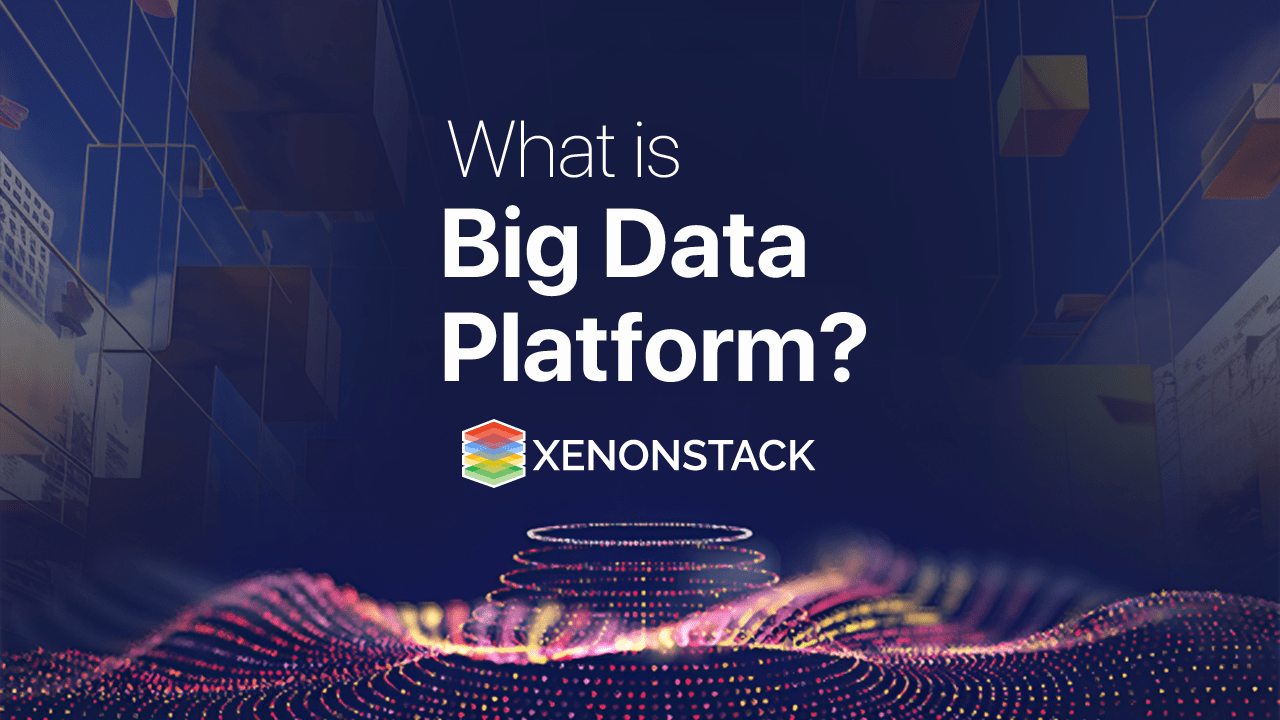
What is a Big Data Platform?
Big Data Platform provide the approach for data management that combines servers, Big Data Tools: Empowering Data Management and Analysis, and Analytical and Machine Learning into one Cloud Platform, for managing as well as Real-time Insights.
Big data Platform workflow is divided into the following stages
1. Data Collection
2. Data Storage
3. Data Processing
4. Data Analytics
5. Data Management and Warehousing
6. Data Catalog and Metadata Management
7. Data Observability
8. Data Intelligence
What is the need for a Big Data Platform?
This comprehensive solution consolidates the capabilities and features of multiple applications into a single, unified platform. It encompasses servers, storage, databases, management utilities, and business intelligence tools.
The primary focus of this platform is to provide users with efficient analytics tools specifically designed for handling massive datasets. Data engineers often utilize these platforms to aggregate, clean, and prepare data for insightful business analysis. Data scientists, on the other hand, leverage this platform to uncover valuable relationships and patterns within large datasets using advanced machine learning algorithms. Furthermore, users have the flexibility to build custom applications tailored to their specific use cases, such as calculating customer loyalty in the e-commerce industry, among countless other possibilities.
Different Types of Big Data Platforms and Tools?
This includes four letters: S, A, P, and S, which means Scalability, Availability, Performance, and Security. There are various tools responsible for managing hybrid data of IT systems. The list of platforms are listed below:
- Hadoop Delta Lake Migration Platform
- Data Catalog and Data Observability Platform
- Data Ingestion and Integration Platform
- Big Data and IoT Analytics Platform
- Data Discovery and Management Platform
- Cloud ETL Data Transformation Platform
Big Data Challenges include the best way of handling the large amount of data that involves the process of storing and analyzing the huge set of information on various data stores.
1. Hadoop - Delta Lake Migration Platform
It is an open-source software platform managed by Apache Software Foundation. It is used to collect and store large data sets cheaply and efficiently.
2. Big Data and IoT Analytics Platform
It provides a wide range of tools to work on; this functionality comes in handy while using it over the IoT case.
Know more about IoT Analytics Platform.
3. Data Ingestion and Integration Platform
This layer is the first step for the data from variable sources to start its journey. This means the data here is prioritized and categorized, making data flow smoothly in further layers in this process flow.
Get more information regarding data ingestion.
4. Data Mesh and Data Discovery Platform
5. Data Catalog and Data Observability Platform
It provides a single self-service environment to the users, helping them find, understand, and trust the data source. It also helps the users discover new data sources, if any. Seeing and understanding data sources are the initial steps for registering the births. Users search for the Data Catalog Tools and filter the appropriate results based on their needs. In Enterprises, Data Lake is needed for Business Intelligence, Data Scientists, and ETL Developers where the correct data is needed. The users use catalog discovery to find the data that fits their needs.
Get more guidance regarding the Data Catalog.
6. Cloud ETL Data Transformation Platform
This Platform can be used to build pipelines and even schedule the running of the same for data transformation.
Deep research on data transformation platforms using ETL.
What are the essential components of a Cloud Data Platform?
1. Data Ingestion, Integration and ETL – It provides these resources for effective data management and effective data warehousing, and this manages data as a valuable resource.
2. Stream Computing – Helps compute the streaming data used for real-time analytics.
3. Big Data Analytics Platform / Machine Learning – It Provides analytics tools and Machine learning Tools with MLOps and Features for advanced analytics and machine learning.
4. Data Integration and Warehouse – It provides its users with features like integrating it from any source with ease.
5. Data Governance – Data Governance also provides comprehensive security, data governance, and data protection solutions.
6. Provides Accurate Data – It delivers analytic tools, which help to omit any inaccurate data that has not been analyzed. This also allows the business to make the right decision using accurate information.
7. Cloud Datawarehouse for Scalability – It also helps scale the application to analyze all-time climbing data; it sizes to provide efficient analysis. It offers scalable storage capacity.
8. Data Discovery Platform for Price Optimization – Data analytics, with the help of a big data platform, provides insight for B2C and B2B enterprises, which helps businesses optimize the prices they charge accordingly.
9. Data Observability – With the warehouse set, analytics tools, and efficient Data transformation, it helps reduce the data latency and provide high throughput.
Conclusion
Building a Scalable Cloud Data Platform requires Defined Use cases for Real-time and batch Processing along with Data Strategy and Analytical Tools. According to Streaming or Operational analytics requirements, you can choose to manage, operate, develop, and deploy Cloud Data Platforms.
- Importance of Security Management and Platform Best Practices.
- Explain in detail about Big Data Use Cases.
- How to Accelerate Business Transformation with Data Warehouse Solutions?