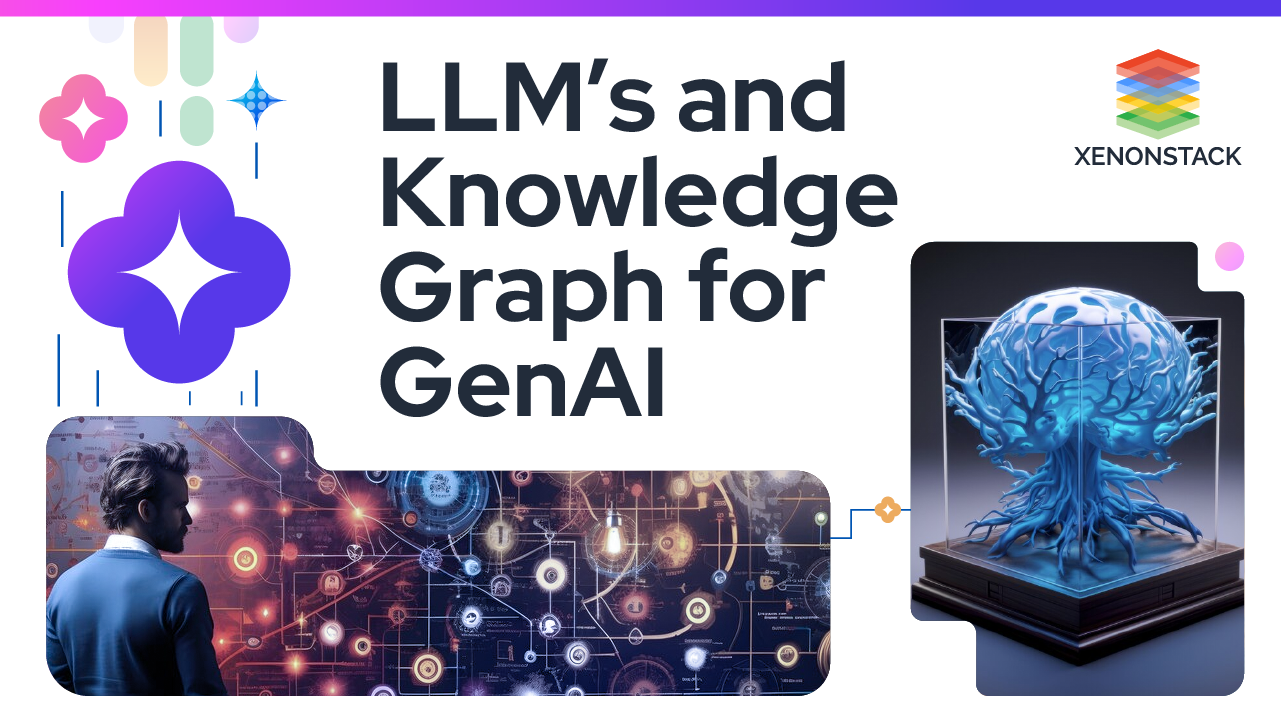
Introduction
The convergence of Large Language Models (LLMs) with Knowledge Graphs (KGs) heralds a groundbreaking evolution in the realm of Generative AI, promising to significantly elevate the way AI understands and generates human-like text. This innovative integration aims to make our interactions with technology more seamless, intuitive, and remarkably akin to human conversation. But the essence of this fusion goes beyond just technological advancement, it represents a pivotal shift towards creating AI systems that can communicate, learn, and provide information with unprecedented accuracy and relevance.
Why use Generative AI with Knowledge Graphs
At its core, this fusion brings together the best of two worlds: LLMs, like GPT-4, which excel in generating coherent, contextually aware language responses from vast amounts of text data, and KGs, which organize complex webs of real-world information into a structured, accessible format. Together, they unlock the potential for AI to not only mimic human conversation but to do so with an informed understanding of the subject matter, bridging the gap between mere language generation and true comprehension. This collaboration between LLMs and KGs is set to redefine the boundaries of AI, transforming it into a tool that understands the nuances of human language and the intricacies of the knowledge it seeks to convey.
Understanding LLMs and Knowledge Graphs
Before diving into their integration, it is essential to grasp what LLMs and KGs are and their unique strengths. LLMs, like GPT-4, are AI systems trained on vast amounts of text data, enabling them to generate coherent, contextually relevant language responses. KGs, on the other hand, are databases that use a graph-structured data model to store interconnected descriptions of objects, concepts, and their relationships. Together, they hold the promise of making AI systems more knowledgeable, versatile, and capable of understanding the nuances of human language.
The Motivation for Integration
The push to unify LLMs and KGs stems from a desire to overcome their respective limitations. While LLMs excel at generating human-like text, they often struggle with accuracy, specificity, and handling information. KGs, conversely, excel in structured knowledge representation but lack the ability to generate fluid, dynamic text. The integration seeks to create a GenAI that combines the best of both worlds: the generative flexibility of LLMs with the factual rigor of KGs.
Key Opportunities
- Enhanced Understanding and Generation of Text
By integrating KGs with LLMs, AI systems can achieve a deeper understanding of text context, enabling more accurate and contextually relevant text generation. This could revolutionize fields like automated journalism, content creation, and even personalized education by providing AI that can understand and generate nuanced, factually correct text.
- Improved Accuracy and Reliability
The factual backbone provided by KGs can significantly enhance the reliability of information generated by LLMs. This is particularly crucial in fields requiring high accuracy levels, such as medical advice, legal assistance, and technical support.
- Customization and Personalization
Combining LLMs' generative capabilities with KGs' structured knowledge allows for personalized content generation at scale. Imagine AI that can tailor educational content to individual learning styles or provide personalized advice grounded in accurate, up-to-date information.
Knowledge Graph using LLM Case Study
Engaging with the Chatbot Application
Users interact with a chatbot application, formulated to understand, and process natural language queries. This is where the prowess of LLMs comes into play. Equipped with the ability to understand human language, the chatbot serves as the interface between the user and the vast repository of flight information stored in the Graph Database.
Translating Natural Language Queries into Graph Queries
When a user poses a question to the chatbot, the underlying LLM technology interprets the natural language query. The real magic happens as this query is then translated into a specific graph query that can navigate the structured data of the Graph Database. This step embodies the seamless integration of LLMs' linguistic capabilities with the structured knowledge representation of KGs.
Querying the Graph Database
With the graph query formulated, the system then proceeds to fetch the relevant data from the Graph Database. This involves querying the database with precise parameters derived from the user's original question, ensuring that the search is as targeted and efficient as possible.
Converting Data into Natural Language Responses
The final step closes the loop, as the information retrieved from the Graph Database is converted back into natural language. This process, once again, leverages the strength of LLMs to articulate the data in a form that is easily understandable by the user. The chatbot then presents this information, completing the interaction cycle with clarity and ease.
Use cases of Gen AI with Knowledge Graph
1. Personalized Education Platforms
Tailoring educational content to individual learning preferences and knowledge gaps. By combining LLMs' ability to generate engaging educational material with KGs' structured knowledge about subjects, concepts, and student progress, platforms can offer personalized learning experiences that adapt to each student's pace and interests.
Example: A platform that customizes study materials for students struggling with algebra by using KGs to identify their weak points and LLMs to generate tailored exercises.
2. Enhanced Search Engines
Improving the accuracy and contextuality of search engine results. Integrating LLMs with KGs allows search engines to understand queries better and provide answers that are not only factually accurate but also contextually relevant.
Example: A search engine that uses KGs to understand the context of "Renaissance art" in a query, ensuring results are focused on historical art movements rather than modern interpretations.
3. Advanced Healthcare Assistance
Providing more accurate and personalized healthcare advice. By using KGs to store detailed medical knowledge and patient data, and LLMs to interpret this information in the context of individual health inquiries.
Example: An AI system that offers specific physiotherapy exercises for a user recovering from knee surgery, combining medical KGs with LLMs for personalized advice.
4. Intelligent Virtual Assistants
Creating more knowledgeable and context-aware virtual assistants. LLMs can generate human-like responses to queries, and when integrated with KGs that contain information about the user's preferences, history, and the world, these assistants can provide highly personalized and accurate information, advice, or assistance.
Example: A virtual home assistant that suggests a week's meal plan based on your past preferences and nutritional goals, utilizing KGs for dietary information and LLMs for recipe generation.
5. Automated Content Creation for Media
Generating accurate and context-rich content for news articles, reports, and marketing materials. Media companies can leverage the integration of LLMs with KGs to produce content that is not only engaging and well-written but also enriched with accurate, up-to-date facts and figures.
Example: Automatically generating a news article about a local election, where KGs provide the latest results and statistics, and LLMs craft a cohesive story.
6. E-commerce Personalization
Enhancing product recommendations and customer service on e-commerce platforms. By understanding customer preferences, purchase history, and product details stored in KGs, LLMs can generate personalized product descriptions, recommendations, and even interact with customers in a more informed and helpful manner, improving the shopping experience.
Example: An online store recommending gardening tools specifically for small spaces, based on a user's previous searches and purchases, using KGs for product details and LLMs for personalized messaging.
7. Smart Legal and Compliance Tools
Automating legal document analysis and compliance checks. LLMs can process and generate complex legal text, but when combined with KGs that store legal codes, case law, and company policies, they can also ensure that documents comply with relevant laws and regulations, streamlining legal workflows.
Example: A compliance tool that automatically updates company policies to reflect new environmental regulations by querying KGs for legal updates and using LLMs to rewrite policy documents.
8. Cultural Heritage Preservation
Documenting and narrating cultural and historical knowledge. Museums and cultural institutions can use LLMs integrated with KGs containing historical facts, artifacts, and narratives to create engaging and informative digital guides, interactive exhibits, or educational materials that bring cultural heritage to life for diverse audiences.
Example: A museum app that provides visitors with detailed backgrounds of exhibits, such as the story behind an ancient Greek vase, using KGs for historical data and LLMs to narrate the information engagingly.
Challenges and Considerations
Blending the dynamic capabilities of Large Language Models (LLMs) with the structured precision of Knowledge Graphs (KGs) is a venture into uncharted technological territories. This integration, while promising, comes with complex challenges and ethical considerations that need to be navigated with care and expertise.
Navigating the Complexity of Integration
Imagine trying to have a free-flowing conversation with someone who speaks in bullet points, listing facts and figures. Now, consider the opposite, engaging with someone whose responses, though elaborate, barely scratch the surface of the topic. The former scenario resembles the structured nature of KGs, while the latter mimics the fluidity of LLMs. Bridging these two approaches requires not just sophisticated technology but a nuanced understanding of both language and data. It is akin to teaching two very different minds to work together as one a task that demands innovative algorithms and a deep dive into the mechanics of AI language and knowledge representation.
Upholding Privacy and Mitigating Bias
The fusion of LLMs with KGs also ventures into the sensitive terrain of data privacy and bias. As we feed AI systems with more information and give them access to vast knowledge bases, the risk of infringing on individual privacy escalates. Furthermore, the data used to train these models can carry inherent biases, inadvertently teaching AI systems the same prejudices. Addressing these issues is not just a technical challenge; it is a moral imperative. Ensuring that AI systems treat all data ethically and navigate the fine line between personalization and privacy invasion requires continuous effort and vigilance from developers and ethicists alike.
Keeping Knowledge Fresh and Accurate
The world is in constant flux, with new information emerging at an unprecedented pace. For Knowledge Graphs to remain relevant and for the integrated system to deliver accurate information, they must be updated continually. This is akin to maintaining a library where books never age, and the catalog always knows the latest publications. However, the scale at which information changes in the digital age makes this task daunting. Ensuring the AI's knowledge base is both current and accurate demands not just automated systems capable of learning and adapting but also a framework for verifying the reliability of new information.
Future Direction
- Research and Development
Ongoing research into better integration methods, including advanced machine learning algorithms and novel data structuring techniques, will be crucial. The goal is to make the integration of LLMs and KGs more efficient, scalable, and effective.
- Applications and Impact
Exploring new applications of this technology in various sectors, from healthcare to entertainment, will be key to understanding its full potential. As these AI systems become more sophisticated, they could significantly impact how we interact with information, making technology more accessible and useful for everyone.
- Ethical and Societal Considerations
As we advance, it is vital to engage with the ethical and societal implications of these technologies. Ensuring they are developed and used responsibly, with consideration for privacy, bias, and societal impact, will be crucial.
Conclusion
The unification of LLMs and KGs presents a thrilling frontier in AI development, promising AI systems that are not only more human-like in their interactions but also deeply grounded in factual knowledge. While challenges remain, the potential benefits of such integration ranging from enhanced text generation to personalized and accurate AI assistants are immense. As we continue to explore this constructive collaboration, the future of GenAI looks promising, offering new opportunities to enhance our interaction with technology in ways we have only just begun to imagine.
- Read more about Top Generative AI Use Cases and Applications
- Explore Graph Database Architecture and Use Cases