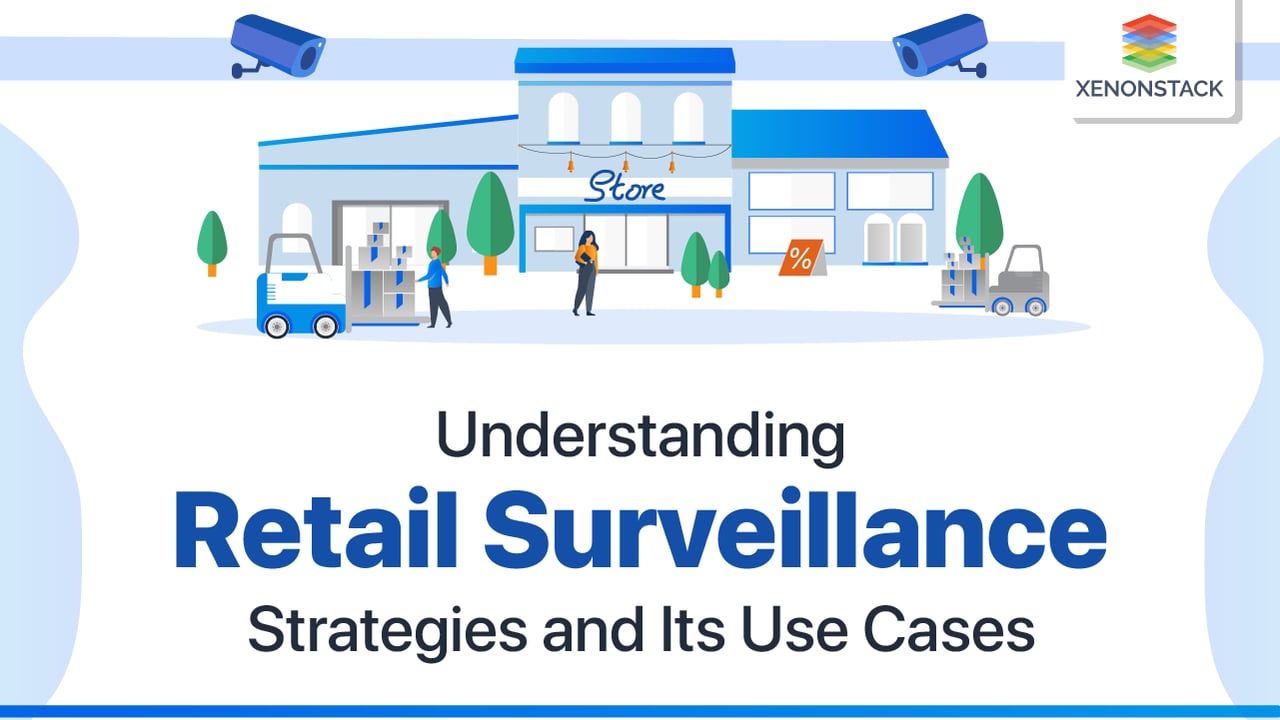
Introduction to Edge AI in Retail Surveillance
Retail surveillance is the use of technology and systems to monitor and evaluate activity inside a retail environment, such as a store or shopping mall. The goal is to increase consumer safety and security, reduce theft and other losses, and improve the entire shopping experience.
Its systems may include cameras, sensors, and other monitoring equipment that record video, audio, and other data regarding consumer behaviour and business operations. This data may then be examined using modern software and algorithms to uncover trends, detect abnormalities, and create insights that can assist businesses in improving their operations and customer service.
Why is Edge AI important in retail surveillance?
Edge AI has recently become one of the most promising technologies. It is a powerful tool for retailers looking to improve their surveillance capabilities, reduce costs, and enhance customers' overall shopping experience.
Edge AI is becoming increasingly important in retail surveillance for several reasons:
-
Real-time insights: Edge AI enables retailers to evaluate data quickly, giving them rapid insights into customer behaviour and shop management. This allows merchants to make educated decisions fast, boosting overall store efficiency and optimising customer shopping experience.
-
Cost savings: Conventional video surveillance systems need significant bandwidth to send data from cameras to a central server for processing. On the other hand, Edge AI solutions process data locally on the camera or device, eliminating the need for costly network infrastructure and saving money on bandwidth.
-
Enhanced security: Edge AI may detect possible security threats such as shoplifting and inform security staff in real time. This assists shops in preventing theft and other forms of loss, which improves their bottom line.
-
Personalisation: Companies may give individualised recommendations and boost customer happiness by analysing consumer behaviour and preferences.
-
Scalability: Since edge AI systems may be readily expanded to support many cameras and devices, merchants can grow their monitoring capabilities as needed.
What is Edge AI?
Edge AI is a technique that allows artificial intelligence (AI) computations to be conducted on a device at the network's edge, such as a camera or sensor, rather than in the cloud or on a central server. This implies that data is handled locally on the device, allowing for speedier analysis and decision-making in real time.
Edge AI employs algorithms designed to function on tiny, low-power devices with limited computing power. These algorithms extract useful information from data gathered by sensors, cameras, and other edge devices.
Edge AI has advantages like decreased latency, increased privacy and security, and lower network capacity needs. Edge AI systems can examine data in real time by processing it locally, allowing quicker decision-making and faster response times. Furthermore, because data is handled locally on the device, it is more secure and less prone to cyber threats than data transferred to a central server for processing.
Edge AI is employed in various applications, including retail surveillance, industrial automation, self-driving cars, and smart cities. Edge AI's potential applications will rise as edge devices become more powerful and common, making it a key technology for the future of AI and the Internet of Things (IoT).
What is Retail Surveillance?
Retail Surveillance is described in the following paragraphs:
The Need for Retail Surveillance
It is essential for several reasons, including:
-
Crime prevention: Retail establishments are prone to various crimes, including theft, burglary, and staff theft. Retail surveillance systems aid in deterring criminal behavior and providing proof of a crime.
-
Safety: It may help guarantee the safety of customers and staff by monitoring for possible safety concerns such as spills or other incidents. It may also be used to watch for suspicious conduct that could suggest a possible threat to one's safety.
-
Operational efficiency: It may monitor shop operations and detect inefficiencies, helping merchants streamline their procedures and increase profits.
-
Customer experience: It may monitor consumer behavior and preferences, allowing shops to make customized recommendations and increase customer happiness.
-
Compliance: It can assist shops in meeting legislation and industry requirements such as data protection and security.
-
Loss prevention: Retailers lose billions of dollars yearly due to theft, fraud, and other losses. It can decrease these losses by watching for suspicious activity and notifying security staff in real time.
-
Liability protection: Retail surveillance can provide proof of a liability claim, such as a slip-and-fall accident or customer injury. This can help shops avoid expensive litigation and insurance claims.
-
Team member monitoring: It may monitor staff conduct and ensure corporate policies and procedures are followed. This can help reduce team member theft and increase efficiency while ensuring that staff provide excellent customer service.
Types of Retail Surveillance
It aims to improve safety and security, prevent theft and other losses, and enhance customers' shopping experience. Several retail surveillance systems include video, access control, and alarm systems.
There are several types of retail surveillance, including:
-
Video surveillance: The most prevalent surveillance involves deploying cameras to monitor consumer behaviour and business operations. Cameras can be stationary or mobile, including motion detection, facial recognition, and license plate identification.
-
Access control systems: Access control systems limit access to specific shop parts, such as stockrooms or cash registers. They may utilise critical cards, biometric authentication, or other means to verify identification and control access.
-
Alarm systems notify security staff of a security breach or other emergency. Motion sensors, door contacts, and other sensors can set off alarm systems.
-
Point-of-sale (POS) monitoring: Monitoring at the point of sale (POS) entails monitoring transactions, such as cash registers or self-checkout kiosks. POS monitoring can aid in the detection of fraudulent transactions utilizing stolen credit card information.
-
Radiofrequency identification (RFID): RFID is a technology that employs radio waves to identify and track items through the supply chain and into the retail shop. RFID can aid inventory management and prevent losses caused by theft or missing products.
Current Challenges in Retail Surveillance
Despite the benefits of retail surveillance, retailers still need help implementing these systems. Some of the current challenges in it include:
-
Privacy concerns: Customers and staff have privacy concerns when video surveillance and other monitoring technologies are used. Shops must be open about using surveillance technologies and assure compliance with data privacy rules.
-
Technological limits: Its technology is continually advancing, but these systems still have limitations. For example, facial recognition technology may be more accurate, and cameras may need help to capture good photos in low-light circumstances.
-
Cost: Implementing a complete system may be costly, especially for smaller stores with limited resources. Retailers must weigh the costs of these technologies against the possible benefits they might deliver.
-
Integration: Retail surveillance systems are incompatible with the retailer's other systems or technology. Integration can be complex, especially for merchants with complicated IT systems.
-
Cybersecurity: Its systems are exposed to cyber dangers like hacking or malware. Shops must guarantee that their surveillance systems are secure and that their data is safe.
A distributed computing paradigm in which processing and computation are performed mainly on classified device nodes. Taken From Article, Edge Computing and its Impact on IoT
Edge AI Solutions for Retail Surveillance
Because of the rising demand for real-time, precise identification and reaction to security risks, edge AI solutions are becoming increasingly critical for it. Retailers may use these technologies to analyze data locally, decreasing latency and boosting decision-making skills. Retailers may use Edge AI to incorporate advanced surveillance techniques, including object and facial recognition, queue management, predictive analytics, and automated alarms. These technologies can assist shops in reducing theft and fraud losses, optimizing team member numbers, and improving the overall consumer experience. As a result, Edge AI is rapidly becoming a vital tool for merchants seeking to create effective systems capable of addressing existing and future security risks. Retailers can implement several Edge AI solutions for retail surveillance to improve security and reduce losses. Some examples include:
-
Object recognition: Edge AI systems may be used in real-time to recognize items such as products or shopping carts. This can aid merchants with the detection of suspect conduct, such as stealing or abandoned products.
-
Face recognition: Edge AI systems may be used in real-time to identify individuals, such as known shoplifters or workers. This can assist merchants in monitoring suspicious activity and reducing theft losses.
-
Queue management: Edge AI systems may be utilized in real-time to monitor consumer flow and wait lengths. This can assist stores in optimizing workforce numbers and decreasing client wait times.
-
Predictive analytics: Edge AI systems may examine previous data to forecast future behavior, such as when and where theft is most likely. This can assist merchants in dealing with security issues and reducing losses proactively.
-
Automated alerts: Edge AI systems can be designed to generate warnings automatically when specified events occur, such as when an item is moved or when a queue reaches a given length. This can assist shops in responding rapidly to security concerns and improving customer service.
Use Cases for Edge AI in Retail Surveillance
Below described are the Use Cases of Edge AI in Retail Surveillance:
Object Detection and Recognition
Object detection and recognition effectively use Edge AI in retail surveillance. The following are the fundamental processes for implementing object detection and recognition:
-
Data collection: To train their Edge AI models, retailers must gather vast data, such as photos or video footage. This information must be diversified and indicative of the retail environment.
-
Data annotation: Retailers must annotate their data by marking things of interest in photos or video recordings, such as products or shopping trolleys. The Edge AI models are trained using this annotated data.
-
Model training: With the annotated data, retailers must utilize machine learning methods to train their Edge AI models. This entails choosing and fine-tuning algorithms to improve object detection and recognition accuracy and speed.
-
Model deployment: To allow real-time item detection and recognition in their retail environment, retailers must install their Edge AI models on local devices such as cameras or sensors.
Several businesses are already using object detection and recognition in retail surveillance systems. As an example:
-
Walmart: Walmart has incorporated object detection and identification in its shops to determine when a product's shelf space is running short and needs to be replenished. The technology monitors the shelves using cameras and alerts personnel when supplies run short.
-
Amazon Go: Amazon Go is a cashier-less shop that tracks clients and their purchases using object detection and identification. The technology tracks consumers using cameras and sensors as they walk about the store and automatically charges them for the things they remove.
-
Zara: It has incorporated object detection and identification in its stores to monitor client behavior and enhance shop layouts. By using cameras to measure client foot traffic and interactions with the merchandise, Zara can discover areas for improvement and improve the customer experience.
Behavioural Analysis and Anomaly Detection
Edge AI applications in retail monitoring include behavioral analysis and anomaly identification. The following are the fundamental processes for implementing behavioral analysis and anomaly detection in retail surveillance:
-
Data collection: To train their Edge AI models, retailers must gather enormous volumes of data, such as video footage. This data must be varied and indicate customer and team member behavior in the retail environment.
-
Data annotation: Retailers must annotate their data by marking relevant behaviors in video footage, such as queue formation, product engagement, or suspicious actions. The Edge AI models are trained using this annotated data.
-
Model training: With the annotated data, retailers must utilize machine learning methods to train their Edge AI models. This includes choosing and fine-tuning algorithms to improve behavioral analysis and anomaly identification accuracy and speed.
-
Model deployment: To allow real-time behavioral analysis and anomaly detection in their retail environment, retailers must install their Edge AI models on local devices such as cameras or sensors.
Several firms are already using behavioral analysis and anomaly detection in retail surveillance systems. As an example:
-
H&M: To monitor consumer behavior and optimize shop layouts, H&M has deployed behavioral analysis and anomaly detection in its stores. The system tracks consumer foot traffic, product interactions, and other activities using cameras and sensors, allowing H&M to discover areas for improvement and improve the customer experience.
-
Adidas: To detect suspect actions such as theft or fraud, Adidas has adopted anomaly detection in its stores. The technology employs cameras and sensors to monitor consumer and team member behavior and notify security personnel when odd behavior is observed.
-
Sephora: To analyze client interactions with items and deliver individualized suggestions, Sephora has adopted behavioral analysis in its shops. The technology employs cameras and sensors to track client activity, such as product interactions and time spent in various shop sections, allowing Sephora to make tailored suggestions and improve the customer experience.
Responsible Artificial Intelligence brings many practices together in AI systems and makes them more reasonable and trustable. Taken From Article, Responsible AI Principles and Challenges
Queue Management
Queue management is a critical use of Edge AI in retail monitoring. The following are the fundamental stages for implementing queue management in retail surveillance:
-
Data collection: Shops must gather video footage from cameras or sensors installed where long lines are likely to form. This data must be diversified and indicate the retail environment's client flow.
-
Data annotation: Retailers must annotate their data by designating queues of interest in video footage, such as checkout lines, product return lines, or customer service lines. The Edge AI models are trained using this annotated data.
-
Model training: Retailers must use machine learning algorithms to train their Edge AI models using the annotated data. This involves selecting and fine-tuning the algorithms to optimize the accuracy and speed of queue management.
-
Model deployment: Retailers must install their Edge AI models on local devices such as cameras or sensors to provide real-time queue management in their retail environment.
Some companies are already incorporating queue management into their retail monitoring systems. As an example:
-
Walmart: Walmart has introduced queue management in its locations to cut customer wait times. The technology monitors the number of people in each queue. It estimates the wait time using cameras and sensors, allowing Walmart to send additional personnel to popular areas and improve checkout lines.
-
Target: To improve customer service queues and minimize wait times, Target has introduced queue management in its shops. The technology monitors customer activity in the customer service area using cameras and sensors, allowing Target to change team member numbers and offer new service desks as needed.
-
Tesco: To optimize checkout lines and cut wait times, Tesco has deployed queue management at its shops. The technology monitors customer traffic in the checkout area with cameras and sensors, allowing Tesco to change team member levels and establish new checkout lanes as needed.
Staff Monitoring and Performance Optimization
Another critical use of Edge AI in retail surveillance is staff monitoring and performance optimization. The following are the fundamental steps for implementing staff monitoring and performance optimization:
-
Data collection: To train their Edge AI models, retailers must gather vast data, such as camera footage and personnel work schedules. This data must be varied and indicate team member behavior in retail.
-
Data annotation: Retailers must annotate their data by marking staff activities of interest in video footage, such as customer interactions or replenishing shelves. The Edge AI models are trained using this annotated data.
-
Model training: With the annotated data, retailers must utilize machine learning methods to train their Edge AI models. This entails choosing and fine-tuning algorithms to improve the accuracy and speed of staff monitoring and performance optimization.
-
Model deployment: Retailers must install their Edge AI models on local devices such as cameras or sensors to allow real-time staff monitoring and performance improvement in their retail environment.
Some firms are already using staff monitoring and performance optimization in their retail surveillance systems. As an example:
-
Amazon: At its warehouses, Amazon utilizes staff monitoring and performance optimization to track team member productivity and decrease mistakes. The technology monitors staff movements and actions with cameras and sensors, allowing Amazon to discover areas for improvement and optimize operations.
-
Starbucks: To manage team member schedules and improve customer service, Starbucks utilizes staff monitoring and performance optimization in its shops. The system monitors customer traffic and team member interactions with cameras and sensors, allowing Starbucks to adjust staffing levels and improve training.
-
Zara: Zara employs staff monitoring and performance optimization in its stores to increase team member efficiency and decrease wait times. By using cameras and sensors to track personnel movements and consumer traffic, Zara can discover areas for improvement and optimize operations.
Monitor and maintain surveillance systems, particularly when dealing with many cameras. Click to explore about our, AI Powered Video Analytics
What are the best practices for Edge AI in Retail Surveillance?
Below are the best practices for implementing Edge AI in Retail Surveillance:
Hardware Requirements
When implementing Edge AI in retail surveillance, several hardware requirements must be considered to ensure the success of the deployment. These include:
- Processing power: Edge AI typically requires high computing power to execute complex algorithms and machine learning models in real-time. However, to optimize the deployment of Edge AI solutions in retail surveillance, it is essential to consider hardware components that require less processing power. For instance, retailers can consider using specialized processors like ASICs or SoCs designed to accelerate AI workloads, enabling fast and accurate data processing. This can minimize energy costs and reduce hardware requirements to deploy Edge AI successfully.
- Memory: Edge AI models require considerable memory to store and analyze data. Retailers should ensure that their devices have sufficient memory to support these demands.
-
Storage: Edge AI models create tremendous data, which merchants must save for analysis and further training. Retailers should consider employing high-capacity storage options such as solid-state drives (SSDs) to allow rapid and effective data storage and retrieval.
Data Management
Data management is a vital factor to consider. Shops must ensure that their Edge AI systems can manage and retain the massive amounts of data generated by surveillance cameras. Companies should also consider adopting compression techniques to limit the amount of data that must be transferred and stored and encrypt critical data. Moreover, merchants must implement robust data governance procedures to guarantee that data is managed correctly and utilized by legislation and ethical standards. It is essential to handle data efficiently to avoid substantial privacy and security problems and impede Edge AI's usefulness in retail monitoring.
Privacy and Legal Concerns
Using Edge AI in retail surveillance presents privacy and legal considerations merchants must solve to secure regulatory compliance and consumer privacy. To ensure personal data, retailers should consider employing privacy-enhancing technologies such as anonymisation and redaction. They should also set explicit data usage and retention rules and verify compliance with relevant data protection legislation. Retailers should also consult with legal professionals to understand the legal implications of Edge AI in retail surveillance and assure compliance with all applicable laws and regulations. Failing to handle privacy and legal concerns can result in severe reputational harm and legal penalties.
Cost Considerations
Edge AI use in retail surveillance might be pricey. While deploying Edge AI, retailers should consider the hardware, software, and staff expenses. Using open-source Edge AI frameworks and tools is one way to reduce implementation costs. Also, merchants should consider introducing Edge AI solutions in stages, beginning with a few use cases and gradually expanding. This enables a more targeted and cost-effective deployment strategy. While analysing the cost-effectiveness of Edge AI solutions, retailers should examine the total ownership cost, including maintenance and upgrade charges.
Retail surveillance is vital to retail operations since it assists merchants in monitoring and optimising their business operations. Retailers now have a tremendous tool for boosting the accuracy, efficiency, and efficacy of their surveillance systems, thanks to the rise of Edge AI. Edge AI allows for real-time data processing and analysis, allowing shops to make educated decisions and adapt rapidly to changing circumstances. On the other hand, Edge AI solutions need careful consideration of hardware, software, data management, privacy, regulatory problems, and economic concerns. Retailers who integrate Edge AI into their surveillance systems may gain a competitive edge and boost their bottom line by improving the customer experience, minimising shrinkage, and streamlining their operations.
- Read here about Edge AI vs Federated Learning
- Explore IoT Edge Analytics and the Latest Trends
Next Steps with Retail Surveillance
Talk to our experts about implementing compound AI system, How Industries and different departments use Agentic Workflows and Decision Intelligence to Become Decision Centric. Utilizes AI to automate and optimize IT support and operations, improving efficiency and responsiveness.