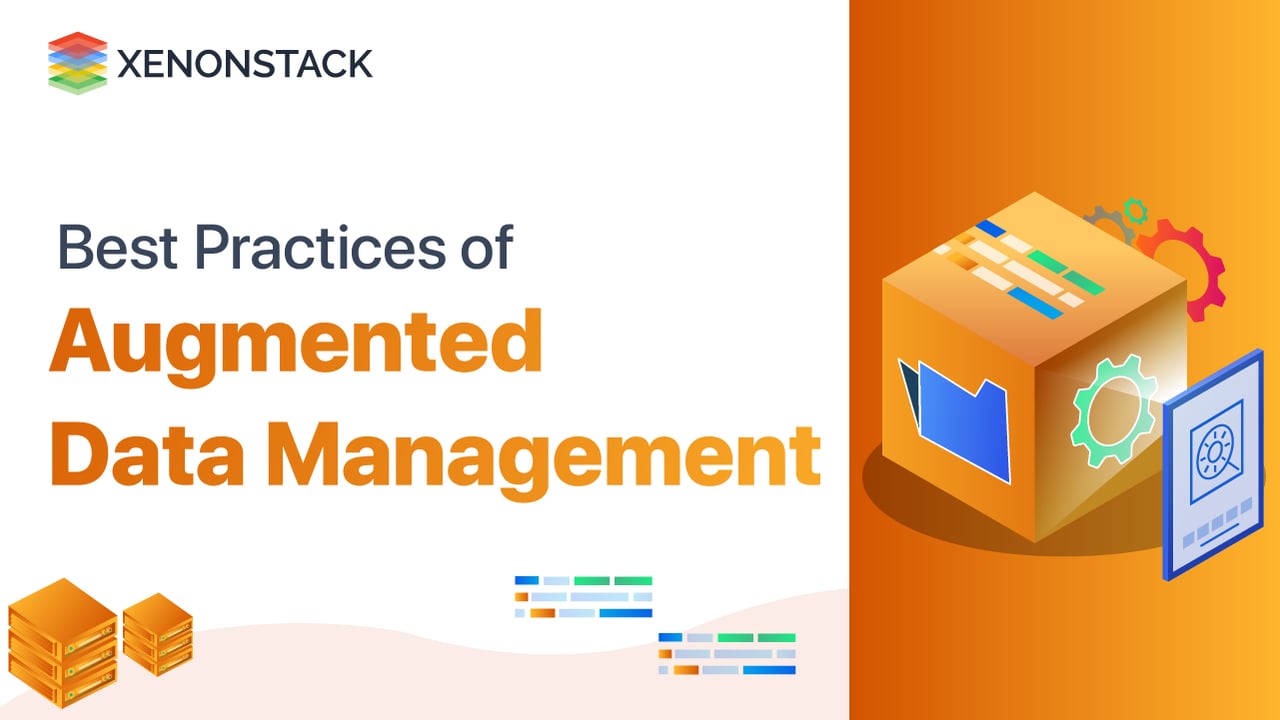
What Is Augmented Data Management?
Augmented Data Management applies artificial intelligence (AI) to improve or automate data management processes. It automates many Data Management tasks previously performed manually, allowing less technically knowledgeable individuals to be more autonomous in their data usage.
Why do we need Augmented Data?
Augmented data is a slight change in existing data, so we don't need to get massive data from various sources and store that data. We need to modify the existing data a little bit, and that works well for training and testing in machine learning, deep learning, and ANN.
Problems with Traditional Data Management
Data management is the process of gathering, processing, storing, and safeguarding data. Traditional solutions may fall short of meeting the problems of data management when the volume of data grows because:
-
The conventional method organizes and maintains data in a classic file environment, storing the same data across several systems, databases, and files. It can result in data duplication. Inconsistencies in data might occur if data is not controlled centrally.
-
The control, organization, and protection of data can become out of hand as the volume of data grows.
-
Traditional dataset backups are time-consuming since they need manual copying, tagging, and re-filtering.
-
Due to the inability of certain traditional data management technologies to preserve data lineages and relationships, data is kept in silos.
What is Augmented Data Management?
Artificial intelligence (AI) to improve or automate data management processes is known as augmented data management. It may help data talent with manual work, such as time-consuming and data-intensive jobs. Finding anomalies in massive datasets, addressing data quality concerns, and tracking detailed data from a report back to its source are just a few examples. AI models are more complex and intended to do these data management duties, taking less time, making fewer errors, and saving money in the long term.
Best Practices of Augmented Data Management
The best practices of Augmented Data Management are mentioned below:
Data Quality
Instead of relying just on statistical profiling to ensure data quality, sophisticated analytics approaches can speed up the Process. Following are some examples of ensuring augmented data quality.
Time-series forecasting and predictive categorization
To get a complete and consistent view of your business, fill in the gaps in your data and enhance it. Time series forecasting methods Include Moving Average, Vector Autoregression, Vector Autoregression Moving-Average, Autoregression, Autoregressive Moving Average, Autoregressive Integrated Moving Average, Simple Exponential Smoothing, etc.
Check for Uniqueness:
SQL rules can automate and look for distinct values. Once these filters are set, they can be scheduled to run automatically in intervals.
Check for Completeness:
Rules can be set that look for null values to see whether any important information is missing.
Ensure Conformity: The term "conformity" refers to data that is in the correct format or Structure. SQL and machine learning can be paired together to ensure the Conformity of data.
Data integration:
Traditional data management technologies are primarily concerned with replicating and relocating data. Augmented data integration combines data from several sources to acquire a unified perspective of your data. This approach can provide more precise recommendations, allowing the team to integrate new data sources easily. They can rest indeed and will not be jeopardized the dataset's quality in any manner.
The main features to consider in augmented data integration tools are: Combined different data destinations, Versatile Data Access, Active metadata analysis, Process at speed, and API support.
Data Fabric
We're aware that data may be accessible in various formats and from a variety of locations around the world, whether on-premise or on the cloud. Unfortunately, the data collected becomes increasingly segregated and inaccessible as more programs are involved in the Process. Creating a data fabric gives businesses a unified picture of all their data and a single environment for accessing, aggregating, and analyzing it. Without requiring an entire army of tools, data fabric helps reduce silos and enhances data input, quality, and governance.
Some examples of data fabrics to consider for enhancing your organization's processes are: Enhancing Machine learning models, building a holistic customer view, Keeping the organization's network traffic safe from the public internet, Managing keys and secrets safely across the cloud.
Master Data Management
By building a master data record, Master Data Management delivers a one-stop shop for business-critical information to everyone in an organization. It gathers information from several departments' systems to build a master record for all departments. By discovering and analyzing master data, Augmented MDM provides a master data model, a single source of truth for multiple data sources.
The most common example of master data is customer records, whereas it may also include product data, asset data, employee data, transactions, and analytical data. Augmented MDM enhances the consistency and quality of these core data assets.
Metadata Management
Data labeling, classification, and search. Datasets are used to generate the metadata model and metadata rules. Technical and business metadata is automatically collected, organized, cataloged, and merged for structured and unstructured data—identifying system relationships, data flow, and anomalies by generating and evaluating end-to-end data lineage.
Database management solutions
With database-as-a-service solutions, automatic patching updates, better data security, data access, automated backups, disaster recovery, and scalability are all possible. Users can access and use a cloud-based database system without the organization needing to buy and install its hardware or database software or manage the database in-house.
Data mesh enables decentralised data operations, which improves time-to-market, scalability, and business domain agility.Click to explore Adopt or not to Adopt Data Mesh? - A Crucial Question
Benefits of Augmented Data Management
The benefits of Augmented Data Management are listed below:
Faster Data Preparation
Data scientists and engineers spent around 80% of their time manually preparing data. AI/ML automation is used in Augmented Data Preparation to bring data from several sources together much more quickly.
Improved Data Literacy
As businesses continue to acquire vast amounts of data, it isn't effortless that everyone can benefit from it, regardless of their analytical talents. Automatically surfacing and explaining findings democratizing AI across the data value chain improves data literacy.
Reduced Analytical Bias
Analysts make assumptions when they don't know where to start looking for answers. Those assumptions can often lead to using specific evidence to back them up. By automating analysis across an extensive dataset of statistical significance, augmented data management can help eliminate potential bias.
Reduced Time to Insights
ML techniques for discovering correlations, segments, clusters, outliers, and relationships are automatically applied to the data with augmented analytics, rather than an analyst manually analyzing all the combinations of variables in the data.
A data strategy is frequently thought of as a technical exercise, but a modern and comprehensive data strategy is a plan that defines people, processes, and technology.Discover here 7 Key Elements of Data Strategy
Understanding the business impact of Augmented Data Management
You can protect high-quality data for real-time analytics and make quick business choices using augmented data management. Using machine learning and artificial intelligence to make data management processes self-configuring and self-tuning allows the company to move away from traditional data management and analytics. In a traditional data analytics process, the business relies on the data team to scout the correct data, clean it, model it, analyze it, and generate insights by traversing the organization hierarchy. Using augmented data management, companies can harness data through cross-departmental communication, numerous complete tasks, and make proactive decisions within their departments
Breaking down data silos and realizing the value of data faster helps break down data silos, lowering corporate operating expenses. The ability of augmented data management to convert metadata so that may use it in auditing, lineage, and reporting to dynamic power systems is an added benefit. Large samples of operational data, including actual queries, performance statistics, and schemas, can be analyzed using ADM solutions.
What is the future of Augmented Data Management?
The Augmented data market is expected to grow between $70bn-$75bn by 2023. We can see the future growth of augmented in the graph.
Conclusion
Organizations are constantly in need of revamping their data management operations to automate their data circulation processes and improve their data analysis. Organizations can improve their products and services by removing data complexity if they have the necessary tools for successful data management. Any firm needs to aim for and achieve its business objectives to reduce complications. The safest path forward is using augmented data management methods to stay competitive and give your competitors a run for their money.
- Explore more about Augmented Data Management Best Practices
- Click to explore Augmented Data Management Solutions
- Know more about Data Management Services and Solutions