The oil and gas industry, long known for its complex infrastructure, capital-intensive operations, and high-risk environments, is on the brink of a transformative era. With increasing pressure to improve operational efficiency, reduce environmental impact, and ensure workforce safety, companies actively seek innovative solutions beyond traditional automation. Agentic AI, with its autonomous, goal-directed, and context-aware agents, offers a new frontier in operational intelligence.
This blog explores how Agentic AI and intelligent agents are revolutionising the oil and gas value chain—from exploration to downstream operations—while addressing industry challenges with a human-and-agent-centred approach.
Understanding Agentic AI in the Oil and Gas Context
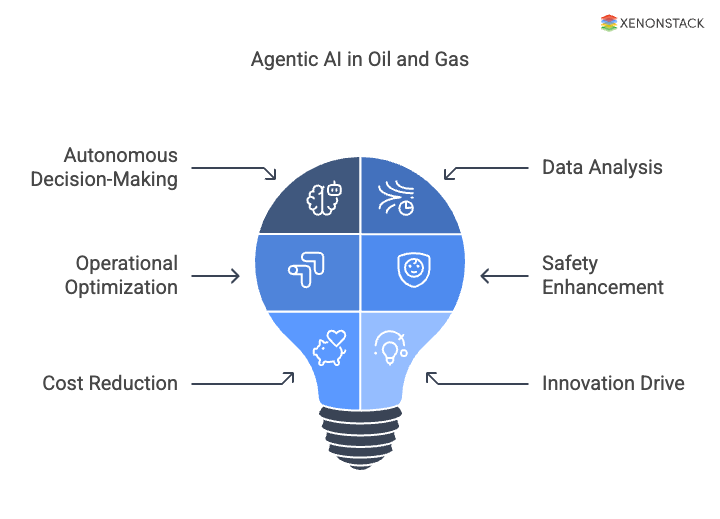
Intelligent agents in this context are software entities embedded across physical infrastructure, digital systems, and decision-making processes. These agents gather sensor data, assess scenarios, suggest decisions, and execute operations autonomously or semi-autonomously.
Industry Challenges: Why the Oil and Gas Sector Needs Agentic AI
-
Complex Supply Chain and Operations
Managing resources, timelines, and safety protocols is immensely complex from offshore drilling to refinery logistics. -
Ageing Infrastructure and Workforce
Many facilities operate with outdated systems and a retiring workforce, creating a knowledge and skills gap. -
Environmental and Regulatory Pressures
Carbon emissions, environmental compliance, and ESG reporting demand accurate and real-time monitoring. -
High Operational Risks
Hazardous environments, unpredictable drilling conditions, and equipment failures make safety a priority. -
Data Overload
The industry generates terabytes of data daily from seismic imaging, sensors, and SCADA systems, which are often underutilised due to a lack of integration or intelligence.
How Agentic AI and Agents Address These Challenges
1. Exploration and Seismic Analysis
Agent Role: Geospatial Exploration Agent
Function:
-
Analyse vast seismic datasets and geological models in real time
-
Recommend optimal drilling locations based on probability, cost, and risk analysis
-
Perform continuous risk assessments for potential subsurface anomalies
Key Outcomes:
-
Reduced time-to-discovery
-
Lower exploration costs
-
Minimised environmental disruptions
2. Drilling Operations and Rig Management
Agent Role: Autonomous Drilling Agent
Function:
-
Monitor and adjust drill bit parameters in real-time using sensor data
-
Predict failures before they occur and trigger preventive maintenance routines
-
Coordinate with safety agents during high-risk operations
Key Outcomes:
-
Improved drilling efficiency and safety
-
Reduced non-productive time (NPT)
-
Lower equipment wear and energy consumption
3. Production Optimisation and Well Performance
Agent Role: Production Optimisation Agent
Function:
-
Use reservoir simulation data to manage production flow rates
-
Recommend artificial lift adjustments or gas injection strategies
-
Monitor for hydrate formation, scaling, and wax deposition
Key Outcomes:
-
Maximised hydrocarbon recovery
-
Lower production losses
-
Better reservoir management
4. Asset Monitoring and Predictive Maintenance
Agent Role: Asset Health Monitoring Agent
Function:
-
Detect anomalies in rotating equipment, pipelines, and compressors using AI-powered diagnostics
-
Integrate data from SCADA, Iot sensors, and maintenance logs
-
Schedule and prioritise maintenance tasks autonomously
Key Outcomes:
-
Minimised unplanned downtime
-
Extended asset lifespan
-
Lower operational risk
5. Energy Trading and Price Forecasting
Agent Role: Energy Market Intelligence Agent
Function:
-
Analyse global oil price trends, market sentiment, and geopolitical risks
-
Recommend hedging strategies based on demand-supply patterns
-
Support decision-making in energy contract negotiations
Key Outcomes:
-
Improved risk management
-
Higher margins on traded volumes
-
Enhanced agility in energy portfolio management
Integration Across the Value Chain
Agentic AI doesn’t function in silos. Its power connects upstream, midstream, and downstream operations through agent collaboration.
Upstream Integration: Agents collaborate across seismic data interpretation, drilling, and production analytics, enabling a seamless digital oilfield. Midstream Optimisation: Agents manage logistics, pipeline monitoring, and environmental compliance, minimising transport losses and regulatory risks. Downstream Intelligence: Agents forecast refinery output, optimise blending operations, and manage customer demand forecasts, ensuring product-market alignment.
Safety and Human-Agent Collaboration
Safety is paramount in high-risk environments like offshore platforms or refineries. Here, agentic systems function not to replace humans but to augment them.
Example Use Case: Emergency Response Planning
-
Agents monitor environmental sensors (gas leaks, pressure build-ups)
-
In real-time, they initiate evacuation protocols, inform safety crews, and optimise escape routes based on current location data
-
Collaborative dashboards allow human supervisors to intervene or override actions, ensuring accountability and trust
This symbiotic interaction ensures critical decisions benefit from human judgment and machine precision.
Environmental Monitoring and ESG Reporting
Sustainability is no longer optional. Agentic AI plays a pivotal role in ensuring compliance and improving environmental impact.
Agent Roles:
-
Emissions Monitoring Agent tracks the plant's CO₂, NOx, and methane emissions.
-
ESG Reporting Agent compiles, analyses, and formats environmental data for regulatory disclosures.
Impact:
-
Real-time environmental footprint tracking
-
Automated reporting for regulatory bodies (e.g., EPA, EU ETS)
-
Support for sustainable development goals (SDGS)
Architecting the Agentic AI System for Oil and Gas
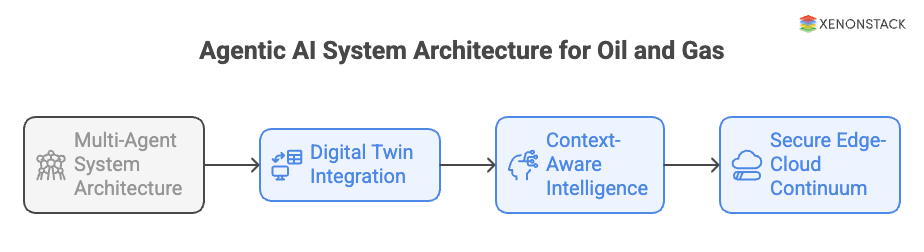
A robust Agentic AI framework for oil and gas includes:
-
Multi-Agent System Architecture
A network of autonomous agents that can communicate, negotiate, and collaborate for task execution across operations. -
Digital Twin Integration
Each physical asset is mirrored by an agent-managed digital twin, enabling simulation, monitoring, and predictive analytics. -
Context-Aware Intelligence
Agents are equipped with contextual data, such as equipment history, environmental conditions, and operational constraints, to enhance decision-making. -
Secure Edge-Cloud Continuum
Critical real-time functions are managed on edge devices, while long-term analytics and planning are centralised in the cloud.
Industry Use Cases and Real-World Applications
Case Study 1: Autonomous Pipeline Monitoring
A leading oil pipeline operator deployed Agentic AI agents across a 1,200-mile network. The system continuously monitored for leaks, temperature fluctuations, and pressure anomalies. Upon detecting signs of leakage, agents rerouted the flow, alerted human operators, and triggered drone inspections.
Result:
-
60% reduction in manual inspections
-
30% reduction in leak response time
-
$5M annual savings in operational costs
Case Study 2: Offshore Rig Optimisation
An offshore production company implemented intelligent drilling and asset maintenance agents. Agents coordinated to reduce energy consumption by optimising engine loads and anticipating maintenance needs.
Result:
-
20% increase in uptime
-
12% reduction in CO₂ emissions
-
Enhanced crew safety through autonomous risk alerts
Ethical Considerations and Governance
While the benefits of Agentic AI are profound, ethical and governance concerns must be addressed:
-
Transparency and Explainability: Agents should provide clear reasoning for decisions, especially in safety-critical environments.
-
Bias and Fairness: Models should be trained on diverse datasets to avoid decision-making bias.
-
Human Oversight: Clear protocols for human-in-the-loop decision-making must be established to prevent autonomous overreach.
Governance frameworks tailored for Agentic AI are essential for maintaining trust, compliance, and accountability.
Future Outlook: The Autonomous Oilfield
The next frontier is the fully autonomous oilfield, where:
-
Agentic systems operate rigs, refineries, and pipelines with minimal human intervention
-
Collaboration between AI agents and human engineers becomes seamless through natural language interfaces
-
Renewable energy integration, carbon capture agents, and ESG agents work in tandem to align with net-zero goals
This shift concerns automation and orchestration—the smart coordination of people, agents, and infrastructure to create a responsive, efficient, and resilient energy system.
Read here about Seasonality Analysis for Power Generation Know more about Generative AI for the Retail Industry
Next Steps with Agentic AI and Agents
Talk to our experts about implementing compound AI system, How Industries and different departments use Agentic Workflows and Decision Intelligence to Become Decision Centric. Utilizes AI to automate and optimize IT support and operations, improving efficiency and responsiveness.