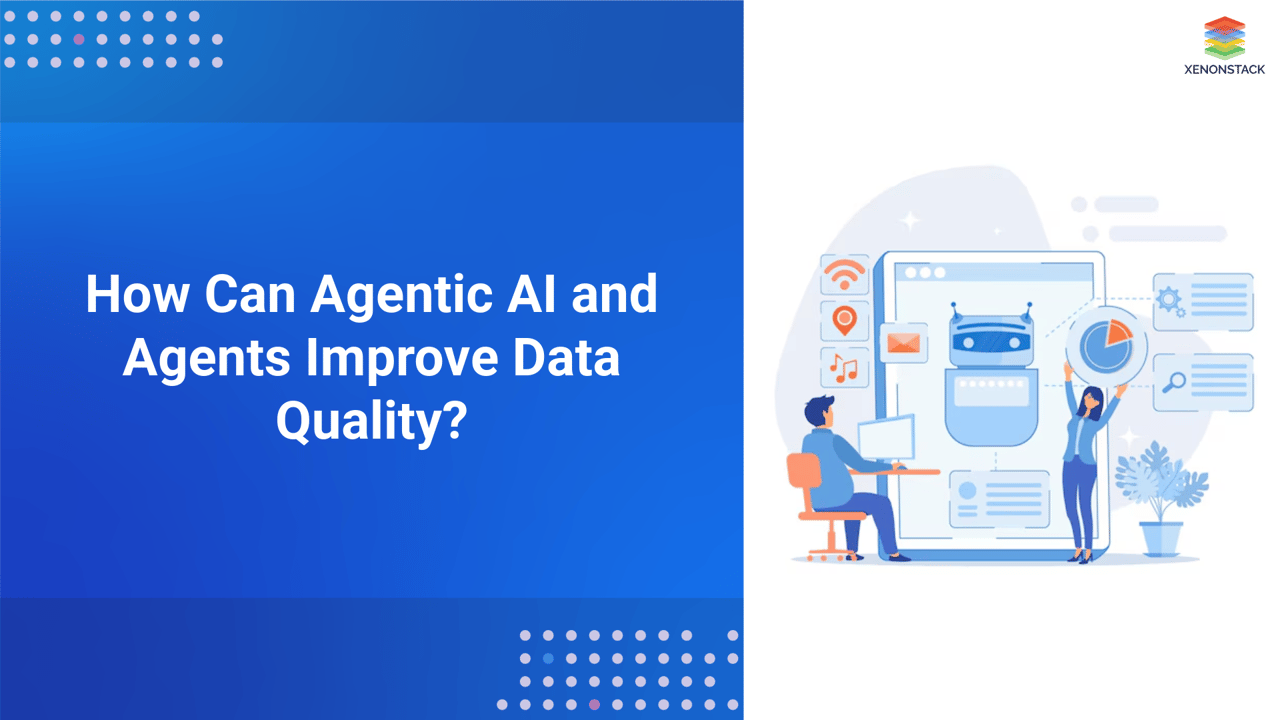
How Agentic AI Enhances Data Quality
1. Automated Data Cleaning
Agentic AI systems autonomously detect and correct anomalies such as duplicate records, missing fields, and formatting errors. They can continuously monitor datasets and apply corrective actions without human intervention, dramatically improving speed and accuracy.
2. Real-Time Data Validation
Traditional data validation happens in batches, which delays correction. Intelligent agents can perform continuous real-time validation, flagging or correcting errors as soon as data enters the system.
3. Dynamic Data Standardization
Agentic systems can learn the preferred formats for various data types and dynamically apply standardisation rules. This ensures consistency across departments, applications, and databases.
4. Intelligent Metadata Management
Agents can auto-generate metadata, track data lineage, and map interdependencies. This not only improves data discoverability but also enhances transparency and compliance.
5. Adaptive Data Governance
Agentic AI ensures that data governance policies are actively enforced. Agents can detect policy violations, automatically rectify issues, and alert stakeholders in real-time.
Real-World Applications of Agentic AI in Data Quality
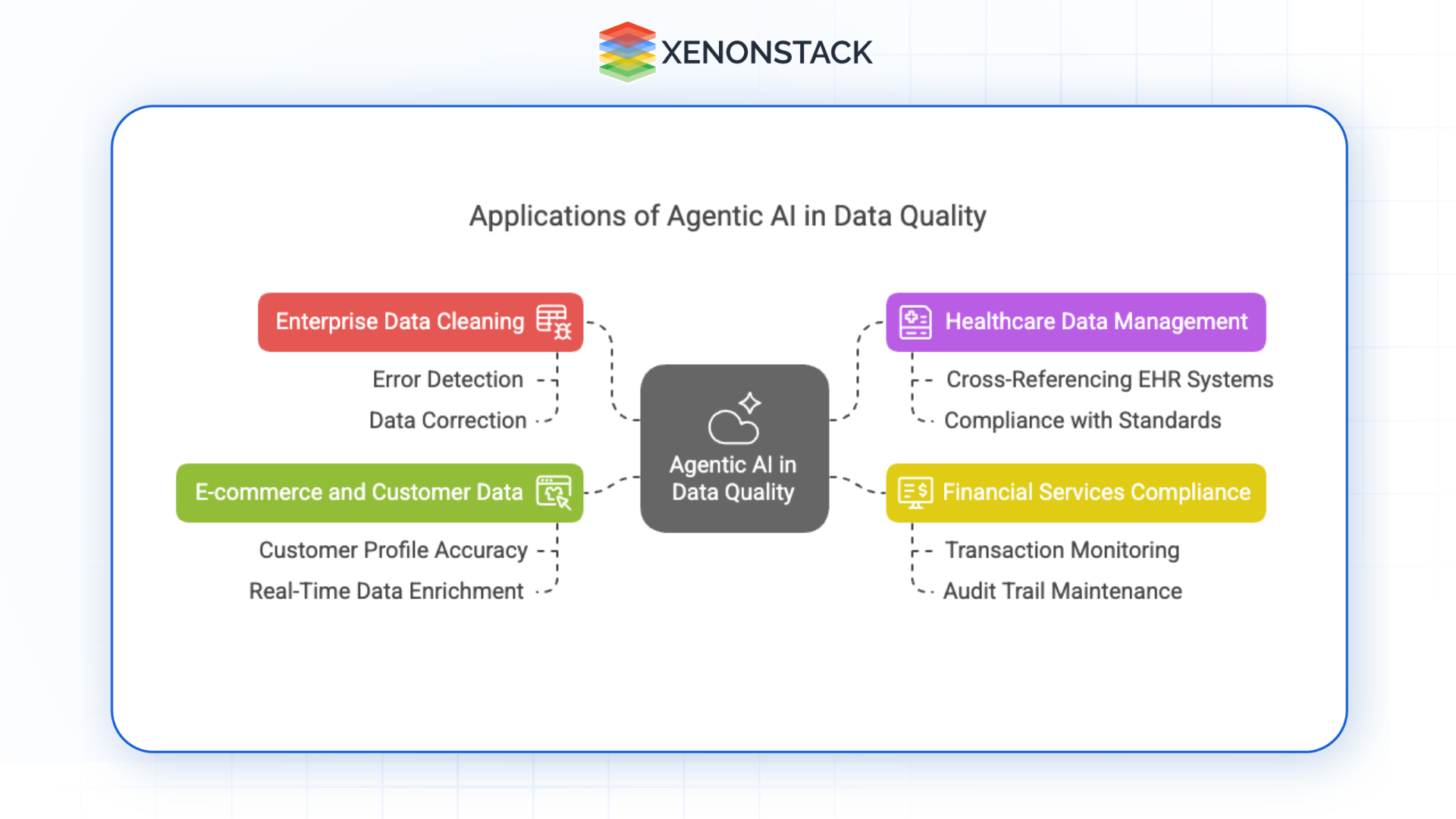
Enterprise Data Cleaning
Data often comes from disparate sources and in multiple formats in large organisations. Agentic AI automates error detection and correction, ensuring clean, high-quality data for analytics and operations.
Healthcare Data Management
Maintaining accurate patient records is critical in healthcare. Intelligent agents can cross-reference data between EHR systems, correct inconsistencies, and ensure compliance with medical data standards.
Financial Services Compliance
Financial institutions are subject to stringent data regulations. Agentic AI can monitor financial transactions, validate report data, and maintain an audit trail for regulatory compliance.
E-commerce and Customer Data
In e-commerce, personalized recommendations and customer experiences hinge on clean, accurate data. Agentic AI can ensure customer profiles are correct, deduplicated, and enriched in real-time.
Benefits of Agentic AI for Data Quality Management
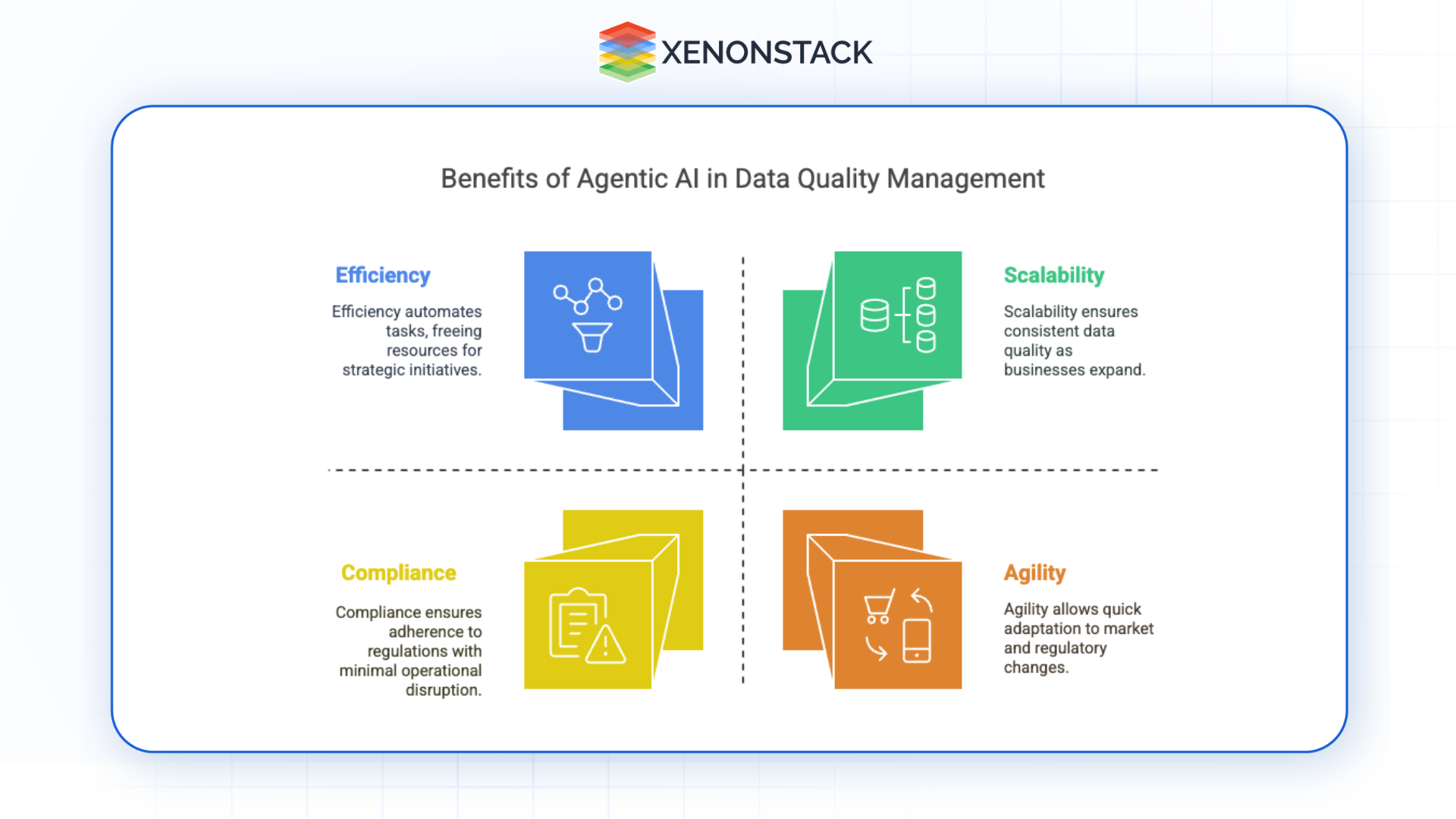
1. Scalability
Agentic AI can seamlessly handle massive volumes of data across various sources and formats. Unlike manual approaches that strain under scale, intelligent agents thrive in data-rich environments. They adapt to growth, enabling consistent data quality as businesses expand.
2. Efficiency
By automating repetitive and time-consuming data tasks—such as data cleansing, validation, and integration—agentic systems free human resources to focus on more strategic initiatives. This efficiency translates to faster time-to-insight and reduced operational costs.
3. Accuracy
Through constant learning and real-time monitoring, agentic AI can significantly reduce errors and anomalies in data. Their ability to contextualize data inputs allows them to make more informed corrections, leading to higher data fidelity and reliability.
4. Compliance
Agentic AI agents can continuously monitor data pipelines for regulatory compliance. They can enforce data retention policies, detect access violations, and automatically generate audit logs, ensuring that the organization stays compliant with GDPR, HIPAA, and CCPA laws.
5. Agility
Businesses need to respond to market shifts and regulatory changes quickly. Agentic systems can be retrained and reconfigured to accommodate new data structures, rules, or objectives—providing a flexible foundation for evolving data management needs.
Best Practices for Implementing Agentic AI for Data Quality
1. Assess Data Readiness
Begin by conducting a comprehensive data audit to identify gaps in data quality, existing infrastructure limitations, and integration challenges. This foundational understanding will guide your implementation roadmap.
2. Define Clear Objectives
Set measurable goals for what you want the agentic AI system to achieve—whether improving accuracy, speeding up data validation, or enhancing compliance. Well-defined KPIs will help track progress and justify ROI.
3. Choose the Right Tools and Platforms
Select AI platforms that support agentic capabilities and can integrate with your existing systems. Look for features such as automated workflows, real-time monitoring, and low-code/no-code interfaces to simplify deployment.
4. Engage Stakeholders Across the Organization
Collaboration is key. Involve data stewards, compliance officers, IT teams, and business users early in the process. This ensures goal alignment, fosters trust in AI systems, and accelerates user adoption.
5. Establish Continuous Monitoring and Feedback Loops
Implement dashboards and performance metrics to monitor the effectiveness of agentic AI systems. Use user feedback and system performance data to refine rules, retrain models, and optimize workflows.
6. Ensure Ethical and Transparent Use of AI
Build explainability and transparency into your agentic systems. Make sure decisions made by AI agents can be traced and justified, especially in regulated industries. Regular audits and ethical guidelines will help maintain trust.
Conclusion: Future-Proofing Data Quality with Autonomous Intelligence
Agentic AI is revolutionizing how we think about data quality. With the ability to act autonomously, these intelligent agents bring unprecedented accuracy, efficiency, and compliance to data management processes. As businesses evolve in a data-first world, embracing Agentic AI is no longer optional—it’s a competitive necessity. By integrating these systems today, organizations can ensure data integrity, support innovation, and position themselves for a brighter, more resilient future.