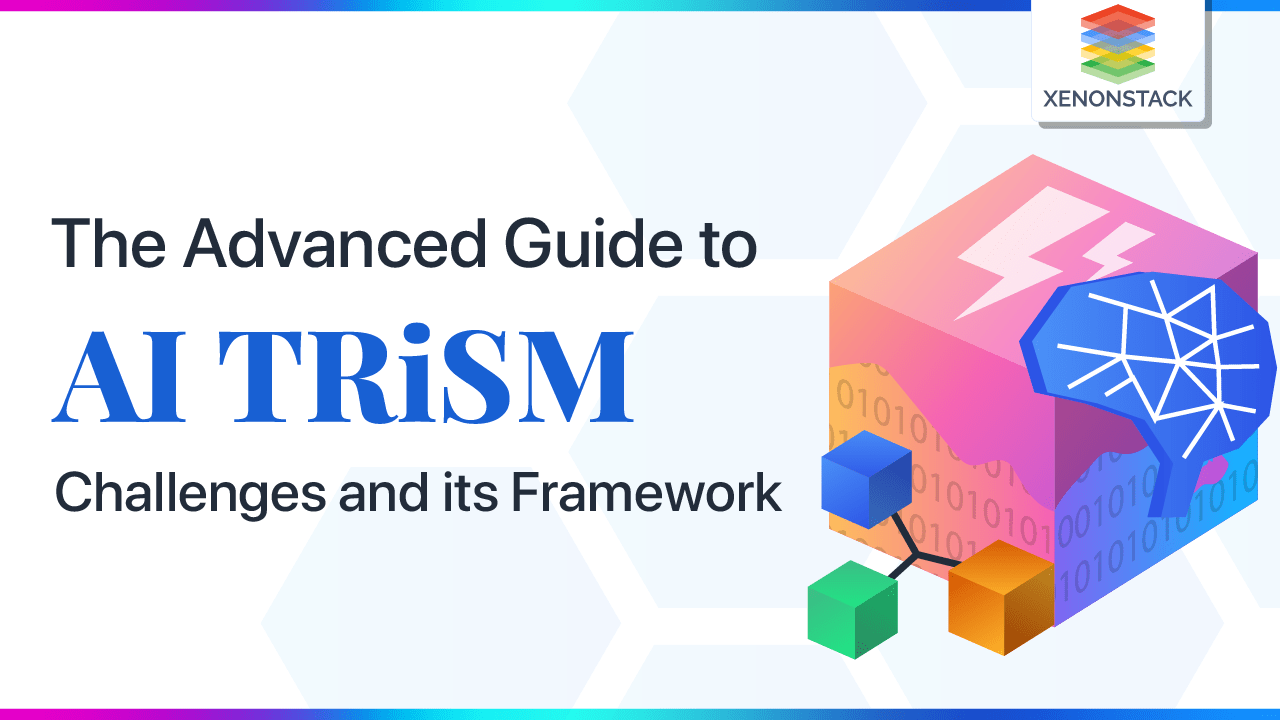
Introduction to AI TRiSM
As our world becomes increasingly dependent on new technologies, it is clear that AI is at the forefront of innovation, driving new applications and approaches to propel us into the future.
Integrating AI into appliances and services has revolutionized our lives, offering a level of accessibility and convenience that was previously unimaginable. The applications of AI are vast, from smart home devices to mobile phones, toys, and machinery. Not only does AI provide numerous benefits that keep businesses competitive and enhance processes, but it also significantly reduces operational costs. Within this realm of technological advancement, a concept emerges AI TRiSM. This concept emphasizes the importance of reliability, trustworthiness, and security in AI systems. This article will delve into the details of AI TRiSM and its significance in our rapidly evolving world.
Artificial Intelligence mimics human action; therefore, it is lightning the burden of humanity. Click to explore about our, Ethics of Artificial Intelligence
What is AI TRiSM?
AI TRiSM, also known as Artificial Intelligence Trust, Risk, and Security Management, is a revolutionary discipline and framework that serves as a backbone for AI Model governance, reliability, fairness, efficacy, privacy, data protection, and trustworthiness.
According to Gartner, AI TRiSM is projected to be a cutting-edge technology in the years ahead. It is estimated that by 2026, organizations that embrace AI transparency, trust, and security will experience a remarkable 50% increase in efficiency in terms of AI Model adoption, business objectives, and user acceptance.
Furthermore, Gartner predicts that by 2028, AI will handle a significant 20% of the workload, with AI and Automation approaches accounting for 40% of the economy. The three essential frameworks of AI TRiSM include:
1. AI Trust
2. AI Risk
3. AI Security Management
6 reasons you need to build AI TRiSM into AI models
1. Proactive Risk Mitigation
AI TRiSM enables organizations to proactively identify and mitigate the risks associated with AI models and applications, ensuring that AI systems are compliant, fair, and reliable and protecting data privacy.
2. Trustworthiness and Reliability
By incorporating AI TRiSM, organizations can ensure that AI systems are trustworthy, reliable, and transparent, fostering user trust and confidence in the AI models and applications.
3. Compliance and Governance
AI TRiSM facilitates the integration of much-needed governance upfront, ensuring that AI systems comply with regulations, standards, and ethical considerations, thereby reducing the potential for legal and ethical issues
4. Security and Data Protection
The framework helps in establishing security protocols and measures to safeguard AI models against cyber threats, ensuring the security and protection of AI data, and respecting individuals' privacy rights
5. Efficiency and Adoption
Organizations that incorporate AI TRiSM into their business operations can see a 50% improvement in adoption rates due to the model’s accuracy, leading to increased efficiency and better customer experiences
6. Future Preparedness
As AI and automation approaches are expected to handle a significant workload and contribute to the economy, AI TRiSM prepares organizations for the future by ensuring the reliability, security, and trustworthiness of AI models and applications
What are the frameworks included in AI TRiSM?
It works to enable Trust, Risk, and Security Management and hold capabilities to anticipate better business outcomes for AI Projects. The main frameworks that are followed for better reliability, security, and trustworthiness are:
1. AI Trust
This framework is associated with transparency or explainability, i.e., the ability to identify if the model achieved the desired outcomes with steps. This helps build trust and transparency.
2. AI Risk
Applying precise and strict governance in managing the Enterprise AI risks. Record and Manage the development and process stages of the models and check all parts of the release process to check the integrity and compliance.
3. AI Security Management
Ensuring Security at each stage of the process in the ML Model operations. AI Security Management is capable of getting access to the entire ML pipeline, identifying anomalies, automating the CI/CD Pipeline, and scanning vulnerabilities.
It is protecting the AI models and their functionality and helping generate better business outcomes with technological advancements and better adoption strategies.
Edge computing is a better option where low latency and decentralization of data are required. Click to explore about our, AI in Edge Computing for Automation
What are the major pillars of AI TRiSM?
There are 5 Basic Pillars of it, which hold the foundation of the AI Trust, Risk, and Security Management concept
1. Explainability
Explainability is the concept of marking every possible step to identify and monitor the states and processes of the ML Models. Simply put, the capability to detect or identify if the model has reached the target.
With this, organizations can monitor the performance of their AI models and propose improvements to make the process more efficient and generate better results with improved productivity.
2. ModelOps
ModelOps focuses on maintaining and managing the end-to-end lifecycle of every AI Model, including models based on analytics, knowledge graphs, decisions, etc.
3. Data Anomaly Detection
As the name implies, this pillar focuses on detecting and identifying issues and helps AI practitioners see the full image of the Data issues to make effective decisions.
4. Adversarial Attack Resistance
Adversarial Attacks are AI attacks or threats that use data to disrupt Machine learning algorithms and alter the machine learning models' functionality. It detects and remediates these threats to ensure a streamlined process throughout.
5. Data Protection
The primary fuel source for machine learning models is data, so the better the data is secured, the better the operations and functionality there can be.
AI TRiSM ensures that there is preferred privacy and security of the data to stay in compliance with the regulations for data protection, such as GDPR
Implementing AI TRiSM Methodology
As it provides high transparency, security, and reliability to operations, most organizations are ready to adopt this approach. They want to gain a competitive edge over other companies or industries. There are three basic steps to adopting AI TRiSM methodology:-
1. Formalizing Documentation and Procedures
With technological advancements, AI is being used everywhere, and the complexity of operations is also increasing. So, proper documentation of the process or the operations provides transparency and enables the monitoring and auditing of events when something goes wrong.
The main reason can be the vast amount of data for the models to operate, as errors are noted when handling exceptional amounts of data. A documentation system can mitigate these errors. It shares its capabilities with industry leaders and data practitioners to formulate an approach and provide an overarching solution to support the technologies.
2. System Checks and Bias Balancing
Checking the systems can enable an organization to prevent breakdowns and improper functionality of the ML models. Checking biases and correcting them to let the model make informed decisions and optimize the processes is preferred.
Checking the situations and alerting to mitigate the issues is something to be focused on to implement AI TRiSM.
3. AI Transparency
The most challenging part of AI is that it still needs more consumer trust. The decision-making capabilities of AI are likely to be questioned because they happen all in the back. Providing transparency and the process structure can help consumers build trust in AI and let it be implemented to improve customer experience and make them comfortable using AI for their daily tasks.
What are the major Challenges in AI adoption?
Although it helps optimize processes and modernize the approaches for most industries and direct customers, AI still faces some challenges. Let’s discuss some key concerns of AI adoption:
- Disruption due to bias
- Lack of human participation
- Insufficient understanding
- Unexpected behavior
Final thoughts on AI TRiSM
AI is poised to revolutionize and automate countless industries in the coming years. Businesses that hesitate to embrace AI may be left behind in the next five years. AI offers a platform for growth, driving business value while ensuring utmost efficiency and accuracy. Furthermore, AI TRiSM enhances business capabilities by optimizing IT systems, leading to improved reliability and data-driven decision-making processes.
- Discover here about AI in IT Infrastructure Management
- Explore here the Future of Generative AI for Enterprises