Understanding AI in Fraud Detection
Fraud prediction is crucial in the digital age because of the increased number of online tools. Criminal activities have increased, too. It is now easier to commit online crimes and not get caught. Understanding AI in Fraud detection is essential to mitigating risks, especially in cases like credit card fraud, where perpetrators steal someone's credit or debit card information to make unauthorized transactions.
As the world shifts towards digital payments and online data sharing, safeguarding measures have become essential to protect users' personal information and financial resources. Fraud Prediction Using AutoAI is an advanced method that leverages AI to predict and stop fraud before it occurs.
Fraud prediction has become more accessible with the rise in data storage capacity, computational power, and Enterprise AI Platforms. Each transaction generates data—such as the amount debited or credited, time, location, item sold/bought, and transaction platform.
By leveraging enterprise AI architecture, this data can be analyzed to detect patterns in user activities and flag unusual behaviors. AI models process vast amounts of data in real time, allowing for AI-driven quality control in fraud prevention. Automated actions can be taken to restrict access or halt transactions when anomalous behavior is detected, strengthening fraud detection mechanisms.
Key Technologies: Machine Learning, Neural Networks, and Behavioral Analytics
-
Machine Learning (ML): Fraud detection systems use ML algorithms to enhance their ability to "learn" from previous transactions. ML models detect fraud by identifying anomalies within datasets, even without explicit programming to recognize specific threats. Enterprise AI use cases in fraud detection showcase how ML-driven solutions improve financial security.
-
Neural Networks: Neural networks are a subset of ML and pattern data based on the structure of neurons in the human brain. They are particularly suitable for discovering preparatory or implicit patterns in financial transactions that may involve fraud.
-
Behavioral Analytics: Behavioral analytics focuses on each user's activity and flags anomalies other than previous trends. Parameters like transaction frequency, spending habits, and even typing speed are analyzed. If a user suddenly conducts unusual large transactions, the system flags it as potential fraud. Enterprise AI Chatbot Platforms can be integrated into security protocols to provide instant alerts and responses to suspicious activities.
How AI Detects and Prevents Fraud Efficiently
Real-Time Transaction Monitoring
Real-time enforcement sets up a network of computers to watch the flow of money through the entire organization to detect any peculiarity in every case of exchange. When a transaction appears to be anomalous in one way or another, for example, the amount being transacted is much higher than usual or is taking place in an area the client has never used before, the AI system can alert the user to observe the transaction more closely, or the system can even put a block to the transaction if the risk level is low. When the high-risk score has been calculated, it will be forwarded to a human reviewer for him to review and then store the result in the database—fraud control benefits from real-time monitoring to reduce the impact of fraud in organizations.
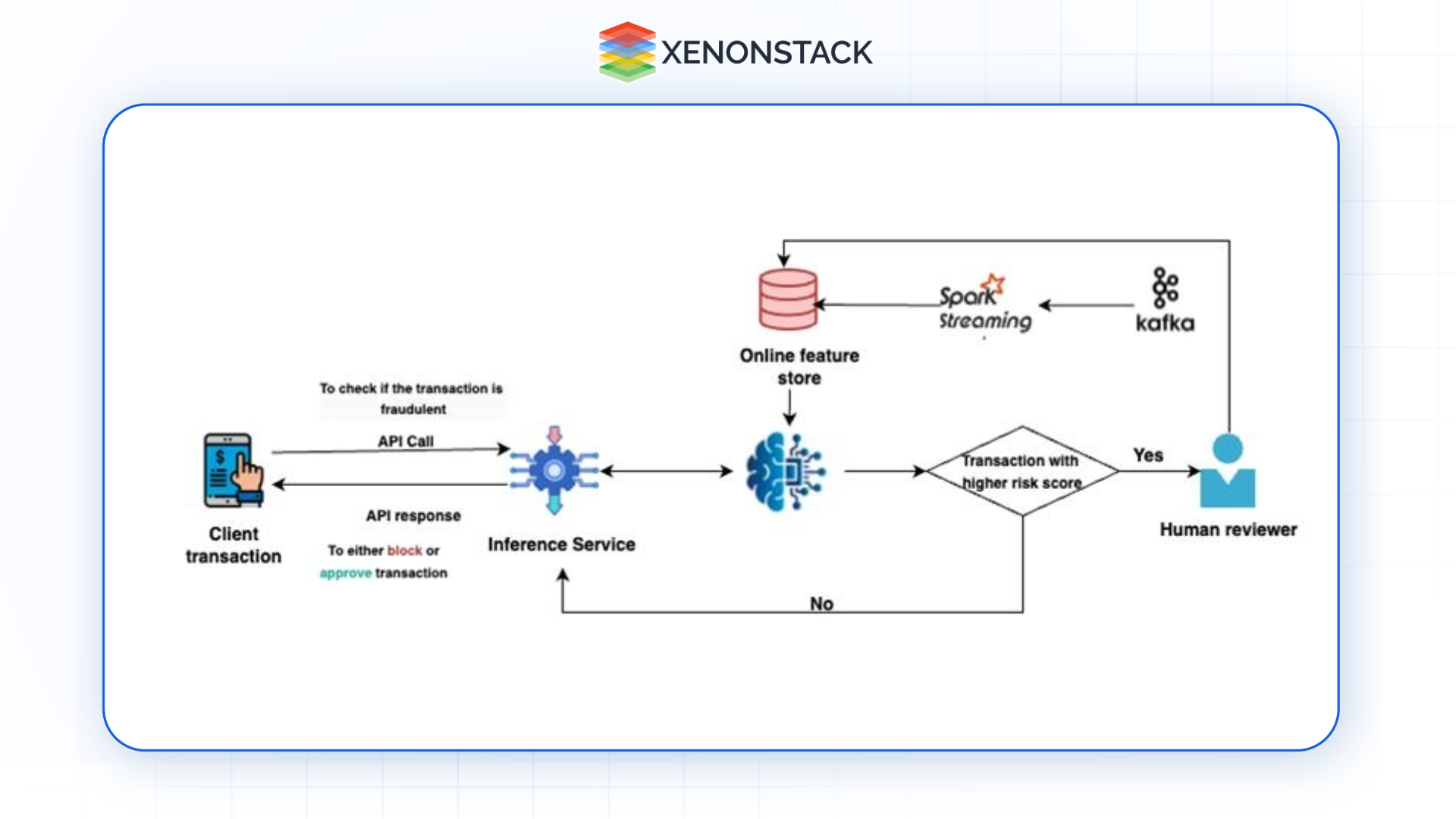
Pattern Recognition and Anomaly Detection
AI also gains a mastery in working with big data and identifying patterns in such data. AI models can obtain the level of such users’ activity through the former by using historical transaction data to create a typical behaviour pattern. Where a transaction is not congruent with these patterns, the algorithm raises the alarm and investigates possible fraud cases. That is much more flexible and progressive than rule-based systems, which will likely only single out particular forms of fraud.
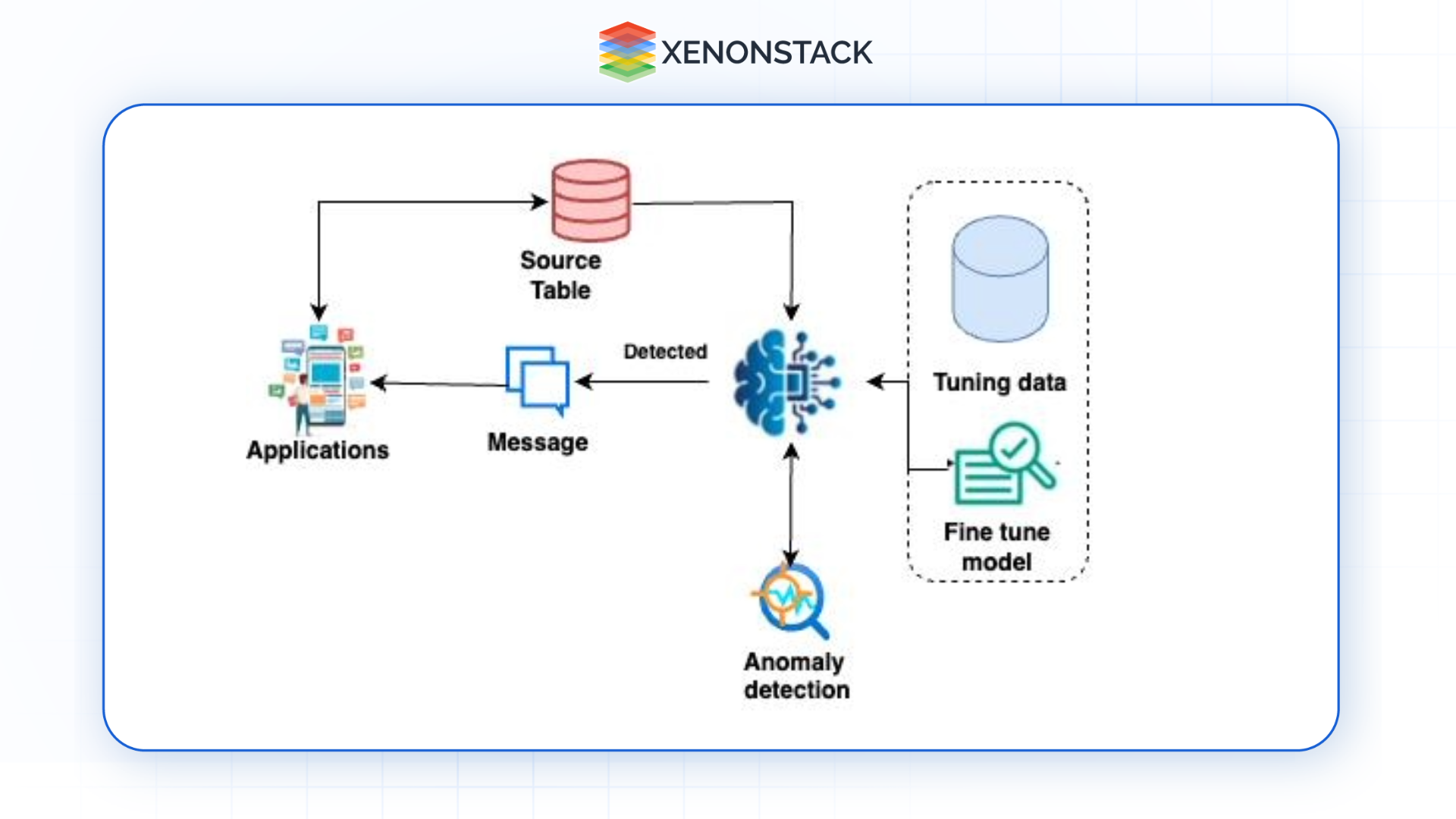
Behavioral Biometrics and User Profiling
Behavioural biometrics involves analyzing how individuals interact with devices, such as how they type, swipe, or navigate websites. By establishing a baseline of a user’s behaviour, AI systems can detect when someone other than the account holder is attempting to make a transaction. Combined with user profiling, AI can monitor a user's typical behaviour and flag any significant deviations that may suggest fraudulent activity.
Top Fraud Prediction Methods Using AI Models
Data preparation and cleaning become crucial with the ever-increasing data related to user transactions and logs. Machine learning pipelines can be developed to handle organised and clean unorganised data.
Supervised Machine learning
Supervised Machine learning has been used to classify fraud or non-fraud cases after learning from past data. Unlike rule-based analysis, where we manually set rules to label the user or transaction as fraud or non-fraud, supervised machine learning tries to learn the parameters or rules by iterating over past data. When it comes to ML, fraud prediction models can be divided into two parts, namely:
Profile-based models
In the profile-based approach, the models classify users as fraudulent or not based on their transaction history, e.g., identifying spam or duplicate accounts.
Transaction-based models
Transaction-based approaches classify transactions rather than users as fraudulent. Sometimes, the user's identity is compromised, and their credentials are used to extract information or money from their account. Transaction-based models can detect fraudulent activities in a specific transaction to alert the authorities.
Unsupervised Machine Learning
Unsupervised ML can be applied to identify users or general transaction patterns on a platform to detect anomalies in behaviour. User profiles can be created by analyzing features such as spending habits, where they spend most, how much, etc. If the system detects unusual transactions, it can alert the user or lock their account or card. Principal Component Analysis and Deep Learning Auto-encoder are some methods that can be used to create models that create new features from original features to cluster the transactions as fraudulent or not fraudulent more effectively.
Case Studies: Success Stories in AI-Powered Fraud Detection
Example 1: Banking Sector
Banks worldwide are implementing AI-powered fraud detection systems to protect their customers. For example, JPMorgan Chase employs machine learning algorithms to flag potentially fraudulent transactions in real-time, offering users an enhanced level of security.
Example 2: Payment Processors
Companies like PayPal and Stripe use AI to prevent fraud in digital payments. These payment processors utilize ML algorithms to detect unusual activity during transactions, such as mismatched billing addresses or suspicious IP addresses, ensuring safe and secure transactions for millions of users globally.
Example 3: E-Wallet Security
E-wallets like Apple Pay and Google Pay leverage AI to secure digital wallets. These platforms can detect unauthorised access attempts or fraudulent activity by analyzing user behaviour and transaction data. They also use AI to improve user authentication through biometrics and other advanced security methods.