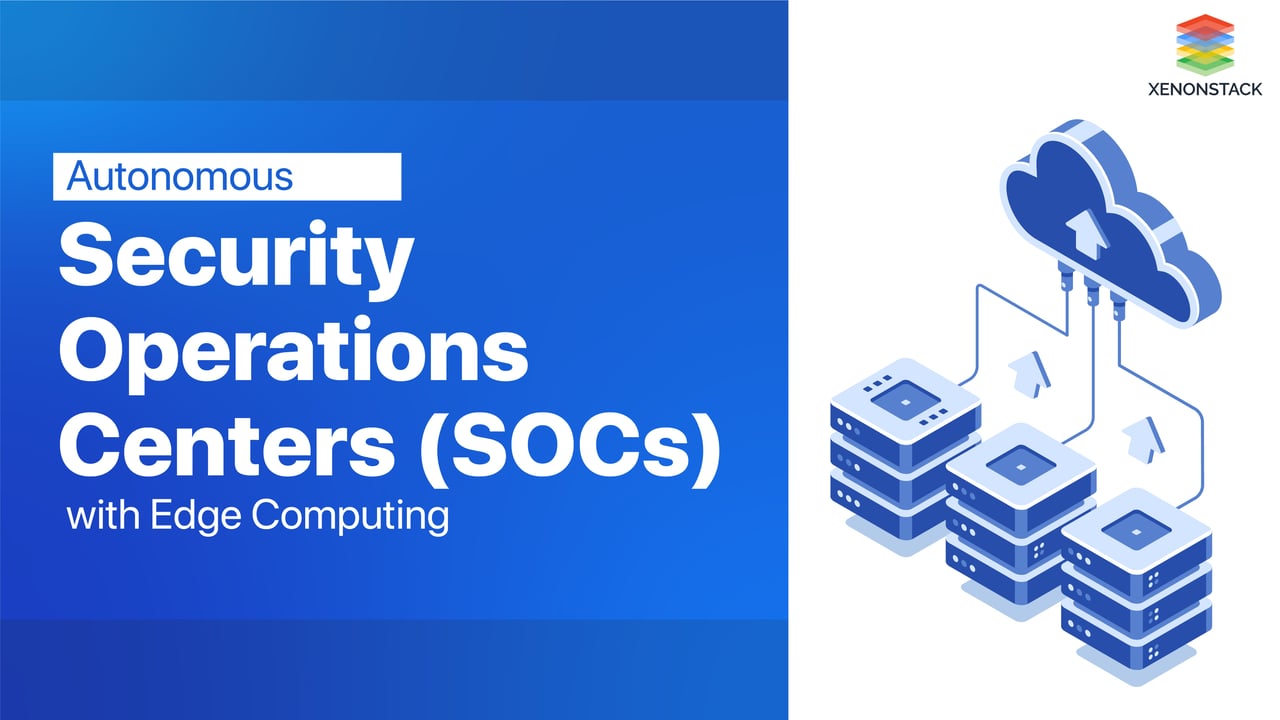
-
Improved Decision-Making
-
Quick Actionable Insights: With edge computing applied, SOCs can use local computing to process the data and generate insights quickly, thus improving their working efficiency.
-
Automated Responses: Specifically, the elements of decision-making can be implemented on edge devices. For instance, in the case of detected attempts to unauthorized access, the current account may be locked, or the security personnel may be notified of the situation without always waiting for a signal from the central server.
-
Resource Optimization
-
Efficient Data Processing: By analyzing the data locally, the SOC can identify how to manage its resources effectively, ensuring that the key systems do not get bogged down.
-
Cost Savings: Less information sent to headquarters servers results in huge savings on bandwidth and disk space. Substantial centralized structures can be reduced while still achieving very good security, which allows organizations to save money.
-
Scalability: Edge computing within SOCs makes scaling efficient. Network changes as organizations expand and need heightened security, which requires incorporating new edge devices that can be effortlessly implemented without affecting established operations. This scalability lends flexibility to SOCs so that, if and when required, the scope of their performance can increase in synchronization with the organization.
The deployment of edge computing at the base layer of the autonomous SOCs serves to revolutionize the functions of threat identification, assessment, and management by improving speed and overall utilization of resources. Through real time threat identification, distributed analysis, enhanced decision making and efficient resource consumption edge computing assists SOCs to stay poised and not reactive. This flexibility is especially crucial in an environment where the level of cyber threats keeps on rising, thus aiding organizations in protecting their resources better and maintaining a proactive security posture while minimizing response times and operational risks.
Use Case: Practical Application of Edge Computing
Scenario: Enhanced Security in IoT Environments
In different sectors, organizations use many IoT devices to track essential processes, identify the location of their assets, and address their processes. All of these devices are data-intensive, and such data may indicate security threats that range from simple unauthorized access to unusual activity or system faults.
Edge Implementation:
-
Local Data Processing: Every IoT device is fixed with edge computing that processes data on demand and in real-time. For example, a temperature sensor is attached to walls in a production line; it will identify unsteady readings that may indicate faulty machinery or vandalism.
-
Anomaly Detection: If a device discovers that, for instance, the computer temperature has risen or its access has been attempted, it can alarm an organization's SOC without relaying all censored raw data collected to a central server. Instead, it sends an abstract of a brief message regarding the anomaly and even more context to avoid excessive data transmission.
-
Automated Response: The SOC can leverage this data to perform reactive actions like stopping functioning equipment that has been compromised, blocking or denying points of entry, or notifying the staff or clients about the threat. These actions can be in real-time, provided that the organization can respond to the threats in good time.
-
Feedback Loop: The system can also update these occurrences and adjust its detection parameters from the former and new threats. Such a feedback loop raises the security level by responding to new threats and increasing the effectiveness of threat recognition over time.
Applying edge computing to IoT settings allows various organizations across industries to improve security systems, increase reaction rates, use resources efficiently, and provide better protection against new and emerging threats.
Conclusion: The Future of SOCs with Edge Computing
However, integrating edge computing into further autonomous SOCs is a new cybersecurity feature. Reducing data or information processing at the edges means that aid organizations can get improved response time, better results in threat detection, and better operations. The rapid evolution of threats in the cyber world means that traditional approaches yield innovative ones like edge computing. Adopting this mindset not only improved an organization's defence but also better-equipped an entity to face the threats in a constantly evolving environment. As each second becomes critically valuable in the business world, edge computing is a great opportunity for SOCs to act more rapidly and protect enterprises across industries from cyber threats, leading to a more secure future.
- Discover More Security Operations vs Network Operations
- Read More Role of Edge AI in Automotive Industry