What are AI Agents?
AI agents are intelligent software systems capable of sensing their environment, processing input data, and executing actions autonomously to achieve specific goals. In the automotive space, these agents are used for in-vehicle applications and supporting backend systems. When integrated with Edge AI, they form an agile system that learns from and adapts to real-time driver behaviour and external conditions.
Main Types of Automotive AI Agents:
-
Autonomous Driving Agents: For self-driving capabilities, control vehicle motion—steering, acceleration, braking.
-
Voice & Conversational Agents: Employ natural language processing (NLP) to interpret driver commands and assist with navigation, calls, and music.
-
Health Monitoring Agents: Monitor mechanical systems and predict failures based on pattern recognition and sensor anomalies.
-
Personalization Agents: Learn user habits and preferences to optimize in-car settings and user experience dynamically.
Real-World Applications in Automotive Engineering
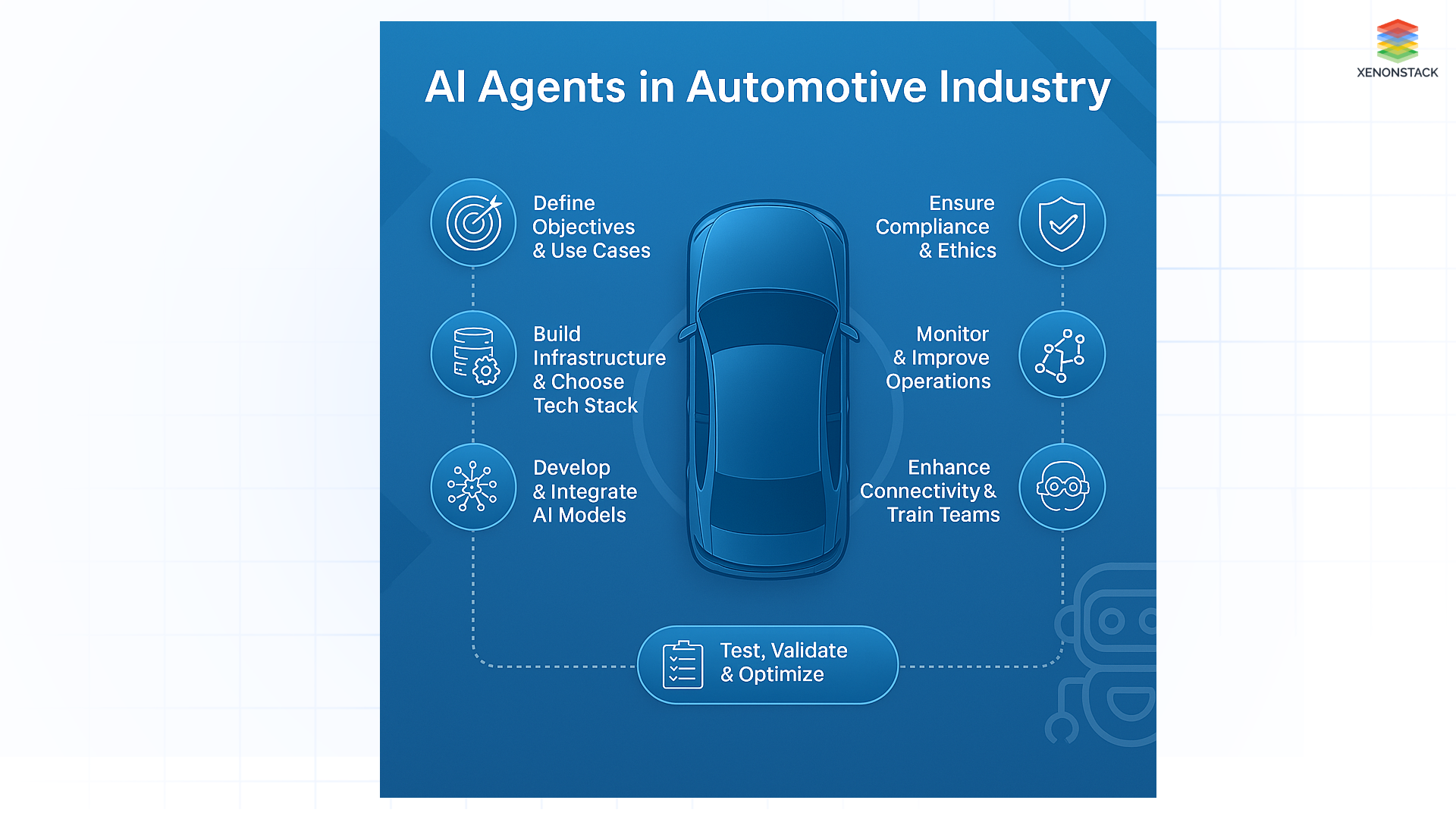
1. Advanced Driver Assistance Systems (ADAS)
ADAS uses a combination of lidar, radar, ultrasonic sensors, and edge computing to enable functions such as:
-
Adaptive cruise control
-
Lane-keeping and departure warnings
-
Automatic emergency braking
-
Blind-spot detection
-
Pedestrian and cyclist avoidance
Startups like DeepRoute.ai, working alongside chipmakers like Qualcomm, build scalable ADAS solutions powered by efficient edge-based sensor fusion and AI.
2. Predictive Maintenance and Remote Diagnostics
Edge AI and AI agents work together to analyze vehicle sensor data in real-time, identifying potential issues before they become major failures. Key benefits include:
-
Minimized vehicle downtime
-
Lower maintenance costs
-
Real-time diagnostic alerts via dashboard or mobile apps
-
Fleet-wide monitoring for logistics and transportation companies
This technology allows fleet managers to remotely manage repairs, optimize service intervals, and prevent breakdowns through predictive alerts.
3. Smart In-Car Experiences and Infotainment
AI agents contribute to highly personalized and adaptive in-cabin environments. They learn from driver behaviour and preferences to:
-
Automatically adjust seat positions and climate settings
-
Navigate to preferred destinations using habitual patterns
-
Curate music, podcasts, and media based on taste
-
Seamlessly integrate voice assistants like Alexa and Google Assistant
Mercedes-Benz, through its MBUX system, has incorporated Google’s AI technologies to create intuitive, conversational, and context-aware interfaces across its vehicles.
4. Driver Monitoring and Health Assessment Systems
Using interior-facing cameras and biosensors, Edge AI evaluates driver attention, fatigue, and stress levels by tracking indicators such as:
-
Eye movement and blinking patterns
-
Heart rate variability
-
Facial expressions
These systems issue real-time warnings, suggest rest stops or temporarily activate autonomous driving features if fatigue is detected—thus helping reduce accident rates.
5. Vehicle-to-Everything (V2X) Communication
V2X systems, powered by AI agents and edge computing, allow vehicles to communicate with:
-
Traffic lights: To anticipate signal changes and improve flow
-
Other cars: For coordinated driving and accident avoidance
-
Infrastructure: For real-time road condition updates
-
Pedestrians and cyclists: For dynamic rerouting and enhanced safety
As 5G networks expand, integrating Edge AI in V2X systems will dramatically improve responsiveness and reliability, especially in urban mobility.
AI in Automotive Manufacturing and Logistics
Edge AI and AI agents extend beyond vehicle usage into production and supply chain management. In smart factories and assembly lines, AI is being used for:
-
Visual Quality Control: High-resolution cameras and vision models identify microscopic defects on parts.
-
Flexible Robotics: AI-driven robotic arms can adjust movements based on real-time data and component irregularities.
-
Demand Forecasting: AI models anticipate parts shortages and delivery delays by analyzing real-world factors like climate events and global trade issues.
-
Sustainability Monitoring: Energy consumption, water usage, and material waste are optimized in real-time.
Notable Industry Implementations
Ford
Ford uses Nvidia’s AI and GPU stack to model digital twins of its vehicle systems. Engineers use these twins to simulate real-world conditions and optimize vehicle design, testing, and deployment cycles.
Nio
The Chinese EV manufacturer Nio has developed an in-car AI companion named Nomi. It delivers personalized interactions, emotional responses, and context-aware suggestions, creating an engaging driver experience.
Stellantis
Through a partnership with SoundHound AI, Stellantis vehicles feature conversational voice systems that allow hands-free control of essential functions like AC, navigation, and audio.
Toyota
Toyota integrates Nvidia’s DRIVE platform into its autonomous vehicles to enable high-performance computing and real-time decision-making for autonomous navigation.
Key Challenges and Considerations in the Automotive Industry
Even as the benefits grow, several technical and operational challenges persist:
-
Heat & Power Constraints: Edge processors' thermal and power limitations restrict AI model complexity.
-
Ecosystem Fragmentation: Integrating multiple third-party AI tools into a cohesive vehicle platform can lead to compatibility issues.
-
Cybersecurity Risks: On-device processing can be vulnerable to physical and digital attacks if not properly secured.
-
Compliance Overhead: Meeting varying global safety and data protection standards requires continuous adaptation.
Future Trends Based on Current Market Insights
According to current search trends and market behaviour, these developments are gaining traction:
1. Integration of 5G and Edge AI
The convergence of 5G with edge computing enables:
-
Faster, more reliable OTA updates
-
Real-time vehicle-cloud interactions
-
Low-latency AR-based navigation overlays
2. Autonomous Driving at Higher SAE Levels
AI agents will increasingly handle complex environments with minimal human intervention. Companies like Waymo, Cruise, and Tesla are training AI on massive driving datasets to perfect autonomy at SAE Levels 4 and 5.
3. Use of Digital Twins and Simulation
Automotive OEMs are leveraging digital twins to:
-
Run safety simulations
-
Validate performance under various real-world conditions
-
Predict long-term component wear and tear
4. AI-Driven Green Manufacturing
AI is making factories more sustainable by:
-
Reducing emissions in high-energy processes like welding
-
Predicting carbon footprint per manufactured vehicle
-
Facilitating circular production and reusability
5. Emotionally Intelligent AI Systems
Next-gen in-cabin systems will interpret:
-
The tone and sentiment in the driver's voice commands
-
Visual cues for stress or agitation
-
Situational context to adjust lighting, music, and suggestions
This ushers in a more empathetic and human-centric AI ecosystem within vehicles.
AI Agents in the Automotive Industry
Edge AI and AI agents are transforming every stage of the automotive lifecycle—from design and manufacturing to real-time driving and after-sales service. They are turning vehicles into intelligent, adaptive systems that can think, learn, and respond in ways that were unimaginable a decade ago.
As advancements in AI model optimization, edge hardware, and connected infrastructure accelerate, vehicles will evolve into proactive partners, enhancing safety, personalization, and sustainability. Today's companies investing in this transformation are positioning themselves to lead the next era of intelligent, resilient, and human-centric mobility.