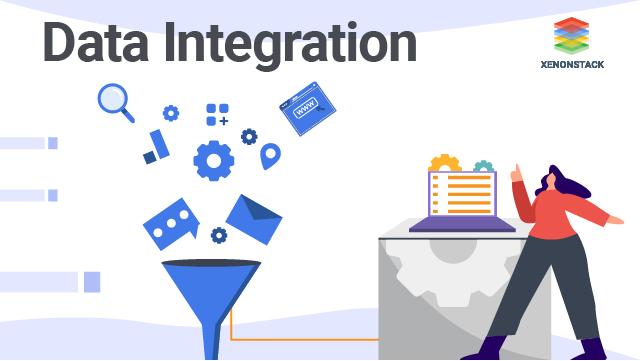
Introduction to Data Integration
It is collecting and combining data from various resources. It provides a unified structure or view of the combined data to manipulate operations, perform analytics, and build statistics. Integration is the initial step towards transforming data into more descriptive and critical data. There are mainly, two types:
Enterprise Data Integration (EDI)
It is technological instructions that help us to manipulate data over two or more data sets. As the name suggests, it typically involves acquiring data from diverse business systems and crunching them to perform various management activities and business intelligence reports.
Customer Data Integration (CDI)
For a business organization to be successful, its main motive must be satisfying customers, understanding their needs and preferences. With the humongous amount of data already available, it is pretty obvious to assume that there is marginal difficulty accessing and operating on the data at a much faster pace., So CDI is nothing but the process of collecting and manipulating customer data among numerous multiple sources and framing data in a unified way so that it would be easy to share among every member of that business organization that deals with customers. Predictive Insight, Improved Customer Service, Loyal Customers are some of the benefits to name under CDI.
Why Data Integration is important ?
With the increasing volume of data collected through various sources and at a much faster velocity every day, it is very much clear that Data is and has been the most valuable possession. The businesses are very keen on implementing various strategies to utilize the data to complete applications as possible. Still, the real question is how efficiently that can be done. So, let's understand what it means- The problem with such an immense quantity of Data is also quite extensive. According to a survey conducted online by Experian, thewhir.com, and others, nearly 60% of companies today lack a properly functioning business strategy, resulting in catastrophic effects. It tends to solve this issue quite effectively by doing a real-time view and analysis of the Data, thus collecting various targets.
-
Helps in reducing Complexity in Data
-
Increases the value of data crunched through unified systems
-
Centralizing the data, i.e., making it more valuable and easy to use
-
Collaborations make easier among various business systems.
-
Make Smarter Business decisions.
-
Improves the communication between different departments under the hood
-
Secures your data live by keeping information timely up-to-date
-
Better customer experience
Best tools for Data Integration
No doubt, the demand for data integration arises from complex data center environments where various multiple systems are creating large volumes of data. One must understand the Data in accumulation rather than in isolation. It is nothing more than a technique and technology for providing a unified and consistent view of enterprise-wide data. There are numerous tools available in the market that would help us query the Data effectively since our data will not integrate itself. To name a few, we have some Open Source, Cloud-based, and On-premises tools. The best tool to choose depends on the requirements, platform, and data type that particular business organizations are likely to use.
According to recent search results, some of the best data integration tools for 2023 include:
- Hevo Data
- Dell Boomi
- Informatica PowerCenter
- Talend
- Pentaho
- Informatica Cloud
- MuleSoft Anypoint Platform
- Oracle Data Integrator (ODI)
- IBM InfoSphere DataStage
- Fivetran
What are the challenges to implementing Data Integration tools?
Implementing data integration tools may pose several challenges, including:
1. Varied Data Formats and Sources: Businesses collect data from diverse applications and sources, leading to inconsistent formats and structures.2. Data Availability: Ensuring timely access to data where needed can be challenging.
3. Data Quality Concerns: Inconsistent definitions, lack of validation mechanisms, and poor data cleansing practices hinder quality improvements.
4. Escalating Data Volumes: Managing extensive daily data generation complicates integration, demanding more resources.
5. Diverse Data Sources: Integrating structured and unstructured data from multiple origins complicates the process.
6. Hybrid Environments: Integrating data across cloud-based and on-premises systems adds complexity.
7. Consistency Issues: Maintaining data consistency across various formats and sources is challenging.
8. Complex Implementation: Data integration tool implementation requires meticulous planning, mapping, and cleansing for accuracy and reliability.
Benefits of Data Integration
The whole data management system has a nucleus cover called data integration. It is essential to carry out any expected result. If any system goes through the discussed methodologies, they are expected to taste numerous fruitful benefits.
The advantages of data integration are vast and impactful, contributing to enhanced business operations and decision-making. These benefits encompass:
1. Enhanced Data Quality: Integrating data ensures consistency and accuracy, elevating its reliability for analysis and informed decisions.
2. Cost Efficiency: By automating tasks and refining processes, data integration reduces operational expenses and minimizes manual data handling.
3. Improved Decision-Making and Collaboration: Integrated data offers a comprehensive business view, fostering informed decisions and interdepartmental collaboration.
4. Operational Efficiency: Streamlined data access and processing via integration enhance operational productivity.
5. Enhanced Customer Experiences: Integrated data provides insights into customer needs, leading to better experiences and tailored services.
6. Revenue Opportunities: Unveiling new opportunities and market insights drives new revenue streams and business expansion.
7. Data Accessibility and Security: Integrated data offers improved accessibility for analysis and reporting, along with centralized management for enhanced security.
What are the use cases of Data Migration ?The use cases of it are defined below:
1. Data Mining
We operate on the existing data from the database and try to bring out all the necessary information from that raw data, it is Data Mining. The pre-processor to fetch data from multiple distributed sources is called Data Integration. Then these are stored in a structured manner in the database and using that database. There are two approaches for it, namely Tight Coupling - In this, the data warehouse is assumed as an interface that retrieves information using the ETL(Extract, Transform, and Load) operations from multiple targets into a single centralized location. Loose Coupling - An predefined interface is provided that manipulates and transforms queries so that the root storage can understand and ensure no temporary storage is done. Everything acts in the source database only.
2. Data Warehousing
It is one of the significant aspects of Data Warehousing. At the highest level, if we talk about Data Warehousing, it is nothing but the innovation, manipulation, and mapping practices to match the correct set of requested data with the data to be forwarded as a response to the end-user. ETL(Extract, Transform and Load) is a significant data integration component in data warehousing. The most well-known implementation of data warehousing is building a data warehouse for the enterprise side. The data warehouse is all about internal operations. But the constraint is that all the integration operations and management are completely external to the organization. To bring them as a collective unit without any redundancy, we can use it as a local-as-a-view approach. Each table in the database is used as a globally defined source to a corporate view.
3. Business Intelligence(BI)
Business Intelligence is the set of operations done to bring out useful information from the raw data available. BI facilitates data integration by centralizing, contextualizing, and enhancing the quality of data, streamlining the data gathering process, and ultimately improving decision-making within organizations. Additionally, it supports developing better communication to collaborate effectively and support decision-making pointers for better outcomes. First, collect and integrate the data with the data warehouse, where it goes under various manipulations. The valuable data obtained is held under multiple BI tools to support the data analysis. Consider BI Tools as Decision support systems (DSS) tools as they allow the business members to make analyses and extract useful information. Sometimes it gets complicated as one really would feel that everything is the same, and there's no key difference among the impact of it in mining, warehouse, and business intelligence. The critical link among these is that for everything to work out efficiently, the top priority is it.
Conclusion of Data Integration tools
From business processes to analytics, warehouses, and anything that is either way directly or indirectly dependant on Data, is nothing without data integration. So, organizations should have complete knowledge and access to every Source to grow as a collective unit.
- Discover more about Data Preparation Process
- Read here about Real Time Big Data Integration Solutions