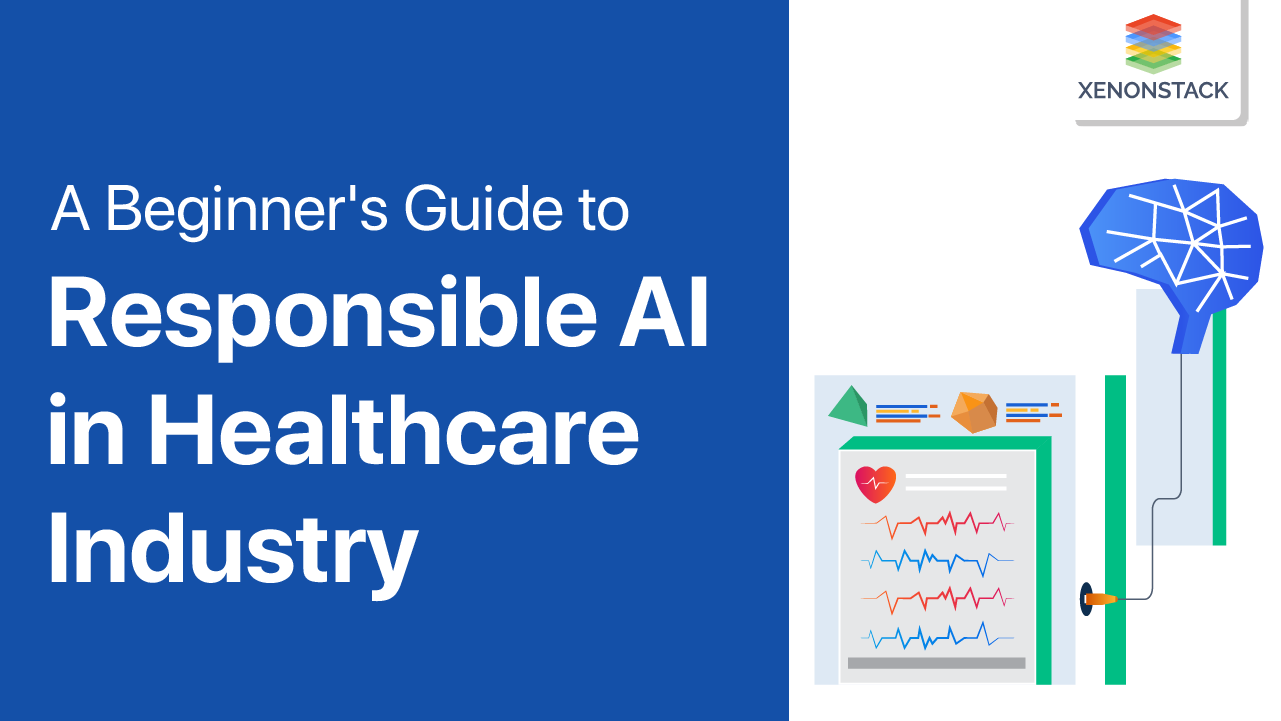
Introduction to Responsible AI
Experts have raised concerns about AI ethics and transparency with raising failure of artificial intelligence applications. Currently, the black box implementation of it cannot detect issues. The truth is that it is a very crucial industry, so these ethical challenges must be identified and mitigated since artificial intelligence has the tremendous capability to threaten patient privacy, safety, and preference. Such issues hinder its adoption in the healthcare industry. The issue must be resolved because any action and decision taken in the industry is a matter of life and death for a person. Several questions are raised before the adoption of artificial intelligence in healthcare:
BI is the set of processes, architectures, and technologies that transform raw data into meaningful information that drives beneficial business action. Click to explore about our, Business Intelligence in Healthcare Industry
- In the industry, it is a matter of life, death, and the well-being of individuals, so to what extent can we rely on artificial intelligence algorithms?
- How do we safeguard that its algorithms are only used for intended purposes?
- How do we ensure that AI does not discriminate against specific cultures, communities, or other groups?
Responsible AI in healthcare
Although, it creates business values and benefits but also raises a host of unwanted and severe consequences. For example, privacy violations, discrimination, inequalities, proliferation, etc.
Artificial Intelligence applications require careful management to prevent unintentional but significant damage to society. It can solve this issue and build confidence between humans and computers by justifying how they reach particular solutions.
What are the common challenges?
The complexity of algorithms increases to deliver better outcomes. Thus understanding these algorithms is also becoming challenging due to their complexity. It raises reliability issues in healthcare. This process can’t work for the industry because knowing how and why artificial intelligence gives these results is very important. Below has listed some of the challenges of AI in healthcare:
Diagnostic Error
- Diagnostic error is 60% of all healthcare errors. This error could give a huge amount of wrong diagnostic approaches.
- Undoubtedly, it can offer more accurate diagnostics, but there is always a chance of making mistakes. Thus companies hesitate to adopt artificial intelligence in diagnosis.
- The primary reason for these errors is poor quality and biased data.
Quality Data collection
ML model needs fair and reasonable data quality to make accurate predictions, but finding high-quality, bias-free data is a significant challenge in the healthcare industry. Biased data might perpetuate inequalities.
Privacy and Security
Data privacy and security is a prominent issue. As patient data may contain some sensitive details, such as personally identifiable information, according to the Health Insurance Portability and Accountability Act (HIPA) and GDPR (General Data Protection Regulation), this type of data needs to be protected. Concerns about data privacy, security, and leakage reduce the adoption of artificial intelligence. For instance, the University of Washington has accidentally shared data of almost 1 million people due to database configuration errors.
Data Ingesting, use, and preprocessing have become increasingly difficult as the amount of unstructured data is being ingested from various sources. Hence, it’s easy to fall prey to pitfalls such as inadvertently using or revealing sensitive information hidden among anonymized data.
Healthcare industries must use automation as there are so many paper-based processes or time-consuming processes that can be automated. Click to explore about our, RPA in Healthcare
What are the approaches for Responsible AI in healthcare?
The best approaches are described in the following paragraphs:
Empower leadership
A chief artificial intelligence ethics officer should be appointed as a leader. That will be responsible to convenes stakeholders, identifying champions across the organisation, and establishing principles. They also guide the team in the creation of AI systems.
Develop principles, policies, and training
Responsible artificial intelligence principles are base of it. They are an integral part of it. Spending time on principles, policies, and training is very important. The team should have a clear idea of principles.
Human + AI governance
Defining an ethical framework, roles, responsibilities, procedures, and policies are equally important to build a successful, Responsible artificial intelligence application. It should have a highly effective governing system to review things and bridge gaps.
Conduct Reviews
A process should be built to conduct an end-to-end review. Effective integration depends on regularly assessing the risks and biases associated with the outcomes of each use case. Its practitioners can sport risks early and flag them to resolve them as soon as possible.
Integrate tools and methods
For a productive and impactful system evolving and integrating tools are very important. Such as conductive review manually is time-consuming and complex. But if there is a tool to perform that task, it could simplify the process.
Build and test a response plan
In addition to the Responsible AI procedure, there should be a proper plan to handle if any lapse occurs. It should be tested well. Policies and procedures need to be developed, validated, tested, and refined to ensure that harmful consequences are minimized to the greatest extent possible if an artificial intelligence system fails.
A framework to help technologists while designing, developing, or maintaining systems. Click to explore about our, Ethical AI in Healthcare and its Principles
What are its benefits in the industry?
The benefits of responsible artificial intelligence are described below:
Minimize unintended bias
It helps to mitigate the bias from data and algorithms to ensure and build Bias free and responsible AI applications.
AI Transparency
Explainable artificial intelligence interprets the working of an algorithm which helps to understand it better than how an algorithm makes decisions.
Ensure Privacy
Protect the privacy and security of data using Privacy and Preserving AI.
It keeps sensitive information secure from malicious attacks.
It makes sure that sensitive data never be used unethically.
Conclusion
AI can accelerate and advance the medical research, prevention, diagnosis, and treatment of diseases. But humans must govern it to mitigate bias and embed explainability. It will make artificial intelligence trustworthy and responsible for its actions. Healthcare Industry is already using AI and ML in powerful ways, but they must be applied judiciously.
- Discover here about Explainable Artificial Intelligence
- Click to explore about Edge AI vs Federated Learning