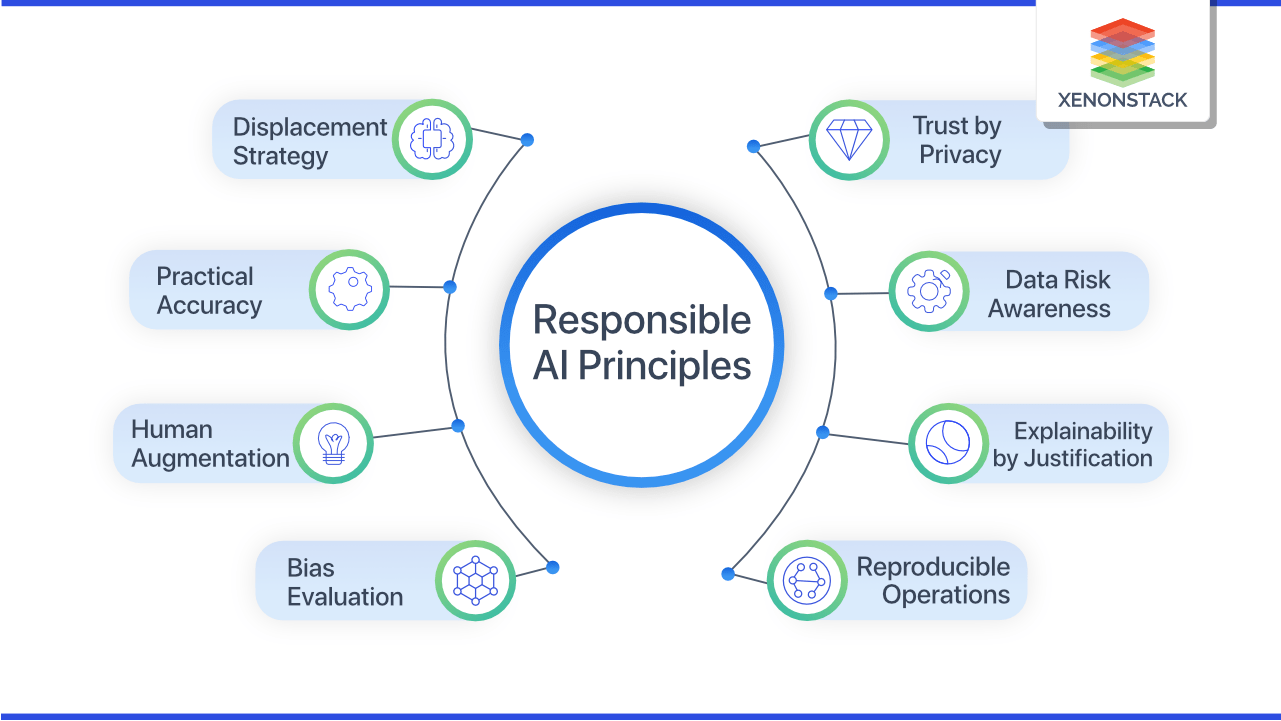
Overview of Responsible AI
Enterprises, businesses, government sector, and workers are continuously exploring new ways to remain operational in the COVID-19 pandemic. Nationwide lockdowns, stay-at-home related orders, border closures, and several other measures taken at various levels to fight with viruses that made the working environment more complicated than before.
Businesses are moving towards Artificial Intelligence (AI) based technology-oriented solutions and data to formulate processes that can function efficiently. The Government started the process of facial recognition by cameras to identify and track people that are travelling from a virus-affected area. In some countries, police are using drones to impose stay-at-home orders for patrolling as well as broadcasting important information. At airports and railways, AI-based face mask detection systems are implemented that raise the alarm to concerned departments if it detects a person without a face mask. In malls, restaurants or other crowded places where it isn't easy to track social distancing manually. The Government imposed AI-based systems that are continuously monitoring the real-time status of buildings and raising an alert if any zone needs special attention.
AI is the reproduction of intelligent human processes, especially machines and computer systems. Taken From Article, Artificial Intelligence Adoption Best Practices
What are the Principles of Responsible AI?
The eight principles that should follow to make AI responsible and support technologies while designing, developing or managing systems that learn from data.
Human Augmentation
When we introduced AI to automate the human task using machine learning systems, we should consider the impact that occurs due to wrong predictions in end-to-end automation. Developers and analysts should understand the outcomes of incorrect predictions, especially when they are automating critical processes that can have a vital impact on human lives (e.g. Finance, health, transport, etc.).
Bias Evaluation
When building AI-enabled systems that have to make crucial decisions, there is always a chance of bias, i.e., computational and societal bias in data. It is not possible to avoid data having a bias issue. Technologists should document and mitigate bias issues, instead of embedding ethics directly into the algorithms. Their focus should be on documenting the inherent bias in the data and features while building processes & methods to identify features and inference results so the right procedures can be put in place to lessen potential risks.
Explainability by Justification
With the hype in the use of Machine learning and deep learning models, developers usually put large amounts of data into ML pipelines without having any understanding of how the pipelines will work internally. Technologists should continuously improve processes to explain the predicted results based on features and models chosen. In some cases, the accuracy may decrease, but the transparency and explainability in processes help to make significant decisions.
The implementation of ethics is crucial for AI systems to provide safety guidelines that can prevent existential risks for humanity. Click to explore our, Ethics of Artificial Intelligence
Reproducible Operations
Machine learning systems at production don't have the abilities to diagnose the situation when something terrible happened and respond effectively with a model. In production systems, it is vital to perform standard procedures, such as reverting a Machine learning model to a previous version or reproducing an input to debug a specific functionality. Developers should use the best practices in the tools and processes of machine learning operations. Reproducibility of machine learning systems helps to archive data at each step of end to end pipelines.
Displacement Strategy
When the organization starts using automation in tasks using AI systems, then that impact will be vigilant at the industry level as well on multiple individuals or workers. Technologists should support the necessary stakeholders in developing a change management strategy by identifying and documenting relevant information. Developers should use the best practices to structurize and put related documents in place.
Practical Accuracy
When building systems using Machine learning capabilities, it is necessary to obtain an accurate understanding of the business requirement to assess the accuracy and align cost-metric functions to the domain-specific applications.
Trust by Privacy
When industries are automating work at large-scale, there are a large number of stakeholders that may get affected directly and indirectly. Building trust within stakeholders is not possible by informing them only about what data is being held, but also to explain the process as well as the requirement of protecting the data. Technologists should implement privacy at all levels to build trust among users, relevant stakeholders.
Data Risk Awareness
With the rise in Autonomous decision-making systems, it opens the doors to new potential security breaches as well. 70% of security breaches occur only due to human error instead of having actual hacks, i.e. accidentally sending essential data to someone via mail.
Technologists should work on security risks by establishing processes around data as well as by educating personnel and assessing implications of ML backdoors.
Ethical issues available in the system can only be recognized if data and algorithms are fully understood. Discover about Real-Life Ethical Issues of Artificial Intelligence
AI Adoption will not work under these circumstances
Some of the scenarios where AI is not able to respond appropriately.
- Google facial detection system is tagging black people as a gorillas
- Models trained on Google News come up with the conclusions "man is meant to programmer and a woman is meant to be a homemaker".
- Image recognition models being trained on a dataset related to stock photos where most images are related to women working in the kitchen, that when the image of man comes in a kitchen to identify. It predicts the man also as a woman.
Such data-driven approaches when misused or behave abruptly, then it might be harmful to human rights. There should be a sense of responsibility in data-driven strategies. To make responsible AI, it is essential to adopt ethical principles with proper planning.
It will ensure AI-based models against the use of biased data or algorithms and give decisions or insights that are justified and explainable along With the maintenance of user's trust and individual privacy.
"Why should I trust AI?" For instance, if an AI-based disease diagnosis system uses a neural network to help the doctor in disease diagnosis, a doctor can't go to a patient and say, "Oh so sorry, you got cancer." The patient, will ask "How do you know?" And the doctor here isn't able to say, "I don't know, the AI system told me so." It doesn't quite work that way. The AI system should be liable to provide some explanation related to the outcome to the doctor.
Is Responsible AI compatible with Business?
Responsible Artificial Intelligence brings many practices together in AI systems and makes them more reasonable and trustable. It makes it possible to use transparent, accountable, and ethical AI technologies consistently w.r.t user expectations, values, and societal laws. It keeps the system safe against bias and data stealing.
End-users want a service that can solve their issue and accomplish objectives. Together with the peace of mind knowing that the system is not unknowingly biased against the particular community or group of people. Moreover, protecting their data according to the laws from theft and exposure. Meanwhile, businesses are exploring AI opportunities and educating themselves about public risk.
Adopting Responsible Artificial Intelligence is also a big challenge for businesses and organizations. It is usually mentioned that the use of Responsible AI is incompatible with the business. We will discuss the reasons why it is said that Responsible Artificial Intelligence is incompatible with business. Let's discuss them:
- There is a broad agreement on the Responsible Artificial Intelligence principles that helps to understand how to implement them. However, many organizations are still not aware of how to effectively put them into practice.
- Many people think these are only verbal things that only need to talk about; they think of AI Ethics because they don't have clear visibility of the solution as it is a new term and not matured yet.
- It isn't easy to convince stakeholders and investors to invest in this technology as a new term. They cannot see how a machine can fully act as a human while making decisions.
- So the business thinks that Responsible Artificial Intelligence slows down the innovation by wasting time convincing people and giving them a vision of why this is required and how it is possible.
An approach of proper governance, transparency, and a thoughtfully conceived process based on AI decision-making responsibilities. Source: Responsible Artificial Intelligence in Government
What are the Responsible AI Adoption Challenges?
Some key challenges that need to address for successful adoption of AI:-
- Explainability and Transparency: If AI systems are opaque and not able to explain themselves as to why or how specific results are generated, this lack of transparency and explainability will threaten Trust in the system.
- Personal and Public Safety: Use of autonomous systems such as self-driving cars on roads and robots could be a risk of harm to humans. How can we assure human safety?
- Automation and Human Control: If AI systems can generate Trust and support humans in tasks and offloads their work. There will be a risk of threatening our knowledge related to those skills. This will make it more complex to check the reliability, correctness and result of these systems as well as makes human intervention impossible. How do we ensure human control on AI systems?
- Bias and Discrimination: Even if AI-based systems work neutrally, it will give insights on whatever data it is trained. Therefore, it can be affected by human and cognitive bias, incomplete training data sets. How can we make sure that the use of AI systems does not discriminate in unintended ways?
- Accountability and Regulation: With the increase of AI-driven systems in almost every industry, expectations around responsibility and liability will also increase. Who will be responsible for the use and misuse of AI systems?
- Security and Privacy: AI systems have to access vast amounts of data to identify patterns and predict results that are beyond human capabilities. Here, there is a risk that the privacy of people could be breached. How do we ensure that the data we are using to train AI models are secure?
How can businesses successfully deploy Responsible AI?
How can a business implement AI at scale while reducing the risks? You should undertake a significant organizational reform to transform your business into an ethical AI-driven one.
We provide the following procedure as a starting point to assist in navigating that change:
- Define responsible AI for your business: Executives must define what constitutes appropriate use of AI for their company through a collaborative approach that involves board members, executives, and senior managers from across divisions, to ensure that the entire organization is moving in the same direction. This may be a collection of rules that direct the creation and application of AI services or goods. Such principles should be organized around a practical reflection on how AI can add value to the organization and what risks (such as increased polarisation in public discourse, brand reputation, team member safety, and unfair customer outcomes) must be mitigated along the way.
- Develop organizational skills: Developing and implementing reliable AI systems must be company-wide. Driving the adoption of responsible AI practices calls for thorough planning, cross-functional and coordinated execution, staff training, and sizable resource investment. Companies could establish an internal "Centre of AI Excellence" to test these initiatives, focusing their efforts on two essential tasks: adoption and training.
- Promote inter-functional collaboration: Because risks are highly contextual, they are perceived differently by different company departments. To create a sound risk prioritization plan, include complementary viewpoints from diverse departments while building your strategy. As a result, there will be fewer "blind spots" among top management, and your employees will be more supportive of the implementation.
Additionally, hazards will need to be managed while the system is in operation because learning systems tend to lead to unexpected behaviors. Close cross-functional cooperation, managed by risk and compliance officers, will be essential for devising and executing efficient solutions in this situation. - Use more comprehensive performance metrics: AI systems are frequently evaluated in the industry based on their average performance on benchmark datasets. However, experts in AI agree that it is a relatively limited approach to performance evaluation and are actively looking into alternatives. We advocate a more comprehensive strategy in which businesses regularly monitor and evaluate their systems' behavior in light of their ethical AI standards.
- Establish boundaries for responsibility: If the proper lines of accountability are not established, having the proper training and resources will not be sufficient to bring about a sustainable transformation. The two possible solutions can be:
- Implement a vetting procedure, either as part of your AI products' pre-launch assessment or separately from it. The duties and responsibilities of each team involved in this vetting process should be mapped out in an organizational framework, and an escalation method should be used when/if there is a persistent disagreement, such as between the product and privacy managers.
- Second, as part of their annual performance evaluation, employees who have reported problematic use cases and taken the effort to implement corrective steps ought to be recognized.
Businesses should welcome this change since it will define who is worth doing business with.
ModelOps (or AI model operationalization) is focused primarily on the governance and life cycle management of a wide range of operationalized artificial intelligence. Click to explore our, Deep Learning: Guide with Challenges and Solutions
What are the AI challenges across Five Key Dimensions?
Responsible AI focuses on 5 Key dimensions to handle AI challenges these are:
Governance
Governance is an End-to-end base for all other dimensions. It can answer the following questions:
- Who is accountable for AI decisions?
- How do AI applications can be aligned with the strategy of the business?
- What is required to change to improve the model outputs?
- How can system performance be tracked?
- Are application outputs consistent or reproducible?
As the process of AI is iterative, therefore the AI governance should also be iterative as well. A more flexible and adaptable form of governance can answer the above questions better and respond to the applications' outcomes. A successful governance foundation will do strategy and planning across the organization by taking into account the vendor ecosystem and capabilities. Also, follow the unique model development, monitoring, and compliance process.
Ethics and Regulation
- AI applications not only have to help the organization automate the processes. But should develop that are responsible and respect human ethics and morals.
- Proper ethical consideration and regulation for an organization make them able to identify AI solutions' ethical implications. By considering a defined set of principles into account carefully helps to mitigate the ethical risks.
Interpretability and Explainability
Different system stakeholders may require different explanations of how the system reaches a decision. Lack of transparency and interpretability in systems can frustrate the customers. It can cause operational, reputational, and financial risks. Therefore, It is necessary to have a justification of the application's decisions. Moreover, the explanation that the system will provide must be understandable to various stakeholders.
Robustness and Security
- AI applications should be secure, safe, and resilient to work effectively. The system must have the built-in capability to detect and correct faults, inaccurate and unethical decisions.
- AI applications use data to make decisions. So, data may be confidential; therefore, applications should be secured so that no one can harm them.
Bias and Fairness
- Bias is the most identified and trending issue of AI applications. There are various real-life examples of the application that encountered such issues. Such as the Apple Card is a system that is biased against gender. It offered more credit limits to the menu than the women having the same values or parameters.
- Reasons for bias in a system can be due to data. It can also be algorithmic bias because these applications are trained on historical data that can have bias. These biases can be mitigated using some approaches and make the application fair.
What are the Benefits of Responsible AI?
- Minimizing Bias in AI Models: Implementing responsible AI can ensure that AI models, algorithms, and the underlying data for building AI models are unbiased and representative. This can ensure better results and reduce data and model drift. From an ethical and legal point of view, this can minimize the harm to the users that can be otherwise affected due to a biased AI model’s results. Responsible AI Principles Squad 7.
- AI Transparency and Democratization: Responsible AI enhances transparency and explainability of models. This builds and promotes trust among organizations and their customers. It also enables the democratization of AI for both enterprises and users.
- Creating Opportunities: Responsible AI empowers developers and users to raise doubts and concerns with AI systems and provides opportunities to develop and implement ethically sound AI solutions.
- Privacy Protection and Data Security: Responsible AI takes priority over the premise of privacy and security of data to ensure personal or sensitive can never be used in any unethical, irresponsible, or illegal activity.
- Risk Mitigation: Responsible AI can mitigate risk by outlining ethical and legal boundaries for AI systems that can benefit stakeholders, employees, and society.
Self-driving cars main goal is to provide a better user experience and safety rules and regulations. Click to explore our, Role of Edge AI in Automotive Industry
What are the Best Practices of Responsible AI?
- AI solutions should be designed to have a human-centric approach. Appropriate disclosure should be provided to users.
- Model deployment should be preceded by proper testing. Developers need to account for a diverse set of users and multiple use-case scenarios.
- A range of metrics should be employed to monitor and understand AI solutions' performance, including feedback from the end users.
- Metrics should be selected concerning the context and goals of AI solutions and business requirements.
- Data validation should be performed periodically to check for inappropriate values, missing values, biasedness, training skew, or to detect drift.
- Limitations, flaws, and potential issues should be properly addressed and communicated to stakeholders and users.
- A rigorous testing procedure should be in place. Unit tests to test individual components of the solutions. Integration tests to test seamless interaction between the components and quality and statistical tests to check for data quality and drift.
- Track and continuously monitor all deployed models. Compare and log model performance and update deployed model based on changing business requirements, data, and system performance.
Is Responsible AI slowing down Innovation?
Undoubtedly, adopting and implementing Responsible Artificial Intelligence can slow down the process, but we cannot say that it is slowing down innovation. Using AI systems without Responsible, ethical, and human-centric approaches could be a fast race but no longer belong. If these systems start working in opposing human morals, ethics, and rights, people will no longer keep using them.
"I don't think we should spend time talking to people. They don't understand this technology. It can hinder progress."
Some people think that Responsible AI takes a lot of time; it wastes time and hampers innovation. Hence, Leave things in the way they are. But, Responsible Artificial Intelligence is a new term, so it is required to give the people the vision needed. It could be challenging to convince people and provide them with a picture, but it will deliver more innovative and robust systems later on. We need to tell them taking things with care would take time. No doubt, creating relationships with partners and stakeholders takes time. It will result in a human-centric AI. Slowing innovation is committed to providing human-centric solutions that protect humans' fundamental rights and follow the rule of law. It will promote ethical deliberation, diversity, openness, and societal engagement.
Future of Responsible AI
People are looking for an approach that could be used to anticipate rather than react to risks. A standard process, communication, and transparency are required to achieve that. Therefore, demand for general and flexible Responsible Artificial Intelligence is also rising because its framework can handle different AI solutions, such as Predicting credit risk or video recommendation algorithms. The outcome that it will provide is understandable and readable for all types of people or stakeholders. So that respective audiences can use that outcome for their purpose. For instance, end-users may be mean for justification of decisions and how they can report incorrect results.
A Holistic Strategy
AI potentially have many risks to human rights, more than a risk for human values, Responsible Artificial Intelligence and its principles brings in itself enough potential to make better the lives of many, and to ensure human rights to all.
- Learn more about Enabling Artificial Intelligence (AI) Solutions on Edge
- Explore more on Explainable Artificial Intelligence