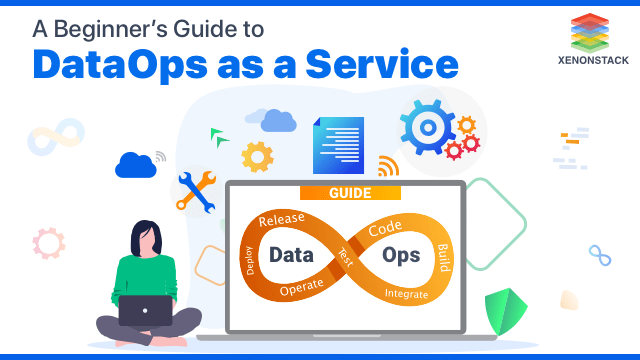
Introducing DataOps as a Service
In the ever-shifting era of technologies where a new term emerges and evolves each day, data being generated is also increasing. Businesses are investing in data science and ML operations to get value out of this data. Sadly, every business cannot get what it aims for and cannot call itself truly data-driven. In reports, it shows more than fifty percent of data science projects never make it to production. All POC remains at your local system driving you away from the real value of data. Often the Data Science projects are manual processes handcrafted by highly trained professionals. They are like wizardry that would not scale automatically. It is like manufacturing plants run with automation that pushes you into the pitfall of tribal behaviors. These behaviors, in turn, will yield poor quality results, ever going life cycle, scalability issues, malformed data, and hogs time. Every Problem has a solution to it. To this, it is DataOps as a Service.What is DataOps
DataOps is to data what DevOps is to development. DataOps is a discipline that provides agile and process-oriented methodologies for developing and delivering data insights and analytics. Inspired by DevOps, DataOps seeks to bring streamlined changes in data analytics. What started as just a set of best practices has evolved into an independent approach to the analytics platform. It is applied to the whole life cycle from data ingestion to processing to governance to cataloging to quality assurance.DataOps Methodology is a new and independent view approach to data analytics based on the whole data life cycle. Source: Implementing DataOps Architecture in AWS, Azure, and GCP
What DataOps as a service Offers?
DataOps as a Service is offered as a combination of a multi-cloud big-data/data-analytics management platform and managed services around harnessing and processing the data. It provides scalable, purpose-built big data platforms that adhere to best practices in data privacy, security, and governance using DataOps components. Data Operations (DataOps) as a service means providing real-time data insights. It reduces the cycle time of data science applications and enables better communication and collaboration between teams and team members. Increasing transparency by using data analytics to predict all possible scenarios is necessary. Processes here are built to be reproducible and reuse code whenever possible and to ensure higher data quality. This all leads to the creation of a unified, interoperable data hub.Enable Deeper collaborations
As business knows what data represents, it thus becomes crucial to have collaboration between It and business. It enables the business to automate the process model operations. Additionally adds values to the pipelines by establishing KPIs for the data value chain corresponding to the data pipelines. Thus enabling businesses to form better strategies for their trained models. DataOps bring the organization together in different dimensions. It helps bring localized and centralized development together as a large amount of data analytics development occurs in different corners of enterprise that are close to business using self-service tools like Excel. The local teams engaged in distributed analytics creation play a vital role in bringing creative innovations to users, but as said earlier, lack of control pushes you down towards the pitfall of tribal behaviors; thus centralizing this development under it enables standardized metrics, better data quality, and ensures proper monitoring. Too much rigidness chokes creativity, but with Dataops, it is easy to move into and for motion between centralized and decentralized development; hence, any concept can be scaled more robustly and efficiently.Read more about Data Management Best Practices with DataOps.
Set up Enterprise-level DevOps Ability
Most organizations have completed or are iterating over the process of building Agile and DevOps capabilities. Data Analytics teams should join hands and leverage the enterprise’s Agile and DevOps capabilities to:- The transit from a project-centric approach to a product-centric approach (i.e., geared toward analytical outcomes).
- Establish the Orchestration (from idea to operationalization) pipeline for analytics.
- automated process for Test-Driven Development
- Enable benchmarks and quality controls at every stage of the data value chain.
Automation and Infra Stack
One of the primary services that DataOps provide is to scale your infrastructure in an agile and flexible manner to meet ever-changing requirements at scale. The integration of commercial and open-source tools and hosting environments enables the enterprise to automate the process and scale Data & Analytics platform services. For example, it provides infrastructure automation for- Data warehouses
- BI platforms
- Data lakes
- Data catalogs
- Machine learning infrastructure
Orchestrate Multi-layered Data Architecture
Modern-day data platforms are complex with different needs, so it’s essential to align your data platform with business objectives to support vast data processing and consumption needs. One of the proven design patterns is to set up multi-layered architecture (raw, enriched, reporting, analytics, sandbox, etc.), with each layer having its value and meaning and serving a different purpose and increasing the value over time. It is also essential to register data assets across various stages to support and bring out data values by enabling enterprise data discovery initiatives. Enhance and maintain data quality at different layers to build assurance and trust. Protect and secure the data with security standards so providers and consumers can safely access data and insights. Scale services across various engagements and reusable servicesBuilding end to end Architecture
Workflow orchestration plays a vital role in binding together the data flow from one layer to another. It helps in the automation and operationalization of the flow. Leverage the modularized capabilities to Key pipelines supported by DataOps are:- Data Engineering pipelines for batch and real-time data
- Common services such as data quality and data catalog pipeline
- Machine Learning pipelines for both batch and real-time data
- Monitoring reports and dashboards for both real-time data and batch data
Click to explore Amundsen Lyft – The New Revelation in DataOps
What are the Benefits of DataOps as a Service?
Listed below are the benefits of DataOps as a service.- Simplify complex data analytics orchestrations and operations.
- Automated process and attain more value from them.
- DataOps connects organizations in two ways from development to operations and from localized development to centralized development.
- Reduce the life cycle of data processing, cleaning, and loading.
- Increases the value of data by increasing the quality of data.
- Reduce time and cost of data by automating the redundant and reusable process.