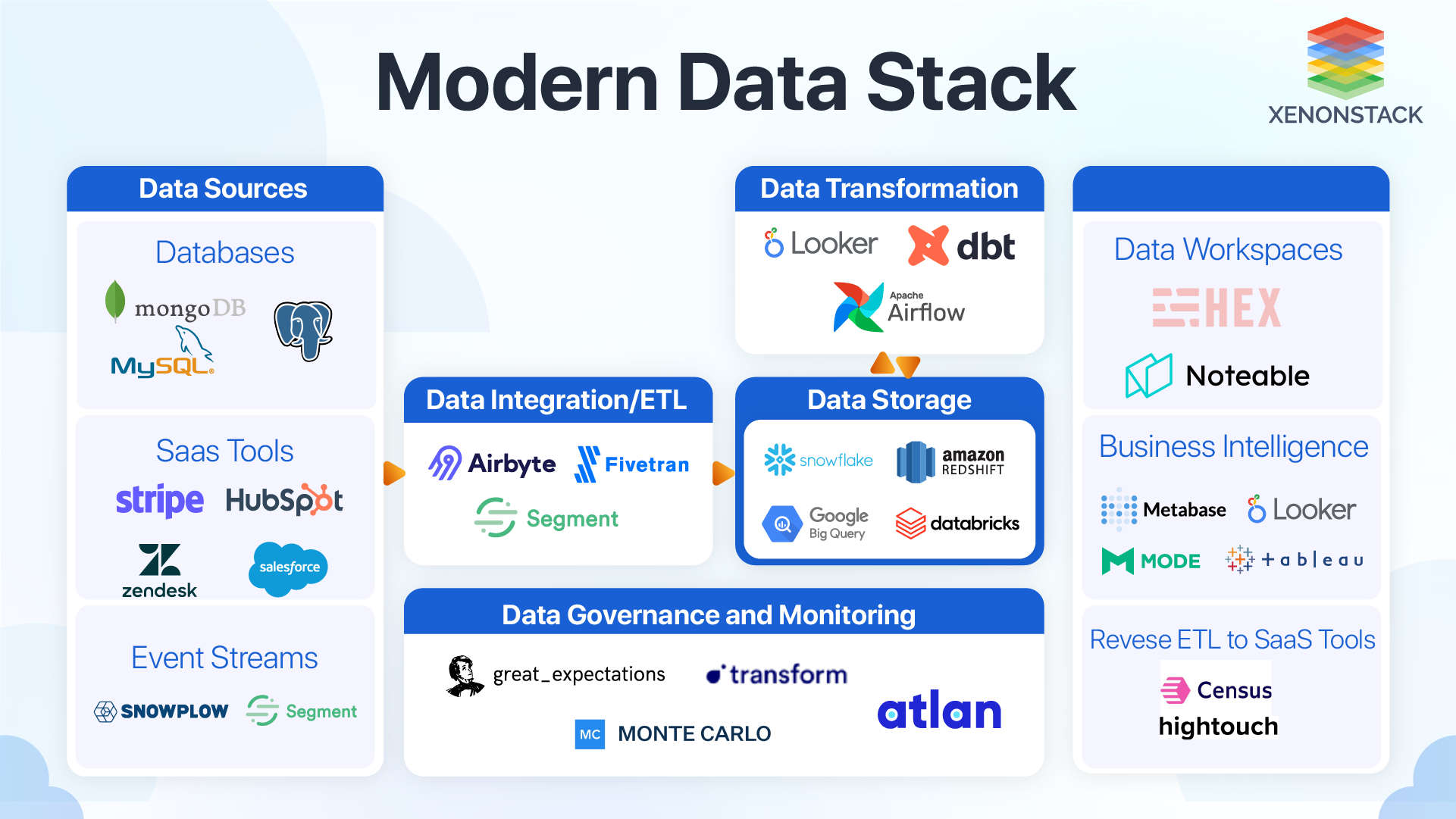
What is Modern Data Warehouse?
Modern Data warehouse comprised of multiple programs impervious to User. Polyglot persistence encourages the most suitable data storage technology based on data. This "best-fit engineering" aligns multi-structure data into data lakes and considers NoSQL solutions for JSON formats. Pursuing a polyglot persistence dat strategy benefits from virtualization and takes advantage of the different infrastructure.
Modern DW requires Petabytes of storage and more optimized techniques to run complex analytic queries. The traditional methods are relatively less efficient and not cost-effective to fit into the modern day Data Warehousing needs. There are tons of Cloud solutions to build data warehouses performance optimized, inexpensive, and support parallel query execution.
- Incorporate Hadoop, traditional data warehouse, and other data stores.
- Includes multiple repositories may reside in different locations.
- Include Data from mobile devices, sensors, cloud and the Internet of Things.
- Includes structure/semi-structured/unstructured, raw data.
- Inexpensive commodity hardware in cluster mode.
Data Warehousing is processing for gathering and handling data from various sources to provide essential business insights. Source: Data Warehouse Modernization
What is the working architecture of Modern Data Warehouse?
The working architecture of real-time Modern Data Warehouse is mentioned below:
Multiple Parallel Processing (MPP) Architectures
- MPP architecture enables a mighty scale and Distributed Computing.
- Resources add for a linear scale-out to the largest Data Warehousing projects.
- Multiple parallel processing architecture uses a "shared-nothing". There are numerous physical nodes, each runs its instance. This results from performance many times faster than traditional architectures.
Multi-Structured Data
- Define Big Data & Analytics Infrastructure for multiple storage data with a polyglot persistence strategy.
- Integrate portions of the data into the Data Warehouse.
- Federated query access.
Lambda Architecture
In lambda, architecture defines three layers -- Speed Layer - Low latency data.
- Batch Layer - Raw Data processing to support complex analysis.
- Serving Layer - Response to queries.
Hybrid Architecture
Scale up MPP compute nodes during -- Peak ETL data loads.
- High query volumes.
- Utilize existing On-Premises data structures.
- Use Cloud services for Advanced Analytics.
A mini Data Warehouse design that shows the contents to be needed only to the client-side, i.e. it holds the overview of the data. Click to explore about, Data Mart a Subset of The Data Warehouse
Why Modern Data Warehouse is important?
It solves the problems for various businesses such as:
- Data Lakes - Instead of storing in hierarchical files and folders, as traditional data warehouse do, a data lake is the repository that holds a vast amount of raw data in its native format until needed.
- Data Divided Across Organizations - Modern Data Warehousing allows for quicker information Assortment and Analysis across organizations and divisions. It keeps the Agility model and promotes more alignment and sooner effect.
- IoT Streaming Data - The Internet of Things has completely transformed the scenario, units, etc. share and stock data across multiple devices.
Business Challenges
- Reduce the cost to store and manage data growth.
- Business demand to analyze new data sources requires investment in technologies to process all data formats.
- Current Data Warehouses are good for Multidimensional Analytics but not suited for Image, Video or other new types of analytics.
The core process used to manage, centralize, and organize data according to business marketing and operations. Source: Master Data Management
How to adopt Modern Data Warehouse?
The steps to adopt it are described below:
Growing an Existing DW Environment
- Internal to the Data Warehouse
- Data modeling strategies
- Partitioning
- Clustered columnstore index
- In-memory structure
- MPP
Augment the Data Warehouse
- Complementary Data Storage & Analytical solutions.
- Cloud & Hybrid solutions.
- Data Virtualization/ Virtual DW.
What are the features of Modern Data Warehouse?
- Variety of subject areas & data sources for analysis with the capability to handle the large volume of data.
- Expansion beyond a single relational DW/Data Mart structure to include Data Lake.
- Logical design across multi-platform architecture balancing performance & scalability.
- Data virtualization in addition to Data Integration.
- Support for all type & levels of users.
- Flexible deployment decoupled from the tool used for development.
- Governance model to support security and trust, and Master Data Management.
- Support for promoting the self-service solution to the corporate environment.
- Ability to facilitate Real-Time analysis of high-velocity data.
- Support for Advanced Analytics.
- Agile Delivery approach with the fast delivery cycle.
- Hybrid Integration with Cloud services.
- APIs for downstream access to data.
- Some DW automation to improve speed, consistency, business terminology.
- An analytics sandbox or workbench area to facilitate agility within a BI environment.
- Support for self-service BI to augment corporate BI; Data discovery, Data Exploration, Self-service Data preparation.
The Concept of Database designing is key, whereas the SQL queries part is relatively very simple. Click to explore about our, Data Warehouse Database Design Architecture
What are the best practices of Data Warehousing?
Below highlighted are the best practises of it:
Define the Compression Formats and Data Storage
There can be more than one option for data storage. Each storage option offers distinct advantages and benefits. It is necessary to evaluate the data formats and storage to work smoothly with the applications in an ecosystem.
Look out for Multi-tenancy Support
Multi-tenancy support is important for the BI environment. It gives the advantage of using a single software stack to serve thousand of partners & customers and make upgrades or customization.
Review the Schema
Evaluate the nature of the database storage. Verify how it’s loaded, processes, and analyzed to optimize schema objects.
Ensure Metadata Management
Ensure end-to-end Metadata Management for Data Warehouse initiatives Metadata Management defines. Metadata Management establishes the success of Modern Data Warehousing projects. It captures the necessary information to build, use and interpret the Data Warehouse elements.
What are the benefits of Modern Data Warehouse?
- Rapid integration of data into the environment.
- Improved efficiency in integration reducing time, cost and efforts.
- Opportunity to enable innovative new data models.
- Potential for new insights into the data that provide Preventive analysis and Predictive Analysis.
- Ability to have more extensive datasets for analysis as the data collected and stored continues to grow exponentially.
- Cost advantages of Open source software & Commodity hardware.
Conclusion
The opportunities of Big Data and Advanced analytics are a big challenge. The most sophisticated are changing to meet the requirements of the Modern Data Enterprise. Increase in volume expected to continue. Business velocity continues to change business operations and customer interactions. Data becomes even more diverse and more available than ever before. Big Data means a big impact on business. To dig into the immense new opportunities of Big Data, the Modern enterprise needs a modern data platform. Microsoft solution delivers platform, solutions, features, functionality, and benefits that empower the Modern Enterprise in three essential areas i.e easily manage relational and non-relational data at all volumes and high performance, enjoy a consistent experience across on-premises and Cloud, gain insights from BI and Advanced Analytics across all data wherever it resides. Y
- Explore our insight based on Container Storage Interface for Kubernetes
- Learn more about Container Management and its Platforms