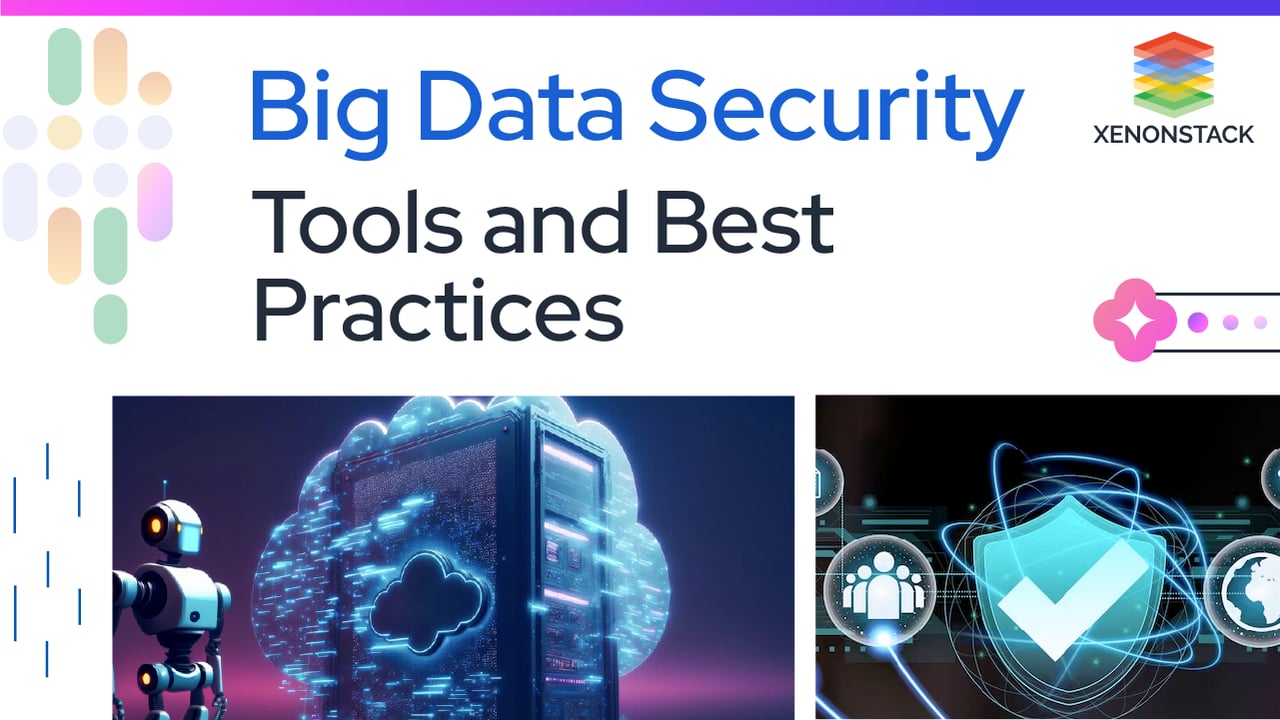
What are the significant challenges to securing Big Data?
Several big data security issues to protecting it can jeopardize its safety. It's important to note that these issues aren't limited to on-premise systems. They even have to do with the cloud. Take nothing for granted when hosting a big data platform in the cloud. With good security service level arrangements, the vendor needs to resolve these same obstacles.
Typical Challenges to Securing Big Data
-
Newer innovations in active growth include advanced computational methods for unstructured and non-relational databases (NoSQL). Protecting these new toolsets for security technologies and procedures can be complicated.
-
Data ingress and storage are well-protected with advanced encryption software. They may not have the same effect on data production from multiple analytics platforms to multiple locations.
-
Administrators of its systems can decide to mine data without obtaining permission or warning. Regardless of whether the motive is interest or criminal benefit, the monitoring software must track and warn about suspicious access.
-
The sheer scale of its installation, which can range from terabytes to petabytes, makes regular security audits impossible. Since most large data platforms are cluster-based, which exposes several nodes and servers to several vulnerabilities.
-
Its owner is at risk of data loss and disclosure if the environment's protection is not updated regularly.
-
Its protection professionals must be proficient in cleanup and know how to delete malware. Security software must detect and alert suspicious malware infections on the system, database, or web.
Top six challenges that come in the way while implementing Big Data. Click to explore about, Big Data Challenges and Solutions
Top 10 best practices for securing big data
The below listed are the top 10 Best Practices for securing it:
1. Safeguard Distributed Programming Frameworks
First, create trust using methods like Kerberos authentication and ensure predefined security policies are followed. The data is then "de-identified" by decoupling all personally identifiable information (PII) from it, ensuring that personal privacy is not jeopardized.
Then, using mandatory access control (MAC), such as the Sentry tool in Apache HBase, you allow access to files based on a predefined security policy and ensure that untrusted code does not leak information through device resources. After that, the hard part is done; all that is left is maintaining the system to prevent data leakage. In a cloud or virtual environment, the IT department should scan worker nodes and mappers for bogus nodes and altered duplicates of results.
2. Secure Non-Relational Data
Non-relational databases like NoSQL are common but vulnerable to NoSQL injection attacks by encrypting or hashing passwords and maintaining end-to-end encryption using algorithms such as advanced encryption standard (AES), RSA, or Safe Hash Algorithm 2.
With the advent of Big Data, the structured approach fails miserably to cater to the needs of the humongous information processing that tends to be unstructured in nature SQL vs NoSQL vs NewSQL: The Full Comparison
3. Secure Data Storage and Transaction Logs
Storage control is a critical component of its reliability. By using signed message digests to have a cryptographic identifier for each digital file or record and using a technique known as a secure untrusted data repository (SUNDR) to detect unauthorized file modifications by malicious server agents
4. Endpoint Filtering and Validation
Using a mobile device management solution, you can use trusted credentials, perform resource verification, and link only trusted devices to the network. Using statistical similarity detection and outlier detection strategies, you can process malicious inputs while defending against Sybil attacks (one person posing as several identities) and ID-spoofing attacks.
5. Real-Time Compliance and Security Monitoring
Using Big Data analytics, organizations can use techniques like Kerberos, Safe Shell, and internet protocol protection to get a grip on real-time data. It's then simple to monitor logs, set up front-end security mechanisms like routers and server-level firewalls, and start putting security controls in place at the cloud, network, and application levels.
Explore 10 Latest Trends in Big Data Analytics for 2025
6. Preserve Data Privacy
Employee awareness training centers on new privacy laws and ensures that information technology is kept up to date by using authorization processes. In addition, data leakage from different databases can be regulated by analyzing and tracking the infrastructure that connects the databases.
7. Big Data Cryptography
Mathematical cryptography has improved significantly. Enterprises can run Boolean queries on encrypted data by creating a method to scan and filter encrypted data, such as the searchable symmetric encryption (SSE) protocol.
8. Granular Access Control
The two main aspects of access management are limiting and allowing user access. The key is creating and executing a policy that automatically selects the best option in any situation.
Graph Databases uses graph architecture for semantic inquiry with nodes, edges, and properties to represent and store data. Role of Graph Databases in Big Data Analytics
To set up granular access controls:
-
Immutable elements should be denormalized, and mutable elements should be normalized.
-
Please keep track of confidentiality provisions to make sure they're followed.
-
Keep track of control marks.
-
Keep track of administrative information.
-
Use a single sign-on (SSO) and a labeling system to ensure proper data federation.
Strategies for Granular Access Control are listed below :
- Point out mutable elements and immutable elements.
- Access labels and admin data should be maintained.
- Use single sign-on and maintain a proper labeling scheme.
- Perform audit layer/orchestrator.
9. Granular Auditing
Granular auditing is essential for its protection, particularly after a system attack. Organizations should develop a unified audit view following an attack and include a complete audit trail with quick access to the data to reduce incident response time.
The integrity and security of audit records are also important. Audit data should be kept isolated from other data and safeguarded with granular user access controls and routine reporting. When configuring auditing, keep it and audit data separate and allow all necessary logging. An orchestrator tool like ElasticSearch can make it easier to do.
10. Data Provenance
It's provenance metadata that Big Data applications produce. This is a different kind of data that requires special protection. Creating an infrastructure authentication protocol that manages access, sets up daily status alerts, and constantly checks data integrity with checksums.
Hadoop File System (HDFS) is a distributed file system. All types of files can be stored in the Hadoop file system. Know more : Apache Hadoop 3.0 Features and Its Working
Essential Security Measures for Big Data Systems
It should meet four critical criteria – perimeter security and authentication framework, authorization and access, data privacy, and audit and reporting.
1. Authentication
Required to guard access to the system, its data, and services. Authentication makes sure the user is who he claims to be. Two levels of Authentication need to be in place – perimeter and intra-cluster - Knox, Kerberos.
2. Authorization
Required to manage access and control over data, resources, and services. Authorization can be enforced at varying levels of granularity and in compliance with existing enterprise security standards.
3. Centralized Administration and Audit
It is required to maintain and report activity on the system. Auditing is necessary for managing security compliance and other requirements like security forensics. - Ranger
4. Data at rest/in-motion Encryption
It is required to control unauthorized access to sensitive data while at rest or in motion. Data protection should be considered at the field, file, and network levels, and appropriate methods should be adopted for security - HDFS and wire encryption.
Big data security solutions for Enterprise
The use cases of Big Data Security are described below:
1. Cloud Security and Monitoring
The communication and data need to be secured on the cloud. It offers cloud application monitoring, security to host sensitive data and support for several relevant cloud platforms.
2. Insider Threat Detection
An insider threat can destroy a network. With the support of big data analytics, threats can be detected and avoided.
3. User Behaviour Analysis
Tracking and analyzing users' behavior can help monitor unusual behavior to detect suspicious patterns and thus prevent failure in the security of big data.
Know more about Big Data Compliance, Security, and Governance Solutions.
Effective big data security Solutions
1. Enabling Control and Agility
For businesses to secure sensitive data across their Big Data environments, including data sources, infrastructure, and analytics, the Data Security Platform provides data discovery and classification, granular access controls, strong encryption, tokenization, and centralized key management. Utilize big data analytics with the knowledge that all collected and mined data, including sensitive data, is secure.
2. Creating a Trusted Platform for Cryptographic Processing
It is crucial to protect sensitive data from compromise with big data encryption because handling exponentially growing volumes of sensitive data carries a high level of risk. Big Data security solutions use the tried-and-true data protection technologies of cryptography and key management to secure Big Data and establish trust for digital assets.