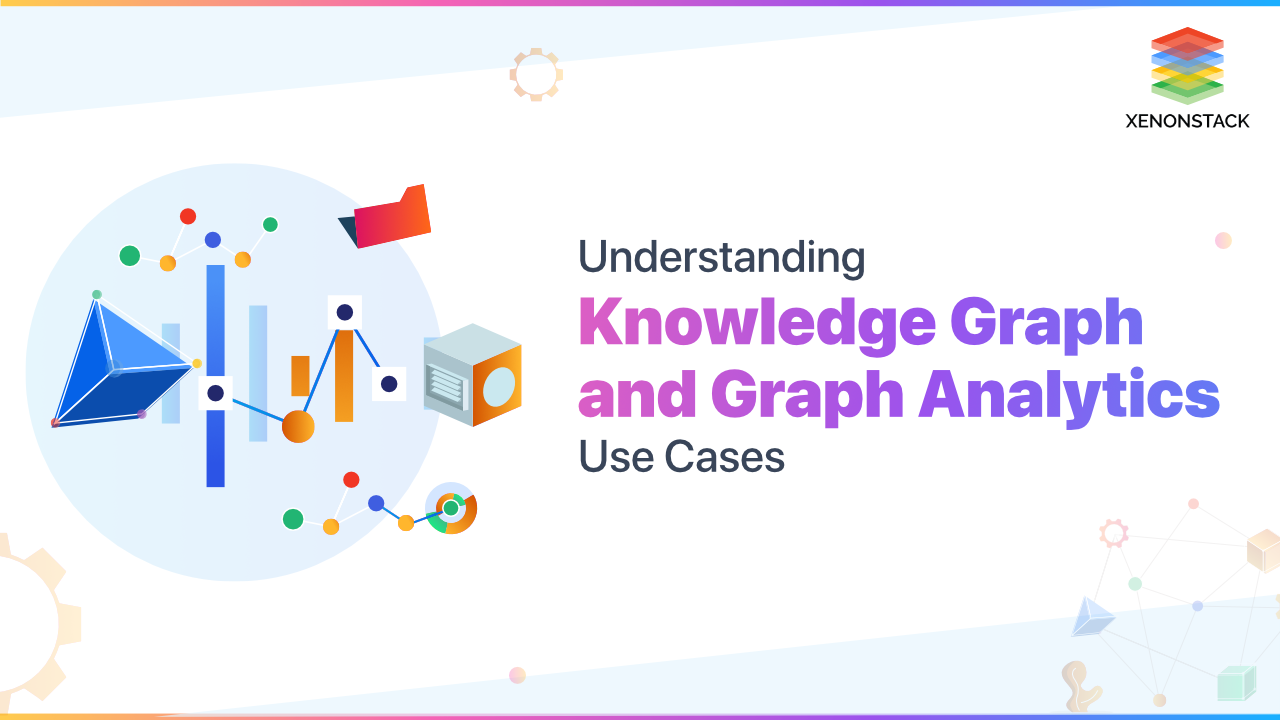
Introduction to Graph Analytics
Graph analytics is a new field of data analytics that helps businesses leverage their model by adopting a variety of its algorithms to identify the best solutions for their challenges. Each algorithm analyzes connections uniquely, revealing new information. They reveal what's going on in a network, such as who has the most influence, is well-connected, member of a sub-network, etc.
Major vendors in the global graph analytics market include Microsoft (US), IBM (US), AWS (US), Oracle (US), Neo4j (US), TigerGraph (US), Cray (US), and DataStax (US).
Streaming visualizations give you continuous information examination and BI to see the patterns and examples in your information to respond to all the more rapidly. Click here to know more about Real-Time Streaming Data Visualization
A knowledge graph, also known as a semantic network, depicts the relationship between real-world entities such as objects, events, situations, or concepts. Knowledge graphs can aid in data governance, fraud detection, knowledge management, search, chatbot, recommendation, and intelligent systems across multiple organizational units.
Types of Graph Analytics
There are four types of graph analytics:
Path Analysis
This type of analysis looks at the connections between nodes in a graph. To put it another way, it finds the shortest path between two nodes.
Connectivity Analysis
It aids in comparing network connectivity by highlighting how strongly or weakly two nodes are linked. This is useful for determining how many edges flow into a node and how many flow out of it.
Centrality Analysis
This method determines how essential a node is to the network's connectivity. It uses ranking to locate the most influential people in a social network or find the most popular web pages.
Community Analysis
This is a distance and density-based analysis of relationships among groups used to locate groups of people who regularly engage with each other in a social network. It also identifies whether individuals are transient and can predict if the network will grow.
How is Graph Analytics different than regular analytics?
Aspect | Regular Analytics | Graph Analytics |
---|---|---|
Focus | Based on statistics, computer programming, and operations exploration | Focuses on identifying patterns and relationships between entities or nodes |
Algorithms | Uses general algorithms based on statistical methods | Uses graph-specific algorithms like Clustering, Partitioning, PageRank, and Shortest Path |
Clustering | General statistical methods for grouping | Groups nodes based on edge weights or distances |
Partitioning | Not specific to regular analytics | Minimizes the group of adjacent nodes or edges that are not in the same partition |
PageRank | Not applicable | Measures the importance of each node based on incoming relationships and source nodes |
Shortest Path | Not directly applicable | Identifies the shortest paths between all pairs in a weighted graph |
Data Retrieval | Uses SQL for querying and retrieving data | Uses graph database queries for faster retrieval of connected data |
SQL Joins | Often requires multiple joins between tables | No need for SQL joins; relationships are inherent in the graph structure |
Performance | SQL joins can be slow, especially with large datasets | Graph databases handle relationships faster (in microseconds) compared to SQL queries (in milliseconds) |
Storage and Relationships | Tables store data in rows with connections requiring joins | Data is stored in nodes and edges, with relationships explicitly defined |
How does Graph Analytics work?
In graph analytics, we store, manage, and query data in the form of a graph, as the name implies. Our "entities" or "nodes" become vertices, while "edges" are associations that serve as communication lines between the various entities. Furthermore, an edge can be a line that runs in one or more directions, or it might be a line that runs in no direction at all. It also has a "weight" associated with it, representing the relationship's strength.
For instance, Knowledge Graph - A Powerful Data Science Technique for Mining Information from Text.
Use Cases of Graph Analytics
Graph analytics use cases for telecom, journalism, social networks, finance, and operations.
Compliance
Graph analytics help to spot frauds and unlawful actions such as money laundering and payments to sanctioned entities. Analysts use the data of social media to detect criminals. They use texting, phone calls, and emails to create a graph that shows how these data are related to criminals’ records. Government agencies can identify the threats from non-obvious patterns of relationships from those graphs.
-
Graphs can be formed from financial transactions and can be used to analyze compliance reasons. For example, now banks have to ensure that their customers are not connected to the sanctioned entities.
-
Using social or financial networks formed over these graphs for loan decisions.
Journalism
Graph analytics is being used to identify networks of relationships in the ICIJ (International Consortium of Investigative Journalists) research on Panama Papers. The research emphasizes how authoritarian leaders and politicians used complex sets of shell companies to obscure their wealth from the public. Using graph analytics and document extraction tools to structure the data from thousands of documents on companies in off-shore jurisdictions. They used graph analytics to navigate the documents' structured data to identify those companies' real owners.
National Security
Though considered a controversial topic, national intelligence agencies detect unlawful activity using graph analytics. Online activity of both suspected and not suspected individuals are collected and analyzed to identify non-obvious relationships and identify potential crimes.
Operations
Supply Chain Optimization: In transportation networks, supply chain networks and airline companies use graph analytics algorithms such as shortest path and partitioning as tools to optimize routes.
Fraud Detection: Graph Analytics is used to detect fraud detection in businesses that work with networks involving e-commerce marketplaces, financial institutions, and telecom companies.
Healthcare
Pandemic Search
2020 was a pandemic year in the hands of coronavirus. Being a highly infectious virus, using a graph database helped governments track the spread of this virus. A Chinese company named We-Yun allows Chinese citizens to check if they can contact a known carrier of the virus. The application uses the Neo4j graph database.
Marketing
Recommendation Engines: “People you may know” or “Songs you may like” are some common phrases you hear these days on your social media profiles. Recommendations rely on collaborative filtering, which is a commonly recommended engine. Graph Analytics helps to identify similar users and enables personalized recommendations when using this collaborative filtering.
Social Network Analysis: Social media networks such as Instagram, Linked In, and Spotify are relationships and connection-driven applications. Graph analytics has an application in identifying influencers and communities on social media.
What is a Knowledge Graph?
It is a knowledge base that integrates data using a graph-structured data model or topology. They are frequently used to contain interconnected descriptions of entities, such as objects, events, situations, abstract concepts, and the semantics behind the terminology used.
Simply put, It converts your data into knowledge that machines can understand. But how to tell the difference between data and knowledge? Knowing this answer is key to understanding its definition of it.
The following are examples of real-world knowledge:
-
Situational: the meaning changes depending on the scenario.
-
Layered: the connections between concepts enable more complex comprehension.
-
Changing: discoveries instantly change meanings.
These aspects of knowledge represent the context that data often lacks. Traditional data management systems fail when it comes to capturing context. These failures result in data mastering gridlock, delays in adding new data sources or properties, and missing datasets from analysis, all of which lead to mistrust. On the other hand, Knowledge Graphs are explicitly designed for the changing nature of knowledge. They provide a more adaptable foundation for digital operations by quickly accepting new data, definitions, and needs.
Importance of Knowledge Graph
Because being data-driven isn't enough – knowledge-driven businesses can act with full context and confidence in their judgments.
A database that uses graph architecture for semantic inquiry with nodes, edges, and properties to represent and store data. Click to explore about, Graph Databases in Big Data Analytics
Benefits of Knowledge Graph
The "graph" in Knowledge Graph refers to a method of arranging data that emphasizes relationships between the data points. Graph representation looks similar to a network of interconnected points. This contrasts to relational databases like Oracle or MySQL, which store data in tables. Relational systems are intended for business processes with a consistent data model.
Less rigidity
The emphasis on stability leads to data integration rigidity which is problematic. Data integration platforms based on relational systems necessitate constant data transformation to ensure consistency across all entities. This produces a so-called "unified view" of data but lacks analytical flexibility since it excludes situational, layered, or dynamic reality representations.
A flexible data layer
It is an appropriate tool for enterprise data integration since it can make real-world context machine-understandable. Graphs' capacity to continually link concepts — without affecting the underlying data — is used to unify data instead of joining tables. As a result, data unification connects data silos and provides an organization with a flexible data layer.
How does a Knowledge Graph work?
The working architecture of knowledge graphs is listed below:
Implemented Ontology
Domain knowledge is defined through ontologies, including definitions, relationships, and rules. It collects and organizes data into an ontology (or several ontologies) before making it available to enterprise applications.
Enhanced by Artificial Intelligence
The Inference Engine of the Knowledge Graph intelligently derives new knowledge from data and business logic. When there are competing definitions — for example, a Top Account in one database may be referred to as a Prospect or Churn Risk in another — you can declare in the data model that all of these entities are "Companies" and use inference to examine the relevant data.
Limitless data access
The data that a Knowledge Graph may access determines its power. Virtualization is essential for combining all relevant data into it and enriching it. Knowledge Graphs, in combination with virtualization, provide for complete flexibility in evaluating dependencies across various systems and data types. We can easily trace data lineage, detect connection and causation, and perform root cause and impact analysis.
Unleash Insights
It makes answering questions more easily. Queries and searches, natural language processing, machine learning techniques, visualization, and business intelligence are all simple to prepare.
Examples of Knowledge Graphs
-
DBPedia: This project uses the structure of Wikipedia's infoboxes to construct a massive dataset of 4.58 objects (https://wiki.dbpedia.org/about) and an ontology with encyclopedic coverage of people, locations, films, books, organizations, species, diseases, and more. The Open Linked Data movement is built around this dataset. It has proven highly beneficial for businesses to use millions of crowdsourced entities to bootstrap their internal knowledge graphs.
-
Google Knowledge Graph: With the announcement of its knowledge graph in 2012, Google popularised this concept.
-
Wordnet: One of the most well-known lexical databases with definitions and synonyms for the English language. They are frequently used to improve the performance of natural language processing and search applications.
-
Geonames: Users of the Geonames dataset have access to 25 million geographical entities and features.
Use cases of Knowledge Graph
Analytics Modernization
Traditional graph or relational data architectures lack the access, context, and inferencing necessary to meet the arduous demands for innovating and monetizing advanced analytic solutions.
Data Fabric
Data fabrics provide a more adaptable solution, allowing for the dynamic delivery of semantically enriched data. An Enterprise Knowledge Graph is a critical component in converting existing data infrastructure into a data fabric. This is accomplished by Stardog's platform, which employs a novel combination of graph, virtualization, and inference.
Data Lake Acceleration
Enable data teams to improve analytics insights by streamlining data access and discovery.
Operational Risk
Unifies software and hardware assets with all relevant knowledge touched by these IT systems and applications, laying the groundwork for your operational risk practice
Final Thoughts on Graph Analytics
Graph analytics and its associated technologies can enable the analysis of data and relationships that would be extremely difficult or unachievable using standard analytics approaches. Graph analytics and graph approaches have grown in popularity in analyzing complex data interactions. It is feasible to draw insights in increasingly complicated ways using it, making it a vital tool for today's enterprises.
Next Steps
Talk to our experts about implementing Graph Analytics and Knowledge Graphs. Learn how industries and different departments leverage graph-based workflows and decision intelligence to become more data-driven. Knowledge graphs help organizations better organize, query, and analyze vast amounts of interconnected data, transforming how decisions are made. By utilizing graph analytics, businesses can automate and optimize data management, enabling deeper insights, improving efficiency, and enhancing responsiveness across IT operations and support.