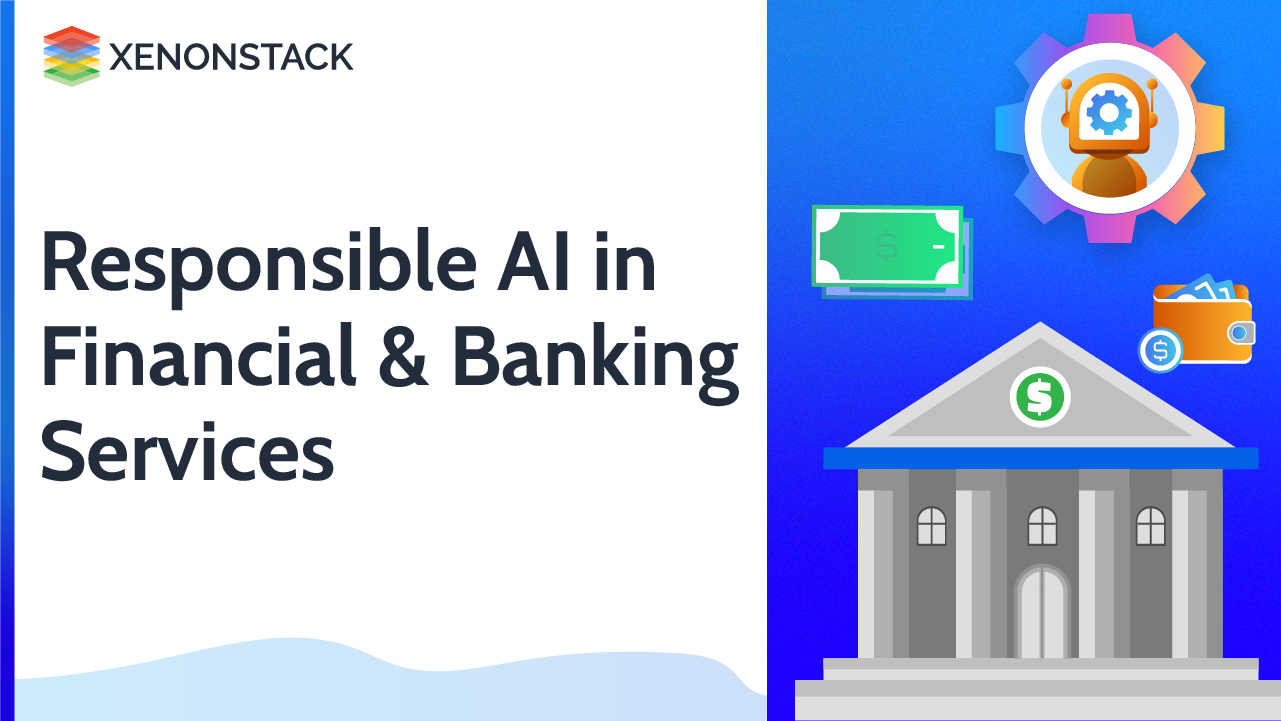
Introduction to Responsible AI in Finance
According to the Indian financial Institutions survey, introducing AI in financial services increases customer satisfaction. The top three uses of AI in financial services are chat automation, fraud detection, and AI virtual assistants. Among the respondents, 57% says AI in Financial service gives a competitive edge in the business firm. As per a survey, 83% of organizations develop well-defined AI strategies, and 65% of the rest work to implement AI.
Role of Artificial Intelligence
Artificial intelligence is changing the financial services (FS) industry. One of the ways banks are using AI is to improve customer experience and engagement. FS businesses now use chatbots to improve customer service and gather customer feedback and other needed information. Many organizations have developed purely virtual assistants like Apple's Siri or Amazon's Alexa. It helps customers find products and also helps with financial transactions.
However, organizations run into problems if they do not show sufficient care and attention to their AI applications. It includes biases in processes and results when analyzing input data, customers and credit scores, and risk due diligence in the supply chain. AI analytics users need a good understanding of the data used to train, test, iterate, adapt, and deploy AI systems. This is important when third parties provide analysis, or analysts generate data from third parties and platforms. There are concerns about the appropriateness of using big data in customer profiles and credit scores.
Artificial intelligence is helping banks become more efficient in the process of detecting fraud and Robotic Process Automation. Taken From Article, Applications of AI in Banking and its Benefits
How to implement Responsible Artificial Intelligence?
The AI mission is a regulatory framework that documents how the organization provides fair and legal solutions to artificial intelligence (AI)-related problems. Removing the uncertainty about who will be held accountable if something goes wrong is essential to responsible AI initiatives.
What are the Principals of Responsible AI?
AI and the machine learning models that support it should be comprehensive, explainable, ethical, and efficient.
- Comprehensiveness – Comprehensive AI has well-defined testing and governance criteria to ensure machine learning is not easily hacked.
- Explainable AI is programmed to explain the purpose, rationale, and decision-making process in a way that the average end user can understand.
- Ethical AI Initiative has implemented a process to find and remove bias from machine learning models.
- Efficient AI can work continuously and respond quickly to changes in the operating environment.
Responsible AI Implementation and its Working
Performance demonstration is complex for the algorithmic model from the responsibility standpoint. Organizations are developing responsible AI, and it will eliminate black-box AI models. Below are a few strategies for responsible AI development:
- Ensure data is explainable in a way that a human can interpret.
- Design and decision-making processes must be documented to the point where reverse engineering can be performed in case of a mistake.
- To help mitigate bias, promote constructive discussions and diverse work culture are required.
- Use interpretable latent features to help create human-understandable data.
- Create a rigorous development process that values visibility into each application's hidden features.
What are the Best Practices of Responsible AI?
Its design and governance process needs to be repeatable. Some best practices are below:
- Make every effort to be transparent to explain all decisions made by AI.
- Responsible Design. Analyze responsibilities early in development.
- Make performing tasks as measurable as possible. Responsibility can sometimes be subjective, so it is essential to have measurable processes such as visibility, explainability, and a verifiable technical or ethical foundation.
- Use reliable AI tools to validate AI models.
- Available options include Explainable AI and TensorFlow Toolbox. Also, perform tests such as displacement tests or preventive maintenance.
- Be careful and learn along the way. Organizations learn more about responsible AI during the implementation process. From Fair Practices to Technology Handbooks and Resources on Technology Ethics.
Explainable AI can solve increasing regulatory demands and enable fairness audits on various dimensions, including race, gender, and income. Taken From Article, Explainable AI in Finance and Banking Industry
Responsible AI Changing Financial Services
Artificial intelligence (AI) is today's biggest bet in finance. Using new solutions can help financial institutions, and banks beat their competitors by helping companies improve their products in a rapidly changing and unpredictable world.
Artificial intelligence has passed its experimental stage and has been successfully applied in real applications. Banks use artificial intelligence bots to attract customers and take the risk of borrowers. Use computer vision, pattern matching, and deep learning to identify ineffective systems. AI-based financial protection solutions help prevent fraud, among many other uses. Financial institutions and financial institutions are combining artificial intelligence with other new technologies to change the game's rules.
Examples of AI Applications in Financial Market Activities
AI applications in financial market activities are:
Risk Assessment
The banking sector is one of the most prominent examples of the FS industry. Banks and apps use machine learning algorithms for more than just determining a person's eligibility for a loan. The most significant advantage of artificial intelligence is that it is not biased. It can be used more quickly and accurately to determine loan eligibility.
Risk Management
Risk mitigation has always been an important and ongoing task in the banking industry (and almost every other industry). Machine learning can help experts use data to "pinpoint trends, identify risks, conserve manpower, and ensure better information for future planning," according to Built In.
Fraud Detection, Management, and Prevention
After several credit card purchases, the user receives a phone call from the credit card company. It happens when someone uses a credit card many times, and such use is exceptional. AI systems trigger some calls or messages on behalf of the credit card company to alert the user. The AI system often blocks the credit card for a specific period for security reasons.
Credit Decisions
Towards Data Science explains that artificial intelligence can quickly and more accurately assess a potential customer based on various factors, including smartphone data (plus, machines aren't biased.)
Governance of AI Systems and Accountability in Financial Services
AI governance combines the policies, practices, and processes that drive and govern AI. AI management aims to enable organizations to leverage AI while reducing costs and risks.
Especially since the industry is well regulated, financial institutions should establish a robust AI management system to monitor AI strategies better. The framework should include a clear strategy for using AI and data collection and management guidelines. Equally important is the need to coordinate to identify and mitigate risks to better secure data and comply with the law.
A hypothesis and set of general prescribed procedures that separate the divisions, or storehouses, between the conventional IT arms of activities. Taken From Article, FinDevOps - Merging Financial Services with DevOps
Relevant Issues and risks stemming from the Deployment of AI in Finance
Consumer protection risks increase in machine learning models used for credit score risk; here, insufficient information can increase the risk of differences in credit benefits and related, discriminatory, or unfair lending practices. AI-driven models distinguish between hard-to-know loan allocations, in addition to creating or creating bias. In contrast, the results of the AI model can be more clearly identified and communicated to reject potential credit.
While many of the risks associated with AI in finance are not specific to AI, they result from the complexity of the technology, the changing nature of AI-based models, and their horizontal freedom for cutting-edge AI applications. The complexity and complexity of describing and reproducing the decision-making processes of AI algorithms and models make risk mitigation difficult. Contradictions in cognitive skills and the complex nature of AI models, and the human need for reasoning and interpretation, more translation problems arise as human knowledge increases.
Why should every financial institution consider Responsible AI?
Below discussed are the reason behind the use of responsible AI in financial institutions.
- Responsible AI is more than just a buzzword or a fad that the financial services sector can weather. It's the cornerstone of the industry-wide mission to ensure that FIs consistently make fairer, ethical decisions that ultimately positively impact people's lives. FIs that commit to a fairer AI framework can rest assured that when automated decisions are made, Fls are much less likely to unfairly deny loans or stop people from paying their bills because of their race, gender, age, or where users live.
- Financial institutions today face three primary forces of change: technological advances, increased customer expectations, and regulatory scrutiny. Today's consumers want a digital identity, including financial services. To achieve this goal, businesses need to understand and use new technologies, including artificial intelligence, today and in the future. With its ability to collect and analyze massive amounts of data, AI can revolutionize business and provide the insights customers need in financial services to deliver personalized service. Organizations that must incorporate AI into their strategies will face competition over the next decade.
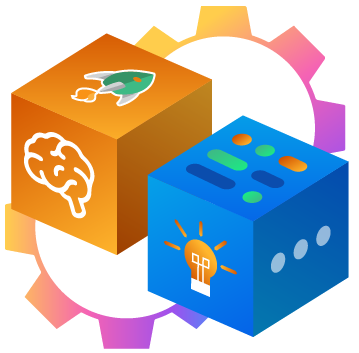
Conclusion
Microsoft has created its own responsible AI governance structure through the AI, Ethics, and Effects in Engineering and Research (AETHER) committee and the Responsible AI Authority (ORA) group. These two groups work together at Microsoft to promote and support specific responsible AI values. ORA is responsible for setting enterprise-wide rules for responsible AI, specifically through the implementation of governance and public policy actions. Microsoft has implemented several responsible AI guides, checklists, and templates.
- Know here about Credit Fraud Detection with Deep Learning
- Read more about Explainable AI in Auto Insurance Claim Prediction.