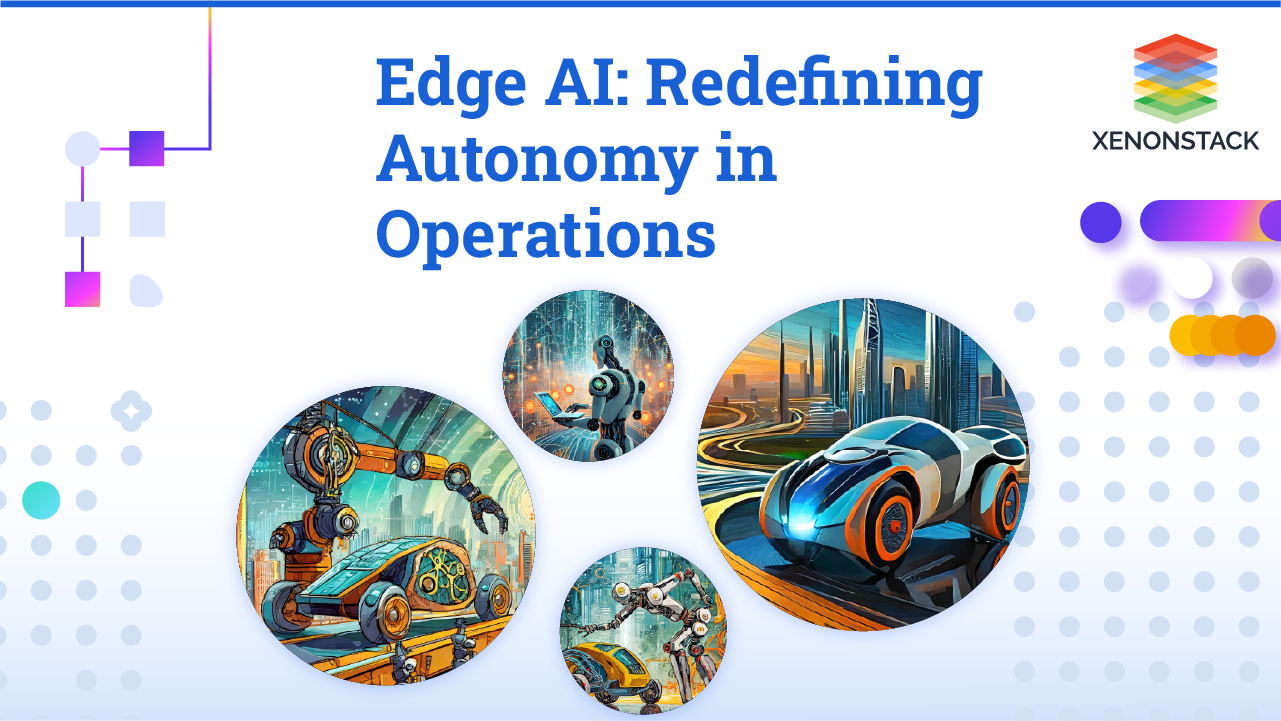
What is Edge AI?
Edge AI is a combination of edge computing and AI. Edge computing is based on the same general idea that data is created, stored, collected, processed and managed at a local location, rather than at a data centre. Edge AI takes this idea to the devices level. Edge AI uses machine learning, or ML, which mimics human thinking, to reach the point where a user interacts with a machine, edge server or IoT device.
Why EDGE AI important for autonomous systems?
EDGE AI provides several advantages for autonomous systems, such as:
-
Reduce latency and response times: EDGE AI processes data on edge devices, eliminating the need for data to be sent to the cloud to be processed. This reduces latency and allows autonomous systems to respond more quickly to environmental changes.
-
Reduce privacy and security risks: EDGE AI stores data locally at the edge device, reducing the chance of data breaches and unauthorised access.
-
Reduce bandwidth requirements: By using EDGE AI, autonomous systems can reduce the amount of data they need to transmit over the network, resulting in lower bandwidth costs and improved network performance.
-
Decreased network outages and fault tolerance: An EDGE AI system is less likely to fail due to network outages or other disruptions, as it can continue to operate in the event of a connection to the cloud being lost.
Components of EDGE AI for Autonomous Operations
Edge Devices
-
Edge devices are physical devices that are used to power the EDGE AI model. Edge devices typically have low processing power and limited memory, but are small, low power, and inexpensive. Common edge devices include embedded systems, microcontrollers, and FPGAs.
-
Edge sensors are devices that collect environmental data. These data can include things like temperatures, pressures, vibrations, images, and more. Edge sensors can be integrated directly into edge devices, or they can be connected to edge devices via wired and wireless interfaces.
AI Algorithms and Models
-
EDGE AI models typically use machine learning (ML) or deep learning (deep learning) algorithms to learn from data and make predictions or decisions.
-
Deep learning algorithms are a subset of machine learning, which uses artificial neural networks (ANNs) to learn complex patterns from data.
-
After an edge device has been trained, an AI model can be deployed to it. The edge device then uses the model to process the data and make real-time decisions.
Edge device connectivity and communication
-
Edge devices are typically connected to the cloud or another network via Wi-Fi or Bluetooth or cellular networks.
Key factors to consider when implementing EDGE AI
Data collection and preparation
-
Data collection and preparation for EDGE AI requires high-quality data. To train effective AI models, you need to collect data that is relevant to the task the AI model will perform.
-
Data must be accurate and free from errors.
-
Data diversity must be representative of a wide range of situations and scenarios.
-
Once you have collected your data, you need to prepare it for training. This may include cleaning your data, preprocessing your data, extracting features from your data, etc.
Model selection and training
-
The selection of an AI algorithm and model is based on the specific job that the autonomous system is going to perform. Once the algorithm and model are chosen, the model can then be trained using the pre-trained data.
What is model training?
-
Model training is the iterative process of fine-tuning the hyperparameters of the model and evaluating the model’s performance. The aim of model training is for the model to be able to generalize to new data and to be able to perform well on the task at hand.
Deploy and Optimize
-
After training an AI model, you can deploy it to your edge device. But before you can deploy your model, you need to optimize it for efficiency and performance. You can do this by using techniques like model compression, model quantization, or pruning.
Applications of EDGE AI in Autonomous Operations
EDGE AI is used in a variety of autonomous operations applications, such as:
-
Preventing equipment failure and condition monitoring
-
Improving safety by preventing unplanned downtime
-
Making decisions and controlling autonomous systems in real-time
-
Robotic, autonomous vehicle and industrial automation applications
-
Detecting and recognizing objects in environment
-
For surveillance, security and quality control
-
Navigating and path planning
-
Drones, mobile robots and autonomous vehicles
Challenges and Future Trends
Data privacy and security
-
EDGE AI systems need to consider data privacy and security. Edge devices collect sensitive data, which must be protected from unauthorized access and exploitation.
Edge computing limitations
-
Edge devices are limited in processing power and memory. This limits the complexity of the AI models you can deploy to them. However, edge computing hardware is improving these limitations.
Advances in AI algorithms and hardware
-
AI algorithms and hardware are constantly being developed. These advances are enabling the development of more efficient and powerful AI models for the EDGE AI applications.
Conclusion
EDGE (Edge-to-Edge) AI is a form of artificial intelligence (AI) that runs on edge devices rather than on the cloud. This type of AI helps autonomous systems make decisions in real-time and respond to changes in the environment.
There are a number of challenges that come with implementing an EDGE AI system. These include:
-
Data privacy and security
-
Edge computing limitations
-
Specialized AI algorithms
-
Hardware
EDGE can be used in a variety of ways in autonomous operations. It can be used for predictive maintenance, decision-making in real-time, object detection and navigation, and more.
- Discover more about Intelligent Enterprise for Retail Industry
- Click to explore about Intelligent Robotic Process Automation