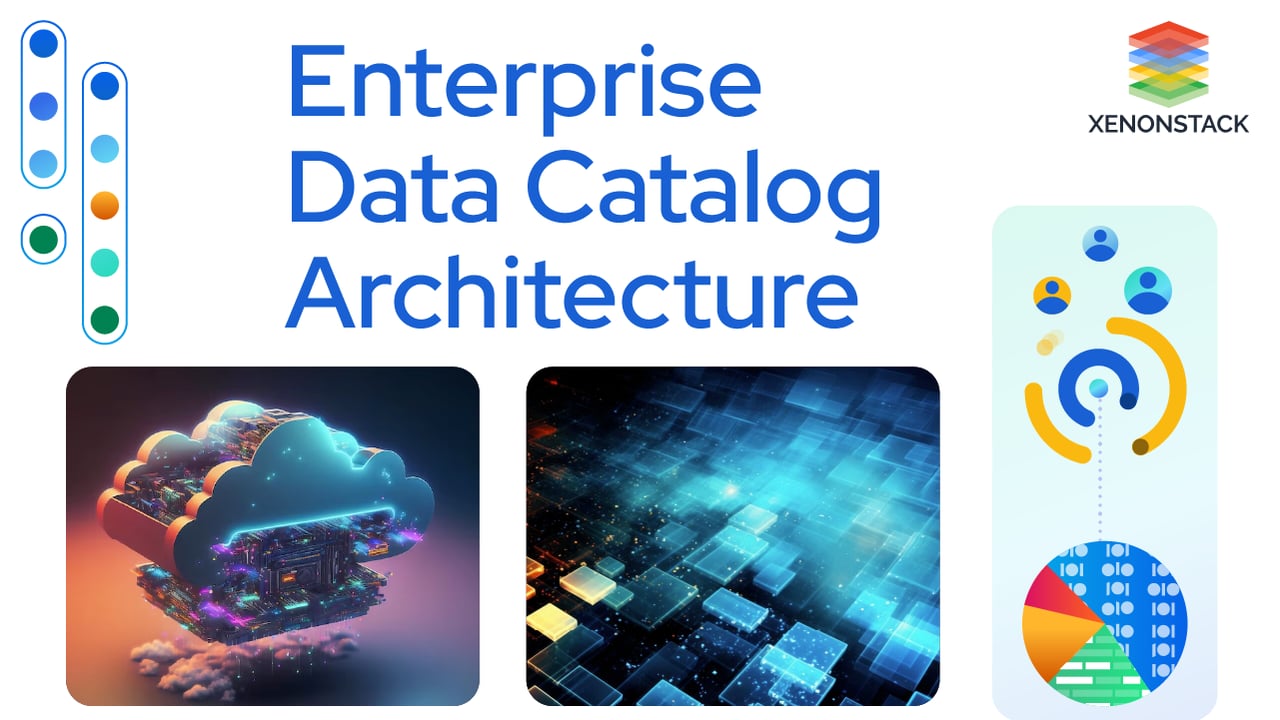
The Data Catalog provides a single self-service environment, helping users find, understand, and trust the data source. It also helps users discover new data sources, if there are any. Discovering and understanding data sources are the initial steps for registering the sources. Users search for the Tools and filter the appropriate results based on their needs.
In enterprises, Data Lake is needed for Business Intelligence, Data Scientists, and ETL Developers where the right data is needed. The users use catalog discovery to find the data that fits their needs. The key capabilities of this approach are as follows:
-
Search and discovery
-
Data Intelligence
-
Manage business glossary
-
Empowering Confidence in Data
Discover further insights into the Top 5 Use Cases of Data Catalog in Enterprises.
What is Metadata?
Metadata is a thread that connects all other building materials, including ways for ingestion to be aware of sources, refinement to be connected to ingestion, and so on. Every component of the architecture contributes to the development and use of metadata.
Explore Azure Data Catalog - Enabling Greater Value of Enterprise Data Assets
Data catalog Architecture
Architecture encompasses the components that gather, manage, and organize data alongside its related information, facilitating user discovery, understanding, interpretation, and utilization of data. The essential elements of its architecture include:
-
Data Assets: These are the datasets available for users to discover and access for analysis and decision-making.
-
Data Metadata: Metadata provides essential information about data assets, such as their origin, lineage, format, and usage, helping users comprehend the context and quality of the data.
-
Data Catalog: The data catalog serves as a central repository, allowing users to search, discover, and understand the data assets they have available.
-
Data Governance: Frameworks for data governance ensure the data catalog's accuracy, reliability, and security, addressing concerns like data quality, privacy, and compliance.
-
Data Lineage: Tracking data lineage enables users to trace the history and provenance of datasets, offering insights into their origins and transformations.
-
Data Usage: Information regarding how datasets are utilized, including the queries, algorithms, and models that depend on them.
-
Data Sharing and Collaboration: It can be shared across the organization, promoting collaboration and enhancing discovery among users.
-
AI/ML Capabilities: It may incorporate AI and machine learning functionalities to automatically discover and extract metadata, reducing the manual effort required for data capture.
-
Integration with Data Sources and Downstream Uses: It should facilitate integration with various data sources and support downstream applications, accommodating both known and potential future requirements.
-
Governance Framework: A robust governance framework is essential to ensure security, privacy, and compliance, especially in a conglomerate of users.
By understanding the key components of a data catalog architecture, organizations can design and implement a data catalog that meets their specific data management and discovery needs.
Deep dive into the Top Enterprise Data Catalog Tools
How to Build an Effective Data Catalog?
Not all solutions are created equal. It's critical to filter options based on key capabilities when selecting a tool. Many platforms, including Talend, rely on essential components that ensure the effectiveness of your data strategy. Let’s examine some of the key features:
1. Connectors and easy-to-curation tools to build your single place of trust
Having many connectors enhances the data catalog's ability to map physical datasets in your dataset, regardless of their origin or source. Using powerful capabilities, you can extract metadata from business intelligence software, data integration tools, SQL queries, enterprise apps like Salesforce or SAP, or data modeling tools, allowing you to onboard people to verify and certify your datasets for extended use.
2. Automation to gain speed and agility
Thanks to improved automation, data stewards won't waste time manually linking data sources. They'll then concentrate on what matters most: fixing and curating data quality problems for the company's good. Of course, you'll need the support of stewards to complement automation – to enrich and curate datasets over time.
3. Powerful search to quickly explore large datasets.
The quest should be multifaceted as the primary component of a catalog, allowing you to assign various criteria to perform an advanced search. Search parameters include names, height, time, owner, and format.
4. To conduct root cause analysis, use Lineage.
Lineage allows you to link a dashboard to the data it displays. Understanding the relationship between various forms and data sources relies heavily on lineage and relationship exploration. So, if your dashboard shows erroneous data, a steward may use the lineage to determine where the issue is.
6. Glossary to add business context to your data
The ability to federate people around the data is essential for governance. To do so, they must have a shared understanding of words, definitions, and how to relate them to the data. As a result, the glossary is helpful. Look for PII in a data catalog. You'll find the following data sources: It's especially useful in the context of GDPR (General Data Protection Regulation), where you need to take stock of all the data you have.
7. Profiling to avoid polluting your data lake
When linking multiple data sources, data profiling is essential for determining your data quality in completeness, accuracy, timeliness, and consistency. It will save time and enable you to spot inaccuracies quickly, allowing you to warn stewards before polluting the data lake.
How Data Catalog Works?
Building a Catalog starts with collecting the metadata of the sources. After obtaining the metadata, the metadata entities need to categorize and assign different tags. ML (Machine Learning) and NLP (Natural Language Processing) are used to automate these processes. Metadata entities auto-assign a tag according to the data entity's name with the Machine Learning model's help. Ultimately, the data steward reviews things and adds value to the Data Catalog.
What are the benefits of a Data Catalog?
The below highlighted are the benefits of Data Catalog:
1. Spend more time using the data not found
As per Forrester Forbes report, data scientists spent more than 75% of their time understanding and finding the data. And more than 75% of them don't like that part of their job—this is due to the questions which they have before working on the queries. The main reason for this problem in an organization is the poor mechanism of handling and tracking all the data. A good Catalog helps the Data Scientist or Business Analyst understand the data and answer their questions.
2. To implement Access Control
When an organization grows, role-based policies are needed; we don't want everybody to modify the data. Access Control should be implemented while building the Data Lake. Particular roles are assigned to the users, and according to those roles, Data Access should be controlled. In the Hadoop ecosystem, implement using Apache Ranger. For the sensitive data in the Data Lake, use encryption for the Data Protection.
3. To Reduce Cost by Eliminating Data Redundancies
A suitable Catalog Tool helped us find the data redundancies and eliminate them. This can help us to save storage costs and data management costs.
4. To follow Laws
There are different protection laws per the data, such as GDPR, BASEL, GDSN, HIPAA, and many more. These laws must be followed while dealing with any data. But these laws stand for different use cases and don't imply every data set. To understand that, we need to know about the data set. A good Catalog helps us ensure that Data Compliance is followed by giving a view of Data Lineage and using Access Control.
AWS Data Catalog is a prospering metadata management service nowadays. Click to explore
Why does Data Catalog Matter?
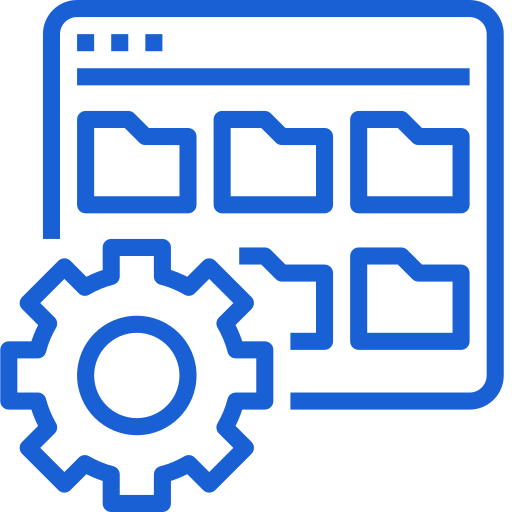
Enhances Data Understanding
A robust catalog aids users in comprehending data by providing easy access to relevant information, including data usage and origin
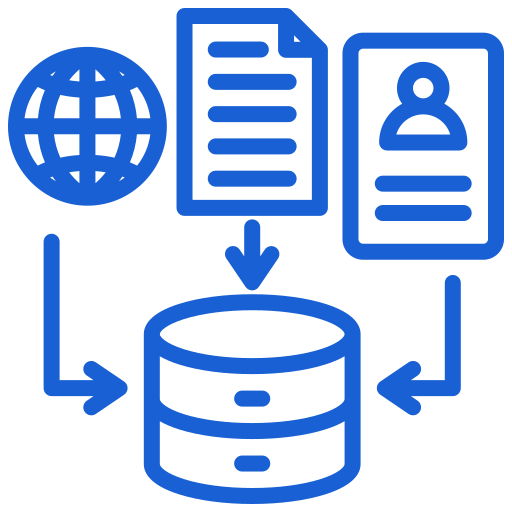
Supports Multiple Data Sources
The catalog aggregates various data sources, helping users identify quality data and gain insights across multiple datasets
How to Adopt a Data Catalog?
Building a Catalog is a multi-step process that includes -
1. Metadata Extraction
It is the very first step of building the catalog. In this step, the metadata of a defined source is collected and stored in the metadata store. It helps in understanding the defined data asset.
2. Data Sampling
It is used to understand the schema, tables, and databases.
3. Auto-Titling (ML and NLP)
Every organization has a naming convention of using abbreviations to define the schema. Natural language processes model to assign that abbreviation a common name understood by the users who are using Catalog.
4. Query Log Ingestion
It collects additional information about the data sets and gives a complete picture of each data set, just like Data Lineage Data Usability.
5. Crowd Sourcing & Expert Sourcing
Up to this layer, the Catalog is ready; it needs to add more values to the Data Catalog. The NLP model has corrected the names of the data assets collected from the data sources, but the Computer-Human Collaboration is also necessary to verify things.
Data Catalog Best Practices
The best practices for Data catalog are listed below:
1. Assigning Ownership for the data set
Ownership of each data set must be defined. There must be a person to whom the user contacts in case of an issue. A good catalog must also tell about the owner of any particular data set.
2. Machine-Human Collaboration
After building a Catalog, the users must verify the data sets to make them more accurate.
3. Searchability
The Catalog should support searchability. It enables data asset discovery; data consumers can easily find assets that meet their needs.
4. Data Protection
Define Access policies to prevent unauthorized data access.
Top Data Catalog software tools
These are some top Data Catalog tools :
-
Alation Data Catalog
-
Alex Augmented Data Catalog
-
Ataccama Data Catalog
-
Atlan Data Discovery & Catalog
-
AWS Glue Data Catalog
-
Collibra Data Catalog
-
Erwin Data Catalog
Explore more in detail about the Data Catalog Tools
Next Step Towards Leveraging Data Catalog
The next step towards leveraging a data catalog involves integrating advanced analytics and machine learning tools, enabling organizations to discover, access, and utilize data more effectively. This empowers teams to make data-driven decisions, fostering innovation and enhancing overall operational efficiency.