.
It means that speed is of the essence for security video analytics. Unfortunately, many computer vision systems are too sluggish to provide real-time analysis. Therefore, instead of processing the acquired images or video locally, they send it to a cloud-based machine with a higher processing capacity. Large-scale deployment of computer vision for safety and security AI is hampered by latency difficulties (waiting for data to be uploaded and processed).
As a result, Edge Artificial Intelligence is becoming increasingly important in security video analytics. Edge computing is a data processing paradigm in which data is processed on "edge" devices that are physically close to the original capture site rather than on cloud servers.
Edge AI in Retail Industry
Retail stores are facing very tough competition from e-Commerce. Also, Covid 19 situation has shifted most buyers from retail stores to eCommerce.
The customer benefits from a better in-store experience using Edge AI, including a walk of a simplified, easy-to-navigate shop, a "Just Walk Out" automatic checkout with no lines, and tailored offers and functions. It's comparable to online shopping, but with the bonus of being able to feel and touch the merchandise. Customers may also have the option of shopping in-store and ordering delivery or in-store pickup and connecting to online resources for navigation, extra information, customization, digital try-on, and more while in-store.
Just Walk Out Stores
Pick-and-pay or pick-and-go stores are sometimes known as just-walk-out stores. This is usually a small convenience store where consumers can use their phones to enter the store, select the things they want, and, as the name implies, walk out. Cameras are used in these systems, occasionally combined with additional sensors.
Smart shopping Carts
The cart records the inserted (or removed) items and allows for in-cart payment through a mobile app. A smart camera serves as the central sensor and recognizes products placed in the cart. Pressure sensors are added to the bottom of some carts to cross-reference weight data with visual input, interact with the shopping cart, and install a smartphone app or an on-cart touchscreen.
Intelligent Checkouts
A counter or gate near the exits of a business that optically scans the products in a shopper's cart, basket, or conveyor belt. The smart camera detects and recognizes the products, and the purchase is billed using a POS system or a mobile app.
Edge AI is a powerful tool for retailers looking to improve their surveillance capabilities and reduce costs. Taken From Article, Retail Surveillance Using Edge AI
Edge AI for Banking and Financial Services
Edge Artificial Intelligence use cases for finance and banking sector are listed below:
Customer Engagement
In retail banking, traditional customer engagement programs are frequently ineffective. Static adverts for mortgages, loans, and other financial products are presented in a bank's physical locations without any targeting of customers beyond a basic analysis of customer segments utilizing that area. The transition to digital banking has resulted in very limited client segmentation for advertising purposes. Banks want to use cloud flexibility (e.g., quick and flexible adjustments to advertising materials) while keeping the advantages of more local computing (e.g., real-time changes to personalize the advertising). This is where cutting-edge solutions come in handy.
Retail banks can enable highly personalized client engagement down to the individual level by promoting appropriate financial products and services using edge capabilities. With the recent deployment of SoftBank's Robotic Pepper robot in some of its US branches1, HSBC illustrates the types of services edge can provide. Pepper interprets and responds to client information requests using natural language processing, and it can even detect and respond to basic human emotions. This necessitates the processing of massive amounts of data in real-time with low latency, which an edge computing solution is meant to provide. While flagship branches in big cities may have the room and resources to run servers on-site, most branches may choose to shift this requirement to an edge location where it can be done more cost-effectively.
Conclusion
Though Edge AI is still in its early stages of research, it is gaining traction in various industries. It could be used in various industries, including driverless vehicles, smart cities, industrial manufacturing, healthcare, and financial services. It can also be used in artificial intelligence virtual assistants and augmented reality gadgets. Edge and cloud technologies must cooperate. Companies will see the necessity of the edge and cloud operating in harmony to drive intelligent business decisions as they embrace digital transformation, Smart Manufacturing, and all of the advanced use cases that these efforts bring.
- Explore the Top 5 Edge Computing Platforms
- Click to know about Role of Edge AI in Automotive Industry
- Click to explore here Generative AI Solutions