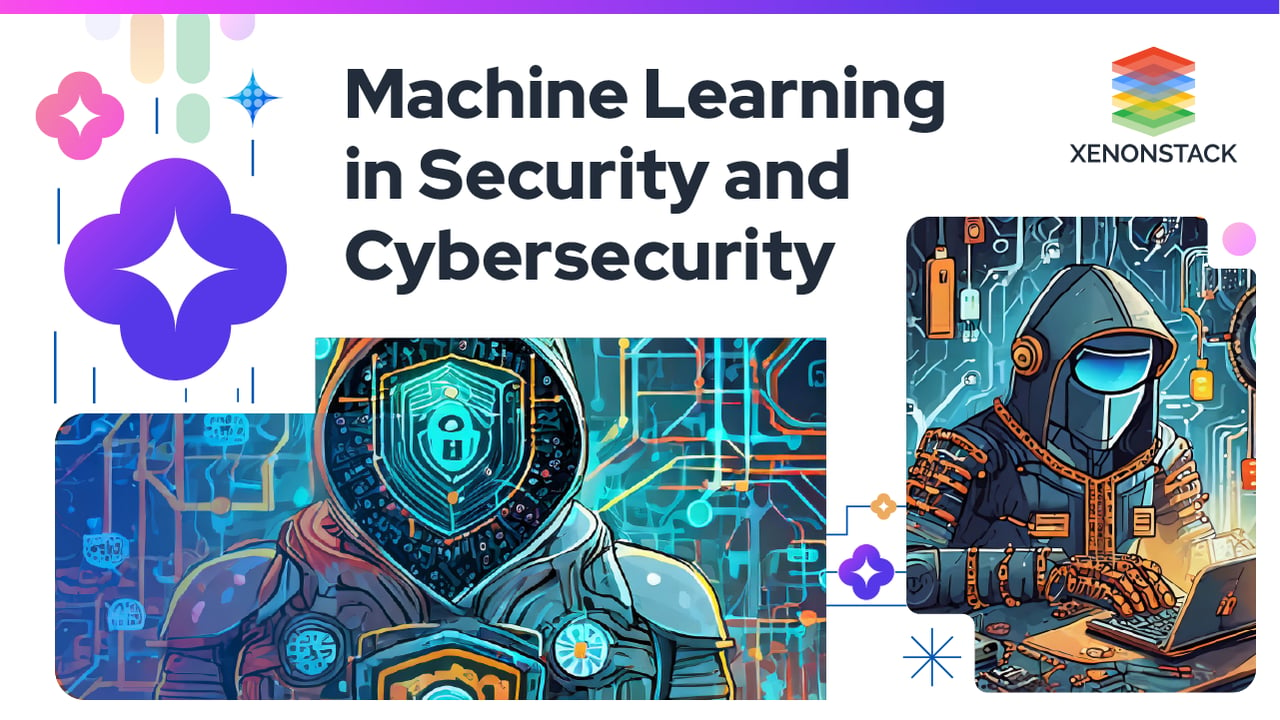
Exploring Machine Learning’s Role in Security
Step into the dynamic digital world, where the internet fuels progress but also presents a playground for cyber threats. In this evolving landscape, the significance of security cannot be emphasized enough. Enter machine learning (ML), a powerful tool within artificial intelligence that stands at the forefront of surrounding defences against these ever-changing cybersecurity challenges.
In the current digital landscape, where internet security is paramount, machine learning and artificial intelligence integration have emerged as formidable defence mechanisms against the ever-changing landscape of cyber threats. ML enables computers to learn autonomously, mimicking human cognition through iterative trial-and-error processes. This transformative capability is particularly impactful in security, where ML algorithms tirelessly analyze vast datasets to unveil data patterns indicative of malicious activities such as malware or insider threats.
Machine Learning for Security
Machine learning enables computers to learn autonomously, mimicking human learning through trial and error. It is a key artificial intelligence component where machines learn to analyze data and identify patterns. In cybersecurity, ML serves as a foundational technology for threat detection, where systems analyze data for anomalies that might indicate malicious behaviour or an insider threat.
ML Technologies for Security
Leveraging AI and ML technologies, security systems can better detect malware concealed within encrypted traffic, identify insider threats, and predict the emergence of "bad neighbourhoods" online.
Online security is crucial for everyone who uses the Internet. Most cyber-attacks are spontaneous and target common vulnerabilities rather than specific websites or organizations. Machine learning (ML) and AI offer a proactive approach to cybersecurity, empowering organizations to fortify their defences against myriad threats. As we navigate the digital landscape, the symbiotic relationship between ML, AI, and cybersecurity promises to pilot a new era of robust digital security, ensuring a safer and more resilient online environment for all.
Why is machine learning essential for Cybersecurity?
Machine learning (ML) is pivotal in enhancing cybersecurity, providing proactive solutions to detect and respond to evolving threats. By leveraging ML, security systems can identify patterns, predict potential risks, and automate responses to breaches, ensuring more effective protection. Here’s how ML contributes to online security:
-
Ensuring Online Security: Everyone connected to the Internet needs security online. Most cyber-attacks target common vulnerabilities rather than specific organizations. By leveraging machine learning (ML), systems can be trained to identify patterns and detect malicious or abnormal activities more effectively than traditional methods.
-
Proactive Threat Detection: Machine learning and AI allow systems to predict cyber threats and detect potential breaches using advanced tools and techniques. ML algorithms can identify anomalies and patterns in data, enabling faster threat detection and response.
-
Automated Responses: With ML, security systems can automatically respond to breaches by recognizing trends and patterns. This reduces manual intervention, enabling quicker detection, reporting, and corrective actions during an attack.
-
Enhancing Behavioral Analytics: Behavioral analytics powered by ML further enhance security by identifying attacks through unusual user behaviour and anomalies, improving the ability to detect insider threats and other suspicious activities.
Discover how autonomous operations powered by machine learning are revolutionizing cybersecurity, enhancing threat detection and response in real-time. Explore the future of self-optimizing defense systems in our latest blog.
Key User and Entity Behavioral Analytics Tools (UEBA)
-
ManageEngine Log360
-
Aruba Introspect
-
Exabeam Advanced Analytics
-
Cynet 360
-
LogRhythm UserXDR
How ML Powers Security and Cyber Defense?
The ever-evolving cyber threat landscape necessitates continuous monitoring and correlation of vast amounts of external and internal data points within an organization's infrastructure and user activities. Managing such a large volume of data is unfeasible with a limited human workforce.
By automating the analysis process, cybersecurity teams can swiftly identify threats and pinpoint instances that require further human investigation. Here’s how machine learning (ML) contributes to enhancing security:
Detection of Network Threats
Machine learning (ML) is crucial in identifying threats by constantly monitoring network behaviour for anomalies. ML engines analyze enormous amounts of data in real-time to detect significant events. These techniques effectively uncover insider threats, previously unknown malware, and violations of security policies.
Enhancing Online Safety
Machine learning algorithms are adept at identifying novel malware attempting to execute on endpoints. They can recognize new malicious files and activities based on known malware characteristics and behaviour patterns.
Advanced Malware Detection
Machine learning algorithms are adept at identifying novel malware attempting to execute on endpoints. They can recognize new malicious files and activities based on known malware characteristics and behaviour patterns.
Securing Cloud Data
Machine learning can scrutinize suspicious login activities in cloud applications, detect location-based anomalies, and conduct IP reputation analysis to identify risks and threats within cloud platforms effectively.
Top ML Frameworks and Tools for Cybersecurity
Machine learning in security involves:
-
Software-defined Networking (SDN): Enhances network flexibility by separating control and data planes, allowing dynamic flow adaptations based on application needs.
-
Network Function Virtualization (NFV): Utilizes virtualization to decouple software from hardware, reducing costs and adding network functionality.
-
Machine Learning Techniques: Employed in network security using algorithms like unsupervised, supervised, and reinforcement learning for precise threat detection and enforcement of security protocols.
Best ML-based Security Tools
-
biotics: A bio-inspired AI framework for securing critical network applications.
-
Cyber Security Tool Kit (CyberSecTK): Python library for preprocessing and feature extraction for cyber-security data.
-
Cognito by Vectra: AI solution for threat detection and response across diverse network environments.
Use Cases of ML in Security and Cybersecurity
Machine learning (ML) transforms cybersecurity by providing practical solutions to various threats. Here are some key applications of ML in cybersecurity and security:
Abnormal/Malicious Activity Detection
-
Problem Statement: Abnormal and malicious activities remain a critical challenge in security and cybersecurity.
-
Solution: ML algorithms can proactively identify and prevent unusual activities, safeguarding systems against potential cyberattacks. Detecting abnormal and malicious activities is at the forefront of ML applications in security. ML algorithms are trained to recognize standard behaviour patterns within a network or system. By continuously analyzing activities, these algorithms can swiftly identify deviations that may indicate a cyberattack or breach, allowing organizations to mitigate potential threats quickly and reduce risk.
SMS Fraud Detection:
-
Problem Statement: With the increasing prevalence of mobile devices, SMS fraud has become a common attack vector for cybercriminals.
-
Solution: ML models are trained to distinguish between legitimate and fraudulent SMS messages by analyzing message content, sender information, and sending patterns. These models can alert users to potential scams, preventing phishing attempts or malicious links. This application of ML protects individual users and helps maintain the integrity of communication networks.
Human Error Prevention
-
Problem Statement: The challenge lies in mitigating human errors in cybersecurity processes, particularly in identifying and preventing security threats within large datasets.
-
Solution: ML platforms help prevent human errors by efficiently filtering out malicious activities from large datasets. These platforms automatically analyze and detect potential security threats, reducing the need for manual analysis and minimizing the risk of human mistakes. This proactive approach enhances the accuracy and effectiveness of threat detection, strengthening overall cybersecurity defences.
Anti-virus and Malware Detection
-
Problem Statement: Traditional anti-virus and malware detection software relies on signature-based methods that often fail to catch new or evolving threats.
-
Solution: ML enhances these tools by employing anomaly detection and behaviour-tracking algorithms. These sophisticated ML models analyze software's characteristics and actions, identifying malicious programs based on their behaviour rather than relying on known signatures. This approach improves the detection rates of zero-day threats, offering a dynamic defence mechanism against malware.
Email Monitoring
-
Problem Statement: Email remains a primary attack vector for cyber threats, with phishing attempts becoming increasingly sophisticated.
-
Solution: ML models, particularly those utilizing Natural Language Processing (NLP) algorithms, scrutinize email content, sender information, and metadata to identify phishing attempts. By learning from datasets of known phishing emails and legitimate communications, ML models can accurately flag suspicious emails, protecting users from fraud and information theft.
Bot Detection
-
Problem Statement: Not all bots are created equally; some perform legitimate functions, while others are designed for malicious purposes. Differentiating between 'beneficial' and 'malicious' bots is essential to upholding online services' security and operational integrity.
-
Solution: ML algorithms analyze behavioural patterns, such as the frequency of requests, nature of interactions, and speed of actions, to identify and block malicious bots. This ensures that services remain available to legitimate users and prevents spam, data scraping, and DDoS attacks.
Network Threat Detection
-
Problem Statement: As networks become more complex, identifying threats amidst the vast amount of legitimate traffic is increasingly challenging.
-
Solution: Machine learning models are highly proficient in scrutinizing network data traffic to pinpoint malicious patterns indicative of cyber threats. Through ongoing data flow monitoring, these models can detect irregularities like abnormal data transfers or sudden spikes in traffic directed towards specific destinations. This capability empowers IT teams to investigate and address potential threats swiftly.
Benefits of Machine Learning in Security
The various benefits of Machine Learning based security are:
- Continuous Improvement: AI/ML technology evolves by learning from business network behaviours and identifying web patterns, making it challenging for hackers to breach the network.
- Scalability: AI/ML can efficiently handle large volumes of data, enabling Next-Generation Firewall (NGFW) systems to scan numerous files daily without causing disruptions to network users.
- Enhanced Detection and Response: Implementing AI/ML software in firewalls and anti-malware solutions accelerates threat identification and response times, reducing the reliance on human intervention and improving overall effectiveness.
- Comprehensive Security: AI/ML solutions provide security at both macro and micro levels, creating barriers against malware infiltration and enabling IT professionals to focus on addressing complex threats, enhancing overall security posture.
Best Practices for Leveraging ML in Cybersecurity
-
Secure Data: Safeguard the data utilized in your machine learning models by implementing appropriate access controls and encryption measures to mitigate the risk of unauthorized access or data breaches. For instance, filters can be integrated into web servers to block requests from suspicious sources and spam.
-
Auditing: Consistently audit and assess your ML systems to verify their proper functionality and absence of vulnerabilities.
-
Monitoring: Continuously monitor the status of your ML systems to detect any potential issues or anomalies in real-time.
-
Testing: Conduct unit and integration testing to ensure proper software functionality and detect potential issues early.
-
Patching: Keep your ML system updated with patches to fix vulnerabilities and stay informed about security issues in open-source projects to prevent exploitation, including threats like phishing attacks.
-
User Authentication and Encryption: Authenticate users who access your ML models and encrypt authorized user sessions to protect against unauthorized access or data interception.
-
Protect Against Attacks: Implement measures to defend against malicious code, data theft, and insider threats that could compromise the security of your ML system.
-
Choose Reliable Companies and Technologies: Select reputable ML service providers and ensure they have robust security measures. Keep yourself informed about the most recent security technologies and optimal procedures.
-
Transparent Auditing: Use ML services that offer transparent auditing processes to track service improvements and address emerging threats.
-
Regular Review and Updates: To stay protected against potential vulnerabilities, regularly review and update firewall rules, software versions, and security patches.
Explore the essentials of multi-cloud security in our comprehensive guide. Learn how to protect your data across multiple cloud environments with advanced strategies and machine learning to safeguard against evolving cyber threats.
Challenges in Adopting Machine Learning in Security and Cybersecurity
While machine learning (ML) holds immense potential to revolutionize cybersecurity and security, deploying and ensuring its effectiveness is not without challenges. Integrating ML into security protocols comes with obstacles, ranging from data-related issues to the inherent complexities of ML systems. Here are some key challenges professionals face in harnessing the full power of ML for cybersecurity:
-
Insufficient Training Data: ML algorithms require significant diverse training data to perform effectively. Inadequate data can lead to biased predictions and hinder model accuracy.
-
Data Quality Concerns: The quality of training data dramatically impacts the performance of ML models. Errors, outliers, or noise in the data can compromise model accuracy, emphasizing the importance of high-quality training data.
-
Complexity of Machine Learning: Machine learning's dynamic nature presents practitioners with a complex and evolving process. Tasks such as data analysis, preprocessing, and complex computations contribute to the challenges faced in ML implementation.
-
AI/ML Vulnerabilities: Security risks associated with AI and ML technologies extend to various applications. Adversarial attacks targeting machine learning systems pose a significant threat. Unintentional information leakage and data breaches due to team member negligence are common vulnerabilities.
-
Adversarial Attacks on ML Systems: ML systems are vulnerable to adversarial attacks that manipulate model behaviour, mainly targeting visual input systems and analytics. Hackers exploit vulnerabilities in machine learning algorithms, posing a growing security threat.
-
Data Poisoning and Model Integrity: Relying on publicly available datasets for training can introduce the risk of tainted data. Deliberate data poisoning during training can compromise model integrity, allowing malicious actors to exploit vulnerabilities in AI systems.
Master multi-cloud management with practical strategies and best practices to optimize security, performance, and cost-efficiency across cloud environments.
Future of Machine Learning in Security and Cybersecurity
As technology advances rapidly, particularly AI and ML, its impact will be shaped by how it is controlled and utilized. The future of machine learning in security and cybersecurity promises to transform how we safeguard digital assets, yet it comes with challenges in its ethical use and technological evolution. Strengthening Apache ZooKeeper Security Using Kerberos.
Scope in Security with ML
Machine Learning holds immense potential in security systems. ML algorithms are increasingly playing a key role in enhancing threat detection as threats evolve. By analyzing vast datasets, these algorithms identify patterns and anomalies that may indicate potential security breaches. In the future, ML will continue to advance in:
-
Adaptive Threat Detection: Continuously evolving to counter increasingly sophisticated cyber threats.
-
Automated Incident Response: Streamlining security operations by automating responses to security events.
-
Enhanced Authentication: Improving user authentication protocols to prevent unauthorized access.
-
Security Orchestration: Integrating ML into various security layers creates a more cohesive, intelligent security system that automatically adjusts to emerging threats.
These advancements will make security systems more adaptable, intelligent, and efficient in protecting sensitive data.
Scope in Cybersecurity with ML
The cybersecurity industry faces a significant shortage of skilled professionals, but ML and AI are expected to alleviate some of this burden. However, the rise of advanced ML technologies also brings challenges, as hackers can use these tools to execute more complex attacks. Future trends in ML for cybersecurity include:
-
Botnets and AI-powered Attacks: Hackers will increasingly employ botnets powered by sophisticated algorithms to exploit vulnerabilities. This trend calls for deep learning systems like Deep Neural Networks (DNNs) that can distinguish between benign and malicious activity to strengthen cyber defence.
-
Weaponized AI: Adversaries may also attempt to weaponize AI against cybersecurity measures. Anticipating and counteracting these threats will be crucial to future cybersecurity strategies.
In the evolving field of cybersecurity, ML and AI must be equipped to predict, detect, and neutralize emerging threats as they become more advanced and pervasive.
ML’s Transformative Role in Cybersecurity: Key Insights
Integrating machine learning (ML) into security and cybersecurity practices offers significant advancements in data analysis, threat detection, and predictive analytics. ML algorithms' ability to identify malicious behaviour, anomalies, and insider threats allows organizations to stay ahead of potential cyberattacks.
However, challenges such as adversarial attacks and ensuring data privacy must be addressed. With continued development in artificial intelligence and automated analysis, ML will strengthen defences against evolving cyber threats, enabling organizations to better safeguard their digital assets and sensitive information.
Moving Forward: Harnessing ML for Security Excellence
Consult with our experts about implementing advanced machine learning systems in security and cybersecurity. Learn how various industries and departments leverage AI-driven workflows and decision intelligence to become more threat-centric and data-driven. Harness machine learning to automate and optimize cyber threat detection, enhancing efficiency and responsiveness in protecting IT infrastructures and sensitive data.