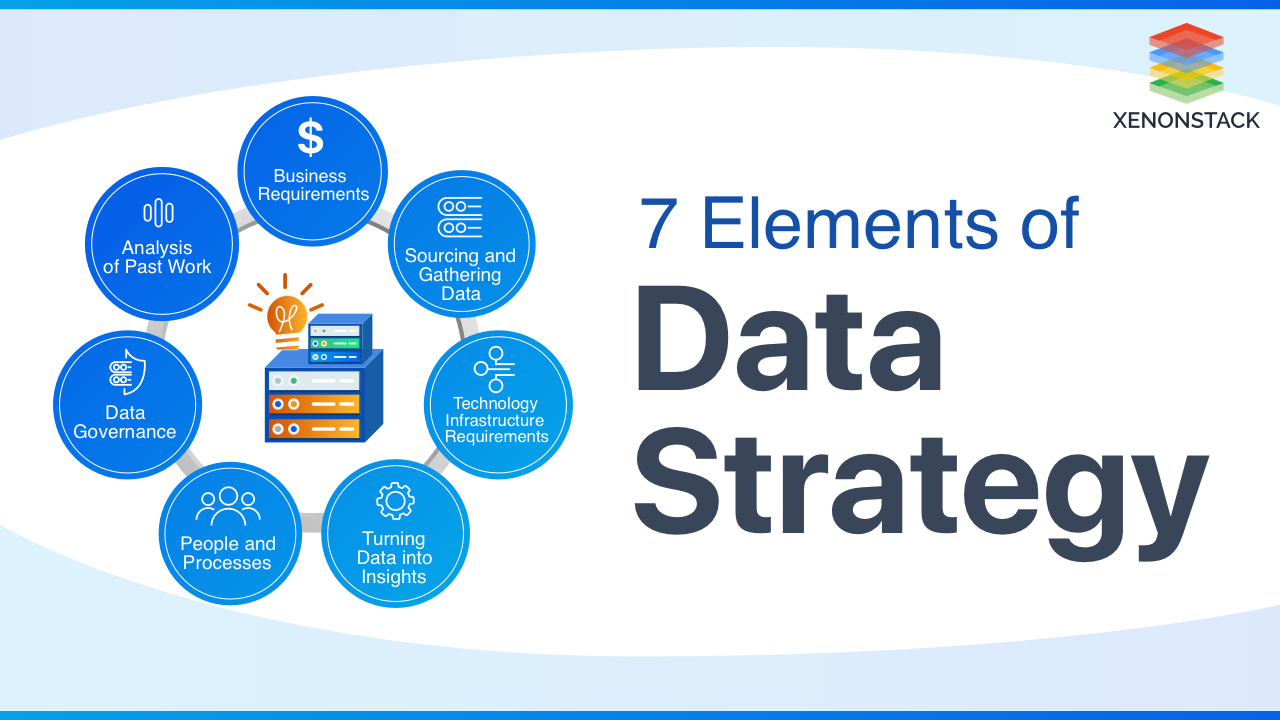
Introduction
According to Gartner, 87 % of firms have low BI and analytics maturity, with only 17 % of BI solutions deployed across a company. Data is a strategic asset that requires the correct business intelligence technology and the right processes and strategy to realize its full potential. While appointing a chief data officer is a good start, it should be part of a larger data strategy. A strategic action plan loaded with realistic targets for data teams and users can result in accurate and timely data-driven decision-making.
Data Strategy is possible to move toward a more data-driven culture, and it begins with a data strategy. All of your data practices are built on the foundation of a data strategy. It's not a band-aid solution for your data issues. It's a long-term strategy that identifies the people, processes, and technology you'll need to address your data issues and achieve your business objectives.
What is Data Strategy?
A data strategy is frequently thought of as a technical exercise, but a modern and comprehensive data strategy is a plan that defines people, processes, and technology. The process of developing a data strategy requires business leaders to consider:
- What employees require to be empowered to use data.
- Processes that ensure data is accessible and of high quality.
- Technology that will enable the storage, sharing, and analysis of data.
Why do we need Data Strategy?
Having a solid data strategy that allows businesses to make the most of their data and business intelligence investments by achieving meaningful business benefits and identifying new revenue opportunities.
It assists business executives in laying the foundation for data-driven businesses and fostering a data-driven culture. In fact, according to a PWC study, highly data-driven firms were 3x more likely than those who weren't to claim advances in their data decision-making. A data strategy enables businesses to do things like:
- Recognize the kind of data being generated and how to store it effectively.
- Create a framework for analyzing the correct data and gaining valuable insights.
- Select the appropriate business intelligence and analytics software.
- Enhance decision-making to drive corporate development and value.
Key Elements of Data Strategy
The below mentioned are the 7 elements of data strategy:
What are the Business Requirements for Data Strategy?
Data ought to deal with particular enterprise wishes, an excellent way to attain strategic dreams and generate actual value. The first step of defining the enterprise's necessities is to pick out a champion, all stakeholders, and SMEs inside the enterprise. The champion of the information approach is the govt chief who will rally aid for the investment. Stakeholders and different SMEs will constitute particular departments or features in the enterprise.
Next is to outline the strategic dreams and tie branch sports to enterprise dreams. It’s herbal for dreams to exist on the enterprise and branch degree. However, the said dreams for each degree ought to sync up. These targets are successfully collected through an interview that begins off evolving on the govt degree and keeps right down to branch’s leaders. Through this manner, we’ll find out what leaders are looking to measure, what they're looking to improve, questions they need to be answered, and ultimately, the KPIs to reply to the one's questions.
Data observability establishes the state of the organization's data and, for the most part, ends data outages by bringing DevOps observability best practises to data pipelines.Read more about How Data Observability Drives Data Analytics Platform?
How to Source and Gather Data for Data Strategy?
With awesome expertise of what questions the commercial enterprise is asking, we will flip to the following elements: reading sources, gathering facts, and wherein the facts exist. It’s not likely that each fact might be to be had inside the enterprise and that it already exists in an area that’s accessible. So, we want to paintings backward to discover the supply.
For facts that may be discovered in-house, we are aware of the supply device and any roadblocks to gaining access to that facts. We also want to decide whether or not the facts have the proper stage of element and are up to date with the appropriate frequency to reply to the query effectively. For example, is the facts private (mainly in mild of GDPR and CCPA)? Is it guarded via means of regulations added on via way of means of software program license?
We’ll be aware of facts that aren’t to be had right here and choose it up withinside the subsequent step. For example, a retail employer also needs to understand how an emblem is perceived earlier than and after a huge product launch. Let’s say the store has name middle facts, site visitors count for shops and online, and normal income and go back numbers. That doesn’t degree how clients are feeling or what they’re pronouncing approximately the emblem. The store can also additionally pick to tug in social media facts to degree sentiment. Call middle facts, site visitors, and income might be stated on this step, together with the possibility to tug in social media facts.
Data governance that is effective protects against misuse and maintains data consistency and reliability.Explore more about Big Data Governance Tools, Benefits, and Best Practices
What are the technology infrastructure requirements for Data Strategy?
Our first piece of recommendation is: Don’t get stuck up within the hype and today's technologies; pay attention to the enterprise motives in your initiatives. Building a bendy and scalable facts structure is a complicated subject matter for which there are numerous alternatives and approaches, so right here are a few vital matters to consider:
- To what volume can an operational device help analytics needs? Likely very little. It usually’s now no longer a high-quality exercise to depend on an operational device to satisfy analytical needs. Because of this that a critical facts repository could be useful.
- Does the enterprise have the talents and technical infrastructureto help a facts warehouse on-prem, or could leveraging a cloud-primarily based answer make greater sense?
- Where the facts don’t exist today, how will the gaps be filled? Can the facts be calculated or estimated? Can it be bought from third-celebration marketplace fashion facts or macro-monetary facts? Or can a brand new supply device be carried out to generate the facts?
- Is there an intelligent integration device to get the facts from supply structures into the critical repository? Will this deposit of the structure be leveraged for enterprise good judgment so that the facts are prepared to be used?
- How will you offer or provision get entry to the facts? Will IT create reviews, or will you permit self-service? Will the reviews be pixel-perfect (printable) reviews, or will the reviews permit a person to interplay with the facts? Will they be embedded into websites and furnished to human beings out of doors the network?
Turning Data into Insights
Data visualization gear must make the information appearance good, however extra importantly, it makes the information less difficult to apprehend and interpret. Some elements that must be taken into consideration while deciding on an information visualization device include:
- Visualizations: You must be capable of fast spot traits and outliers and keep away from introducing confusion through an awful presentation.
- Story Telling: The dashboard must gift the context of metrics and expect the person's course of research and diagnosis.
- Democratization of Data: Who has to get entry to what information? Encourage sharing and wide-unfold adoption and outline not unusual place definitions and metrics throughout the organization.
- Data Granularity: Be capable of offering the proper degree of element for the proper audience. An analyst can also additionally want extra precise statistics than an executive, and a few humans can also additionally want drill-down capabilities.
A data pipeline is a technique for transferring raw data from various data sources to a data store.Click to explore What is a Data Pipeline?
Data Governance
In the end, data governance lets the organization share facts and the oil that lubricates the equipment of an analytics practice. A facts governance application will make certain that:
- Calculations used throughout the organization are decided primarily based totally on entering from throughout the organization.
- The proper humans have got the right to entry to the proper facts.
- Data lineage (in which the facts originate and the way changed into it converted, seeing that that origination) is defined.
People and Processes
When it involves a system, many agencies have unintended roadblocks to using their information in choice-making. Business techniques might also additionally want to be re-engineered to comprise information analysis. This may be done by documenting the stairs in a system wherein unique reviews are leveraged for a choice. We also can mandate that unique information be furnished as intent for an enterprise choice. Recognition also can pass a protracted way—whilst you advantage a win, this is primarily based totally on a new use of information. It has to be celebrated and promoted to construct inner momentum and inspire high-quality behaviors with information.
A data catalogue is a collection of metadata, along with data management and search tools, that aids analysts and other data users in finding the data they require.Click to explore about Data Catalog with Data Discovery
Analysis of past work for Data Strategy
The facts method roadmap results from all of the paintings we’ve performed thus far and makes all our preceding paintings actionable. We’ve diagnosed all that wishes to convey you from where you're to in which you’d want to go; however, earlier than getting beginning out with any design, build, training, or re-engineering of an enterprise process, it’s vital to prioritize the sports. For every piece of advice to assist in bridging the distance from the contemporary nation to the destiny nation, outline the feasibility and predicted enterprise cost it'll offer. The plan must prioritize sports that can be simplest to enforce; however, it offers brief wins to the enterprise.
Other elements to consist of withinside the facts method roadmap are:
- Staff availability and whether or not door assistance is required.
- A company’s budgeting process, particularly if capital funding is needed.
- Competing initiatives that could save you the proper assets from participating.
Conclusion
Emerging technologies may allow a new generation of data-management skills, possibly making defensive and offensive plans easier to deploy.
Only a few back-office activities, such as payroll and accounting, used to rely on data. It is now essential to any business, and the necessity of strategically managing it is only increasing. The consequences are unavoidable: companies who have not yet developed a data strategy and a solid data management function must either catch up quickly or prepare to exit.
- Discover more about Top 9 Challenges of Big Data Architecture
- Read more about Graph Database: Working | Advantages | Use-Cases