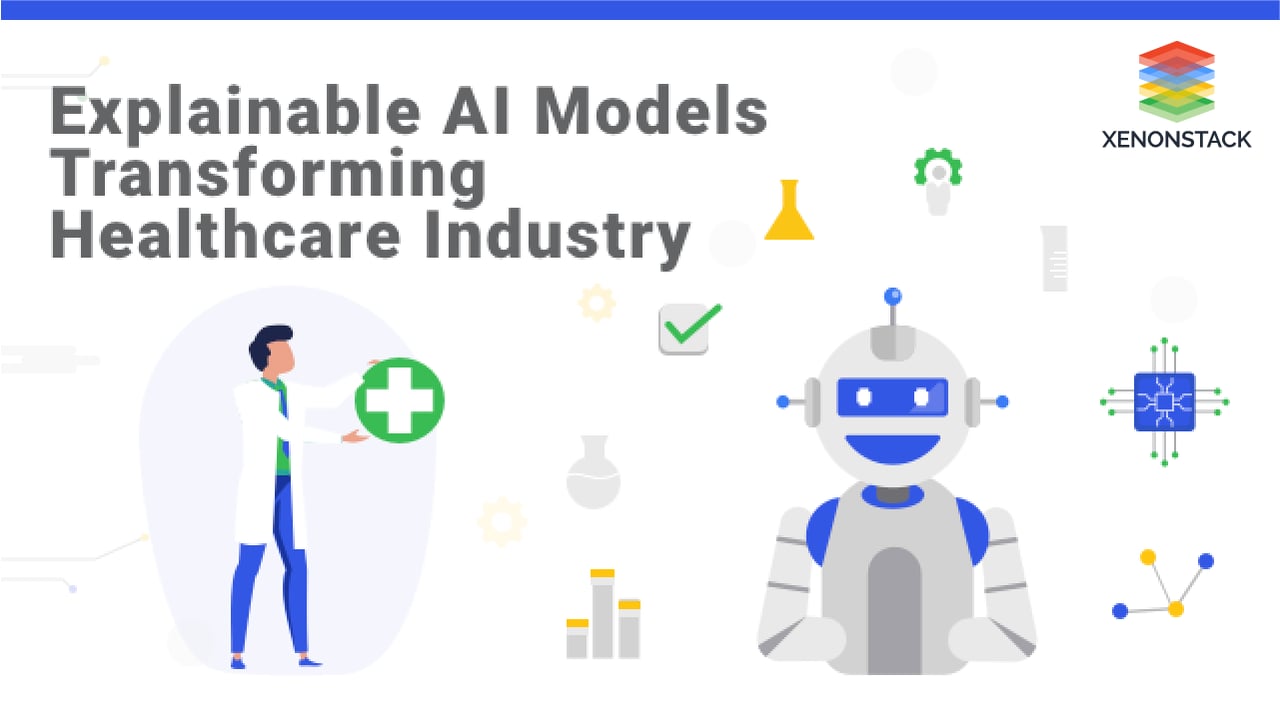
Introduction to AI in Healthcare
Artificial Intelligence (AI) offers various opportunities for the Health industry. AI makes better and quicker decisions in health care. Big data and AI systems can make decisions for the diagnosis that humans cannot. Such as detecting atrial fibrillation from an electrocardiogram.
AI for health is divided into subtopics:
Perceptual AI: Perceptual AI perceives the disease
Intervention AI: Intervention AI decides how the patient should be treated according to their diseases.
But most of the health industry's AI systems function as a black box. It means systems are opaque; they are not explaining the cause of their output. Therefore, users cannot understand how a system reaches a particular result. This results in a lack of trust and confidence in these systems. They are not adopting that system, especially where an explanation is crucial. For this, Explainable AI came into existence. It explains the model. It provides the reason behind each prediction of the model. It explains which data is contributing, why it is selected, and its works.
Generative AI has many potential uses in healthcare, including drug discovery, disease diagnosis, patient care, medical imaging, and medical research. Generative AI in Healthcare
When do we need an Explanable AI?
Not all AI systems need to explain. There are some cases where only we require an explanation. It is necessary to know which system requires an explanation before making that system. So that developers would be able to use a suitable strategy. The following are some points listed down from where we can get to know when our system must explain.
- When Fairness is Critical: The system should explain the systems where fairness is mandatory, and people cannot compromise with fairness.
Predictions are crucial and have an essential and widely effective impact on far-reaching consequences. Such as recommending an operation, recommending sending a patient to hospice, etc.
When the cost of a mistake is high, the system's explanation is mandatory where. The system must provide the correct result. And if it is mispredicted, it can cost a high or a life. For example, the misclassification of malignant tumors can be dangerous for a person. - When performance is critical: When a system or model's performance is critical.
When compliance is required, the system should explain when it is mandatory for privacy concerns of data or a right—for example, GDPR (General Data Protection Regulation). - When trust is necessary: When required to gain the user's trust and confidence, the system should explain how it reaches a particular output. It should tell the feature, parameters, and model that it uses.
Why do we need Explainable AI in Healthcare?
Healthcare is an industry in which some use cases demand an explanation. For many fields except healthcare, AI systems' black box functioning is acceptable. Sometimes, users want their system does not to reveal the logic as it is desirable to keep their logic secret and private. But in the case of Health care, where mistakes can give dangerous results, black box functioning is not acceptable to doctors and patients. As we know, doctors are well-trained to identify diseases and provide treatment. Suppose our AI system is not trained properly on correct data, then how can it diagnose patients? Therefore it isn't easy to trust the system as users cannot be sure of its result. We have to use Explainable AI to overcome machine learning's opaque nature to support Explainable AI's basic principles, such as transparency, fairness, etc.
Let's discuss a case to understand better why we need Explainable AI: An AI system that can detect cancer in Caucasian skin rather than dark-skinned people. It misses potentially malignant lesions on dark skin. So they recognize that this system is providing a biased output. The result of this misjudged output can be dangerous here for the life of some subpopulations. After that, it is noticed that this bias is coming due to insufficient data. The data used to train the system does not contain much about dark skin. Therefore our system needs more transparency and explanation about its result, data, and prediction model.
RPA will revolutionize the healthcare industry, saving time, energy, and costs, reducing paperwork and working efficiency, and ultimately improving patient care. Robotics in Healthcare Management
What are the Principles of Explainable AI in Healthcare?
Implementing Explainable AI in our AI system should obey four Explainable AI principles in healthcare. Listed below are the following principles:
Explanation
The explanation is essential in healthcare, where its consequences can bet on a person's life. According to this principle, the system will provide a reason for each decision. The system predicts the likelihood of admitting patients to the hospital's emergency department. In explanation, the system will focus on the three major questions as these three factors are generating the output.
- What algorithm is being used?
- How does the model work?
- Which inputs or parameters of the data are taking part in determining an output?
These questions help to understand whether the system is working correctly or not. So they can make decisions about whether they will use that system or not. They can also give the reason for the output. Our example system predicts that Malin(patient) has a low likelihood of 28% to be admitted to the hospital. The system predicts that from his age, signs, and medical history. The system also explains the reason for its prediction using visualization.
Meaningful
The explanation that the system provides is significant. It will be understandable to the targeted user. The system provides different explanations for different groups of users. It will be different for the end-user and the developers according to their prior knowledge and experience. If a user can understand the information, it means it is meaningful. The explanation that the system provides should be understandable to the targeted recipient. For example, Figure 1.2 represents a linear model. It is difficult for a recipient to understand model B's features who don't know models and statistics. Because model two explains some statistical variables, this explanation will be meaningless. But if this explanation is for developers, they can understand it and become meaningful.
We can explain the algorithm and model to the developer to understand how the model and algorithm work because they can understand them. But for patients, we can provide the data and parameters that are used and give the output.
Explanation Accuracy
This principle states that explanations should be accurate. Our system explains the same procedure the AI system used to generate output. Because if it generates the wrong output, it can harm a patient's life, significantly when the system predicts a chronic disease in which it is compulsory to take immediate action. This can also be possible because patients know that generating is wrong and losing customers' confidence. For example, there is a system that predicts whether a patient has cancer or not. If the system gives false explanations, then It can be possible that the system predicts correctly that patients don't have cancer, but its explanation shows a chance of cancer. The explanation may be wrong, but it can lose the customer's confidence. Therefore we should use the correct tool and the correct way to represent the system's explanation.
Knowledge Limits
Knowledge limits prevent the system from giving an unjust and fallacious result. Hence users can assure that the system will never mislead. It will simply show the outcome that will be set for the system. For example, it may be possible that the system will get a different feature or scenario rather than giving false results about which system doesn't know our system will provide that these inputs are out of the topic. For example, a system is typically for predicting skin cancer. But by mistake, a user provides different parameters, such as predicting diabetes. Here rather than putting the patient's life in danger by giving the wrong result, the system will say that the input is totally out of the topic and wrong.
Handling this system can be designed as if the system encounters a different situation and can generate results out of topic.
AI in Healthcare can raise ethical issues and can harm patients by not giving intended outcomes. Ethical AI in Healthcare and its Principles
How Explainable AI reduces challenges?
The main challenge in an AI system is customer trust. Opaque AI systems provide the system's output without reason or Explanation. Therefore, It becomes challenging for the customer to trust the machine that does not explain, especially in the healthcare system. Various questions come to the customer's mind that the opaque system cannot answer, as Figure 1.1 shows. Due to this incompetence of opaque AI systems, they are not adopted by patients and medical practitioners.
Explainable AI reduces these challenges.
-
Trust and confidence: it becomes tough to gain trust and confidence in doctors and patients due to the AI system's opaque nature. Users look for explanations of the system for various reasons, such as to learn and understand model logic and to provide working of the system with others to give the reason for making decisions. Explainable AI builds users' trust and confidence by providing them with explanations.
-
Detect and Remove Bias: Users cannot recognize the system's defect and bias because it does not provide transparency. Hence it becomes difficult to detect and remove bias and provide safeguards against bias.
-
Model Performance: Due to less awareness of model users not being able to track the model's behavior.
-
Regulatory Standards: Users are not able to recognize whether the system is obeying the regulatory standards or not. Because, Otherwise, this can harm the system.
-
Risk and Vulnerability: Explainability of how systems tackle risks is very important. Especially in situations where the user cannot be sure of the environment. Explainable AI helps to detect it timely and take action on it. But if the system does not explain how the user can mitigate these risks?
What are the Benefits of Explainable AI in Healthcare?
As a result of Explainable AI, AI systems are rapidly adopted by healthcare. Because AI systems recognize patterns and make decisions based on Big Data, it is difficult for a human to make decisions. Explainable AI is providing us with the following features in our system:
- Transparency: Transparency is the foremost principle of Explainable AI. It is the algorithm, model, and features understandable by the user. Different users may require transparency in different things. It provides a suitable explanation for suitable users.
- Fidelity: The system provides a correct explanation. It should match the model performance.
- Domain sense: The system provides an explanation that is easy to understand for the user and makes sense in the domain. It is explained in the correct context.
- Consistency: Explanation should be consistent for all predictions because different explanations can confuse the user.
- Generalizability: The system should provide a general explanation. But it should not be too general.
- Parsimony: The explanation of the system should not be complex. It should be as simple as possible.
- Reasonable: It accomplishes the reason behind each AI system's outcome.
- Traceable: Explainable AI can track logic and data. Users get to know the contribution of data in the output. The user can track problems in logic or data and solve them.
AI can accelerate and advance the medical research, prevention, diagnosis, and treatment of diseases. Taken From Article, Responsible AI in Healthcare Industry
Solution by XenonStack
To overcome the challenge, XenonStack explains its opaque AI systems. It answers the questions that arise in the customer's mind while using the AI system. It makes an AI system more reliable and productive by providing trust, transparency, and fidelity. To answer all these questions, XenonStack uses Explainable AI.
Conclusion
As already discussed, the most AI system is not answerable for their result, which can sometimes harm society or the user by providing wrong results. Explainable AI and its principle bring a change in the system's traditional functioning and explain the algorithm, model, and features it uses. With transparency, AI systems can become fair and flawless in the Health industry.
- Read here about Artificial Intelligence in Diabetes Detection
- Explore here about Data Analytics in Healthcare Industry