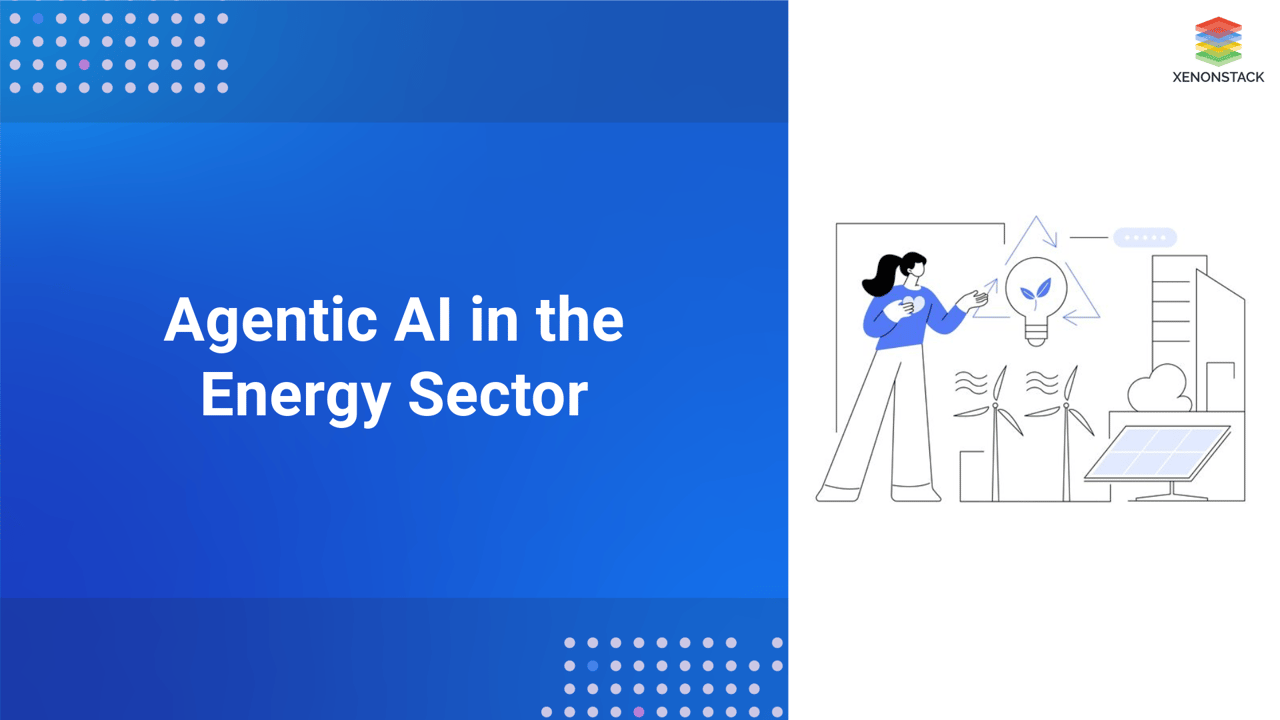
The Dawn of Autonomous Energy Systems
The energy sector is at the cusp of a revolution. With the rise of decentralized grids, fluctuating renewable energy sources, and the increasing complexity of global energy demands, traditional AI-driven automation is no longer enough. What’s needed is a new paradigm—Agentic AI—where autonomous, self-learning AI agents independently perceive, decide, and act to optimize energy production, distribution, and consumption without human intervention.
Unlike traditional AI, which requires predefined rules and human oversight, Agentic AI continuously learns from its environment, interacts dynamically, and executes actions to achieve optimal energy efficiency, cost savings, and sustainability goals.
How Agentic AI is Transforming the Energy Sector
Autonomous Grid Management & Self-Healing Energy Networks
The modern power grid is no longer a simple distribution network; it has become an intricate web of interconnected energy sources, consumers, and regulatory constraints. Agentic AI is revolutionizing smart grids by:
-
Detecting and preventing failures: AI agents autonomously monitor grid health, anticipate outages, and redirect power flow before disruptions occur.
-
Self-optimizing energy distribution: AI dynamically balances load demand between conventional and renewable energy sources for maximum efficiency.
-
Real-time response to external factors: From cyber threats to extreme weather conditions, Agentic AI adapts energy distribution in response to live environmental data.
Intelligent Energy Market Participation & Decentralized Trading
Energy markets are rapidly shifting toward decentralized trading, where consumers and producers engage in real-time energy transactions. Agentic AI powers this transformation by:
-
Automating energy trades in milliseconds: AI agents execute buy/sell decisions autonomously, ensuring optimal prices and reducing market volatility.
-
Facilitating peer-to-peer (P2P) energy transactions: Households and businesses with solar panels and battery storage can trade surplus energy directly, enabled by AI-driven smart contracts.
-
Predicting price fluctuations: AI agents analyze vast datasets of weather patterns, grid load, and geopolitical factors to forecast market trends with precision.
Dynamic Renewable Energy Integration & Climate Adaptation
As renewable energy sources become dominant, their unpredictability presents a major challenge. Agentic AI tackles this by:
-
Intelligent forecasting: AI predicts energy production from solar, wind, and hydropower using live satellite data, atmospheric conditions, and historical trends.
-
Autonomous storage management: AI optimizes battery storage systems, deciding when to store energy and when to release it based on supply-demand fluctuations.
-
Grid-balancing through real-time demand response: AI agents incentivize consumers to shift electricity use to off-peak hours through automated energy pricing adjustments.
Fully Automated Predictive Maintenance & Asset Health Management
The costliest failures in the energy industry arise from unexpected breakdowns. Traditional maintenance relies on periodic inspections, leading to inefficiencies and failures. Agentic AI revolutionizes maintenance by:
-
Self-diagnosing potential failures before they occur: AI agents continuously analyze sensor data from wind turbines, power plants, and distribution lines to detect early warning signs of malfunctions.
-
Autonomous decision-making for repairs: AI schedules and prioritizes maintenance tasks without human input, ensuring minimum downtime and maximum asset longevity.
-
Reducing operational costs: Energy companies can cut expenses significantly by eliminating unnecessary servicing and responding only when AI detects genuine risks.
AI-Powered Energy Storage & Battery Optimization
With the rise of renewable energy, efficient energy storage is essential. Agentic AI enhances energy storage by:
-
Optimizing battery charging and discharging cycles: AI predicts demand fluctuations and adjusts storage usage accordingly.
-
Extending battery lifespan: AI-driven analytics prevent overuse and degradation of energy storage systems.
-
Enhancing grid stability: AI helps synchronize energy storage with grid operations to ensure a continuous power supply.
AI-Driven Cybersecurity in Energy Infrastructure
As energy systems become increasingly digital, cybersecurity threats are a growing concern. Agentic AI strengthens security by:
-
Detecting anomalies in real time: AI identifies unauthorized access, unusual power fluctuations, and potential cyber threats before they escalate.
-
Automating incident response: AI agents can isolate affected areas and deploy countermeasures without human intervention.
-
Enhancing compliance and risk management: AI ensures regulatory compliance by monitoring cybersecurity protocols continuously.
Human-AI Collaboration in Energy Decision-Making
While Agentic AI operates autonomously, human oversight remains crucial. AI-driven decision-making enhances human expertise by:
-
Providing real-time insights: AI-powered dashboards assist energy operators with critical decision-making.
-
Improving regulatory compliance: AI helps policymakers design and implement energy regulations more effectively.
-
Enabling adaptive learning: AI learns from human feedback, ensuring continuous improvement and alignment with industry goals.
Incorporating Software Development with Agentic AI offers the energy sector tailored solutions that evolve continuously, driving smarter grid management and predictive analytics.
Emerging Trends in Agentic AI for Energy
The energy sector is evolving rapidly, and several key trends are driving the adoption of Agentic AI:
Decentralization & Microgrids
AI-powered energy systems are shifting from centralized control to localized, autonomous microgrids.
Blockchain Integration
Smart contracts and AI-driven transactions are enabling secure and transparent energy trading.
AI-Powered Carbon Management
AI is optimizing carbon capture, usage, and storage (CCUS) to reduce environmental impact.
Hybrid AI & IoT Solutions
Combining AI with IoT sensors is enhancing real-time monitoring and predictive analytics in energy systems.
Benefits of Agentic AI in the Energy Sector
Agentic AI offers numerous advantages that make it indispensable for the future of energy:
Efficiency & Cost Reduction: AI-driven automation minimizes energy waste and lowers operational expenses. Sustainability & Decarbonization: AI optimizes the integration of renewable energy, reducing dependency on fossil fuels. Resilience & Reliability: Self-healing grids powered by AI improve energy system stability. Market Competitiveness: Companies adopting Agentic AI gain a competitive edge in dynamic energy markets.
Challenges in Adopting Agentic AI in Energy
Despite its potential, deploying Agentic AI comes with challenges:
Regulatory and Ethical Constraints
AI-driven energy decisions must comply with evolving regulations and ethical frameworks.
Cybersecurity Risks
As AI takes control of critical energy infrastructure, safeguarding against cyberattacks is paramount.
High Initial Investment
The integration of self-learning AI agents into existing energy grids demands significant financial and technological investment.
Public Perception & Trust
The idea of autonomous AI making high-stakes energy decisions needs clear communication and trust-building with stakeholders.
The Future of Energy: A Fully Autonomous Ecosystem
Agentic AI is not just optimizing the energy sector—it is redefining it. With self-learning AI agents managing grid stability, automating energy markets, and predicting failures before they happen, the future of energy is one of autonomy, resilience, and sustainability.
As AI technologies evolve, energy companies that adopt Agentic AI early will lead the transition toward an intelligent, carbon-neutral, and decentralized energy future. Investing today means securing a sustainable and cost-efficient tomorrow.
Next Steps in Implementing Agentic AI Systems
Talk to our experts about implementing a Compound AI System. See how industries and the energy sector use Agentic AI workflows and Decision Intelligence to become Decision-Centric. Leverage AI to automate and optimize energy operations, improving efficiency and responsiveness.