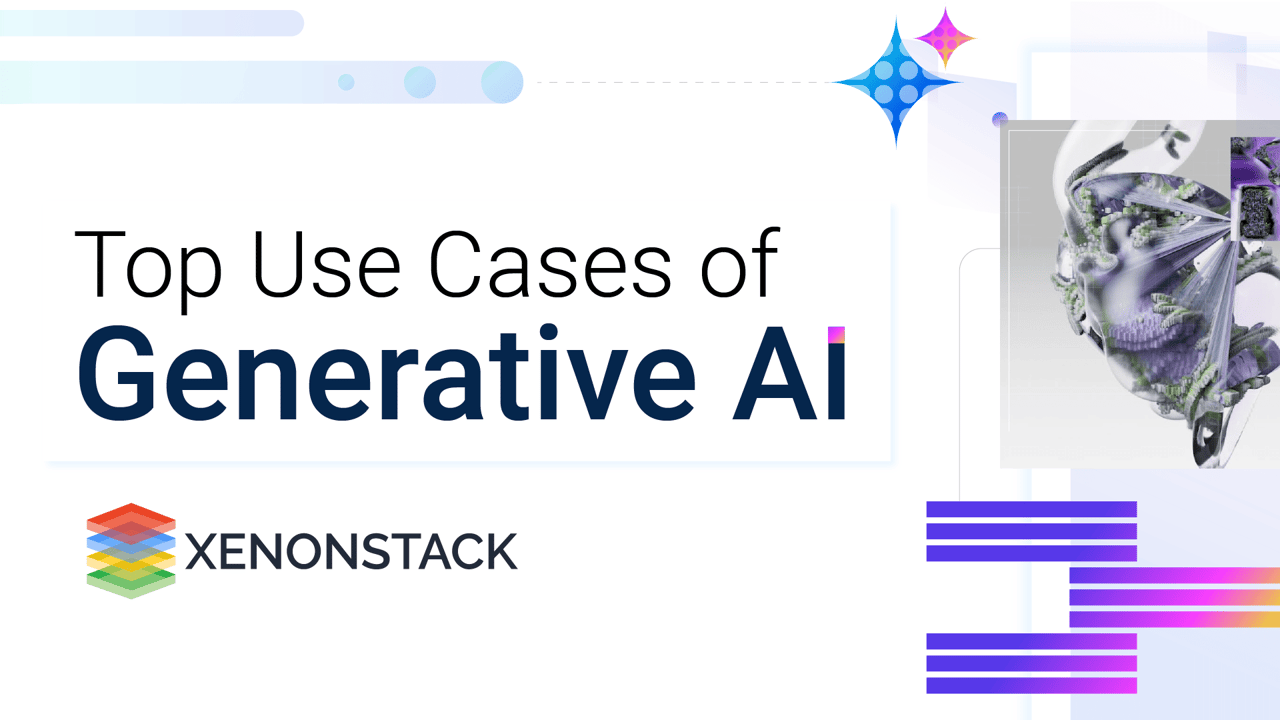
What is Generative AI?
Generative AI is a type of artificial intelligence that can generate new content and ideas, including conversations, stories, images, videos, and more. It is supported by foundation models that are pre-trained on large amounts of data. Organizations can reconfigure their applications, create new customer experiences, achieve unprecedented productivity levels, and transform their businesses through generative AI.
Generative AI tools use sophisticated algorithms to evaluate data and derive new and unique insights, thereby improving decision-making and streamlining operations. These applications can help businesses stay competitive by creating customized products and solutions in a continuously developing market.
Global Generative AI Market Trends
The generative AI market is experiencing rapid growth, driven by technological advancements and increasing demand across various sectors. Generative AI applications are transforming industries by enhancing user experiences, streamlining workflows, and providing valuable insights from complex datasets.
-
Projected Market Growth: The generative AI market is projected to grow at 47.5% CAGR, increasing from $43.87 billion in 2023 to $667.96 billion by 2030.
-
Enterprise Adoption Rate: By 2026, over 80% of enterprises are expected to adopt generative AI APIs or models, up from under 5% in 2024.
Transformative Trends in the Generative AI Market
The generative artificial intelligence market has seen remarkable growth and transformation in recent years. One major trend has been an increased focus on improving user experiences through generative AI-based tools and applications. These applications have been efficient in gaming, entertainment, and design.
The increasing demand for generative artificial intelligence applications is witnessed in various sectors, primarily driven by technological advancements like super-resolution, text-to-image generation, and text-to-video conversion. Additionally, there is a pressing need to streamline workflow processes within organizations, contributing to the surge in demand for such applications.
Advanced generative models, including Deep Convolutional GANs (DCGANs) and StyleGANs, have significantly impacted the market, generating high-quality and realistic images and videos. Generative AI is also increasingly used for automated content creation and curation, benefiting domains such as social media, marketing, and journalism, where AI-generated content can streamline processes and improve content relevance and engagement.
How Does Generative AI Work?
Generative AI Architecture uses neural network models to identify patterns and structures in existing data to create new and original content. One of the breakthroughs with generative AI models is their ability to leverage different learning methods, including unsupervised or semi-supervised learning, for training. This has allowed organizations to leverage large amounts of anonymous data to create baseline models more easily and quickly.
Unlike analytical and traditional AI-based conversational interfaces constrained by pre-defined commands, conversational AI comprehends, learns, and crafts chat responses based on context and intent. Generative virtual assistants, leveraging models like NLP, deep learning, and NLG, empower conversational interfaces to engage users in human-like interactions. Hence, conversational AI overcomes the challenge of providing limited responses by enhancing its understanding of user inquiries.
Key Drivers of Generative AI Value Chain
A new value chain is emerging to support the training and utilization of generative AI systems as they continue to develop and be deployed:
1. Computer Hardware
Massive quantities of data are essential for generative AI systems to generate content, a task that conventional computer hardware cannot handle. Extensive clusters of GPUs or TPUs equipped with specialized accelerator chips are required to process the vast amount of data across billions of parameters simultaneously. The chip design market is largely dominated by NVIDIA and Google, while TSMC is responsible for producing nearly all accelerator chips.
2. Cloud Platforms
GPUs and TPUs are costly and scarce, making it impractical for most businesses to possess and maintain this vital hardware infrastructure on-premises. As a result, most of the work to create, fine-tune, and operate large AI models occurs in the cloud. This allows companies to access computational power and manage their expenses as needed quickly.
3. Foundation Models
Foundation models are the backbone of generative AI. They are large deep-learning models pre-trained for creating specific types of content and can be modified for various tasks. These models have undergone training using vast datasets, including publicly unlabeled data obtained from different sources and privately obtained data from significant databases.
4. Model Hubs and MLOps
Businesses seeking to develop applications using foundation models need a platform to store and retrieve the model and specialized MLOps tools and technologies to customize and implement the model in their end-user applications. Model hubs serve as a gateway for closed-source models, offering various services for open-source models.
5. Applications
Generative AI may increase productivity and economic efficiency across many different industries. The most promising domains for generative AI applications are information technology, marketing and sales, customer support, and product development. IT teams can gain from automated documentation and code. Customer service can utilize virtual assistants and personalized chatbots to answer natural language requests and customer inquiries.
6. Services
Companies will use dedicated generative AI services to fill capability gaps and navigate business opportunities and technical complexities. Existing AI service providers will expand their capabilities to serve the generative AI market. Specialized niche players will enter the market with knowledge of applying generative AI to specific functions, industries, or capabilities.
Advantages of Generative AI Solutions
Generative AI offers several benefits to businesses and industries:
Increase Efficiency
Generative AI can automate tasks and reduce human errors. This can help businesses save time and money and increase efficiency. For example, generalized AI can quickly and accurately create images and videos, which may be used in marketing campaigns or other projects.
Improve Quality
Generative AI can help improve the quality of generated content. It may produce high-quality, attractive photographs and films that are preferable to those made manually. In addition, it can be used to generate text that is more accurate and relevant than text created by humans.
Improve Decision Making
Generative AI can help companies make better decisions. By using it, businesses can generate data that can enhance data-driven decisions. For instance, Generative AI can produce data that can improve decisions about marketing campaigns or product development.
Increase Creativity
Generative AI can help businesses be more innovative. By using Generative AI, businesses can generate unique concepts that can be used to create new products or recommendations. In addition, synthetic AI can be used to create images and videos that are more engaging than those created manually.
Improve Customer Experience
Transform Your Enterprise with Agentic AI – Discover how Xenonstack AI can drive innovation and efficiency
Generative AI Landscape Categories
Generative AI can be divided into various categories based on the type of content it generates:
1. Text: Summarizing or Automating Content
AI-generated content may quickly produce multimedia content using already-existing data. Marketers use it for newsletters, emails, and branding. AI text generators use large datasets to extract and select the best output. Use cases include:
-
Content writing for personalized emails/posts, interview questions/job descriptions
-
Chatbots to boost website conversion rates
-
Improving natural web search through analysis and synthesis
-
Utilizing content writing to improve sales
-
Summarizing legal documents for risk and legal purposes
2. Code: Generating Code
Many generative AI applications are available for multilingual code generation through text inputs. They can be used as coding assistants and generate code based on context and syntax. Examples include Alphacode, Amazon Codewhisperer, CodeGeeX, and GitHub Copilot. Use cases include:
-
Code generation to accelerate app development with automatic code recommendations
-
Quickly generating user interface designs with application prototype and design tools
-
Generating synthetic data sets to improve AI model quality
3. Images: Generating Images
Brands are using generative AI to create images for commercial use, saving time and money. It allows for initial concept creation and design that human professionals can perfect. Use cases include:
-
Stock image generators to create unique media for marketing and sales
-
Image editors to help personalize content quickly for marketing and sales purposes
4. Audio: Summarizing, Generating, or Converting Text into Audio
Integrating large language models and text-to-image generation improved AI-powered audio generation quality. Speech synthesis models have advanced to the point where they can generate voices that are virtually indistinguishable from human voices. Use cases include:
-
Generating voiceovers for training and educational purposes
-
Designing unique sounds for entertainment while avoiding copyright infringement
-
Modifying and refining podcast recordings in post-production without re-recording
5. Video: Generating or Editing Videos
Generative Video Models have advanced significantly and have many practical applications, such as editing, creation, and video production. They can optimize the design process and help create photorealistic videos with digital humans. Use cases include:
-
Video creation: Using AI avatars to create engaging and interactive video lessons
-
Video editing: Maximizing engagement on social media by editing videos to be shorter and more attention-grabbing
-
Video translation: Dubbing videos, providing live translations, creating voice clones
-
Face swaps and adjustments: Visual effects, lip synchronization, real-time gaze correction during video conferencing
6. Chatbots: Automating Customer Service and More
Large language models, such as ChatGPT, have transformed AI with their capabilities in natural language processing. They can perform tasks such as summarization, writing assistance, code generation, language translation, and sentiment analysis. Customer service applications powered by LLMs have gained significant attention and can be used for demand forecasting, inventory optimization, and risk management in business operations.
7. ML Platforms: Applications/ML Platforms
LLMOps is a refined version of MLOps that focuses on managing large transformer models and monitoring them at scale. LLMOps has been added to Microsoft's Azure Machine Learning platform, providing enhanced capabilities for managing large transformer models.
8. Search: AI-Powered Insights
Organizations use AI-powered knowledge management systems to gather and distribute relevant information for insights. Use cases include:
-
Aiding HR departments by generating job descriptions, identifying required skills, and classifying applicants
-
Improving customer service by providing personalized support, searching for answers in internal documents, and identifying customer problems and sentiments
-
Summarizing business objectives and knowledge to help developers focus on coding
9. Gaming: Gen-AI Gaming Studios or Applications
Generative AI technologies have the potential to greatly enhance the gaming industry by helping to create 3D models, storytelling, and characters. Various applications such as CSM, Illiad AI, and Latitude are already utilizing Generative AI for videogame creation. Pixelvibe and Armorlab also aid in creating game assets and textures through AI-powered software.
10. Data: Designing, Collecting, or Summarizing Data
Generative AI can help bridge the knowledge gap by converting data patterns into plain language, providing enriched context through historical comparisons, and boosting time efficiency by automating the generation of basic insights and summaries.
11. Customer Emails Tools
AI-generated content may quickly produce multimedia content. Marketers use it for newsletters, customer email tools, and branding. AI text generators use large datasets to extract and select the best output.
12. Accessible Content Generation Tools
The emergence of accessible content generation tools is bound to revolutionize how content is created. It is expected to transform text and images, hardware designs, music, videos, and more. Consequently, people must focus on content editing instead of content creation, which demands different skills.
Applications of Generative AI
Generative AI applications can ensure the creation of new realistic animated materials, text, and images in minutes. It offers various generative AI applications that benefit multiple industries, including productivity implications technology such as surveillance, healthcare, marketing, advertising, education, gaming, media, podcasting, and more.
-
Augment Data - Generative AI applications can improve data quality by artificially enriching datasets with additional information similar to the original dataset but not previously seen. This helps to improve the performance of deep learning algorithms, which usually require large amounts of high-quality data to function effectively.
- Synthetic Data - Generative AI is a method of creating synthetic data. This class of data is generated rather than from direct observations in the real world. This ensures the confidentiality of the data source used to train the model. For example, health data may be artificially generated for research and analysis purposes without revealing the patient's identity, whose medical records are used to protect privacy.
- Drug Design - A 2010 study found that the average cost of discovering a new drug and bringing it to market is about $1.8 billion, of which about one-third is the cost of drug discovery. The discovery process can take a whopping three to six years. Generative AI is already being used to develop drugs for various applications in months, offering the pharmaceutical industry a huge opportunity to reduce the cost and timelines of drug discovery.
- Design Neural Network - Generative AI applications can help determine which connections work best by examining different configurations to find the best connection. This is like giving Artificial Intelligence a set of puzzle pieces and asking it to figure out how to put them together to get the best image.
- Chip Design - Generative AI uses reinforcement learning (machine learning techniques) to optimize component placement for mold design (floor planning), reducing product development lifecycle time from weeks by human experts to hours with the AI.
- Create Algorithm - Automate the invention of new ML algorithms because who has the time to do everything by hand? This saves time and resources as AI can scan possible algorithm combinations and identify promising ones for further development increase.
- Design of Parts - Generative AI enables industries such as manufacturing, automotive, aerospace, and defense to design optimized parts to meet specific goals and constraints such as performance, materials, and manufacturing methods. For example, automakers can use generative design to create lighter designs. This can contribute to the goal of improving vehicle fuel efficiency.
- 3D Shape Creation - Research is currently underway in this area to create realistic 3D representations of objects. Better shapes can be created using GAN-based shape generation regarding source similarity. In addition, precise shapes can be manufactured and adjusted to achieve the required shape.
- Increase Image Resolution - While creating new documents from existing content, generative AI employs various techniques. One such technique is called a Generic Adversarial Network (GAN). The generator and the discriminator form a GAN, which generates new data and ensures that it is factual. High-resolution image renderings can be generated with GAN-based techniques using super-resolution GANs.
- Creation of an Instance Image - Generative AI is best known for its ability to create real photos of people. When the input data is an image of someone's face, the model is trained on it and then generates an image with the same face.
- Image-to-Image Conversion - It involves changing the external components of an image while maintaining its internal components, such as color, media, or shape. Such a transformation may involve changing the daytime image into the night-time image. Essential characteristics of an image can also be changed, such as its color or style, using this transformation.
- Text-To-Speech Generator - Text-to-speech Generation has several commercial uses, including marketing, education, podcasting, and advertising. To make class notes more engaging, teachers can turn them into audio files. This technique can also provide educational material to the blind or visually impaired.
- Create Music - Generative AI can help you create original songs for commercials and other creative projects. Please note that using copyrighted material in your workout data may constitute copyright infringement. But there's nothing you can't fix with a bit of legal advice.
- Generate Videos - Generative AI can create videos ranging from short clips to feature films. This can be done through image generation to create visual content, text generation to create scripts or storyboards, and music generation to create soundtracks.
- Generate Image - Another popular Generative AI application converts text to images to create realistic images based on specific settings, themes, styles, or locations. This allows you to quickly and easily create the image you want.
Enterprise Generative AI Use Cases
Generative AI Use cases can transform multiple industry applications:
Logistics and Transportation
Generative AI can accurately convert satellite images into map views, allowing previously unknown places to be discovered. This can be especially helpful for logistics and transportation companies looking to navigate new areas.
Explore further about ArtificiaI Intelligence in Logistics
Travel Industry
Generative AI can help with facial recognition and verification systems at airports. By creating a comprehensive image of a passenger from photos taken from different angles, this technology can make it easier to identify and verify a traveler's identity.
Delve deeper into the application of Generative AI for the Hospitality Industry
Retail
Generative AI can improve the customer experience and deliver personalized messages to consumers via email or online with relevant products to the shopper. It can also analyze customer messages or other communications for signs of fraudulent activity, such as phishing attempts.
Further, investigate the application of Generative AI within the Retail Industry
Supply Chain
Generative AI can help businesses predict demand for specific products and recommendations to optimize their supply chain operations. This can help businesses reduce inventory costs, improve order fulfillment times, and reduce waste and overstocking.
Get insights into the utilization of the Generative AI in the Supply Chain Industry
Energy Sector
Predict solar and wind output based on weather data and production history, thereby helping to optimize grid integration and handle the variability of these resources. With generalized AI, they can optimize the distribution and transmission of electricity, considering factors such as load balancing, congestion management, and asset utilization.
Explore the implementation of Generative AI within the Energy Sector
Marketing
Generative AI can help segment customers, predicting target group responses to advertising and marketing campaigns. This can help businesses target specific audiences and increase their sales.
Revolutionize Marketing with Generative AI
Impact of Generative AI on Different Functions Across Industries
Implementing Generative AI Applications and Use Cases is expected to impact different industry functions. The impact will vary depending on several variables, including the degree of automation and the kind of work that other functions are performing.
1. Sales and Marketing
The rise of gen AI can impact marketing and sales in three areas: Customer experience, growth, and productivity. Gen AI can create hyper-personalized content for Customer experience, jumpstart growth by providing sales teams with analytics and customer insights and automate mundane sales activities to free up time for customer interactions.
Learn how Generative AI is revolutionizing marketing strategies
2. Customer Operations
Generative AI can transform customer service by improving productivity and providing personalized support. Here are five ways it can make a difference:
-
Conversational Search - Customers can quickly get relevant information in their preferred language.
-
Agent Assistance - Automate responses and summaries, empowering agents to provide better support.
-
Build Assistance - AI can generate content and suggestions for customer service tools.
-
Call Centre optimization - AI analyzes the data and provides insights to improve performance.
-
Personalized Recommendations - AI considers customer history to provide tailored information in their preferred format.
Discover the transformative impact of Generative AI on Customer operations
3. Product and R&D
Generative AI has a broad range of applications in product research and development. It can be utilized to design optimized parts to meet specific goals and constraints. Moreover, it is also helpful in creating new products faster, including new drugs, less toxic household cleaners, novel flavors and fragrances, new alloys, and better diagnoses.
Explore the way Generative AI is transforming software development
4. Software Engineering
Generative AI can enhance software development by facilitating low-code solutions, streamlining cybersecurity with integrated AI, accelerating digital transformations and remote work, generating code sections, identifying errors, suggesting code modifications, and optimizing performance.
Explore How Generative AI Supports DevOps and SRE Workflows
5. Supply Chain and Operations
Generative AI is useful in supply chain management for demand forecasting, supply chain optimization, and automating clerical work. It can analyze historical sales data, optimize various supply chain stages, predict operational results, and factor tariffs into operational costs.
Leverage Generative AI Solutions for the Retail and Supply Chain Industry
6. Risk and Legal
Generative AI has several potential legal use cases, including compliance and regulatory monitoring, contract analysis and negotiation, document drafting and review, due diligence, intellectual property management, legal research, and legal chatbots.
Exploring Generative AI's Role in Legal Firms and Beyond
7. Strategy and Finance
Generative AI has numerous applications in finance. It can produce educational and financial scenarios, manage portfolios, simulate risks, identify fraud, analyse financial data, produce customised financial reports, and give enterprise operations contextual knowledge.
8. Corporate IT
Generative AI is widely used in businesses to enhance human creativity and speed up innovation. It can drive operational efficiency, create compelling marketing campaigns, detect fraud, generate realistic virtual agents, automate customer support and sales development, and create marketing content.
Transforming ITOps Observability with the Power of Generative AI
9. Talent and Organization
Generative AI is a powerful tool for transforming talent management. It allows for accurate evaluation and prediction of team member performance, personalized training programs, data-driven job requirements, and assistance for employees and hiring managers.
Revolutionary Generative AI Solutions for HR and Recruitment
10. Designing (Fashion)
Algorithms are used in generative AI to produce distinctive and varied visuals that combine human creativity with computer-generated styling. This approach to creating visual art allows for infinite variations of the same image with no limitations on cost or resources.
11. Complete Stack Applications (Legal, Biotech, Finance)
Generative AI technologies are proving to be of immense help in biotech, particularly in molecule modelling, drug discovery, and protein modelling. The advancement of these technologies is happening at a fast pace, and they hold the potential to enhance the accessibility of biotech solutions greatly.
12. Consumer Avatar and Face Apps
Generative AI is widely used in the entertainment, advertising, and graphic design industries to speed up creative processes and create personalized experiences for audiences. It enables streaming services to generate personalized movie titles and visuals and create unique artwork for TV series based on a user's viewing history and preferences.
13. Multilingual Dubbing
Generative AI is transforming the creation and translation of multilingual multimedia content. AI-driven technologies have now automated the workflows for captioning and subtitling, reducing time and guaranteeing accuracy and consistency across languages.
Key Generative AI Use Cases Transforming Various Industries
Generative AI is revolutionizing various industries with its versatile applications and innovative development approaches. Notable use case models include:
-
Generative AI Architecture for designing complex systems.
-
Generative AI Solutions with Private LLMs that provide customized language models for secure environments.
-
Generative AI in Contact Centers enhances customer interaction patterns by analyzing consumer behaviors.
-
Generative AI Search improves information retrieval.
Sectors like Banking and Healthcare leverage AI for advanced data analysis and personalized recommendations. In Digital Marketing, Generative AI creates impactful campaigns, while Smart Cities benefit from AI-driven urban planning and efficiency. The Retail sector sees improvements in inventory management and customer experience through Generative AI.
As industries adopt Intelligent Automation with Generative AI, the emphasis on the Responsible Use of Generative AI is critical to ensure ethical practices and positive impacts. Overall, Generative AI for Industries is setting new benchmarks for technological innovation and advancement.
Approach to Building Generative AI Applications
The emergence of generative AI is bound to revolutionize how content is created. It is expected to transform text and images, hardware designs, music, videos, and more. Consequently, people must focus on content editing instead of content creation, which demands different skills. Moreover, the way users interact with applications will change with the advancement of AI models. They will become more conversational, proactive, and interactive. This will necessitate a redesigned user experience that revolves around suggestions and recommendations. Although this may boost productivity, it will also challenge the conventional notion of human-led strategy development.
Next Steps with Generative AI
Talk to our experts about implementing Generative AI systems and how industries and different departments leverage Decision Intelligence to become decision-centric. Utilize Generative AI to automate and optimize IT support and operations, enhancing efficiency and responsiveness.