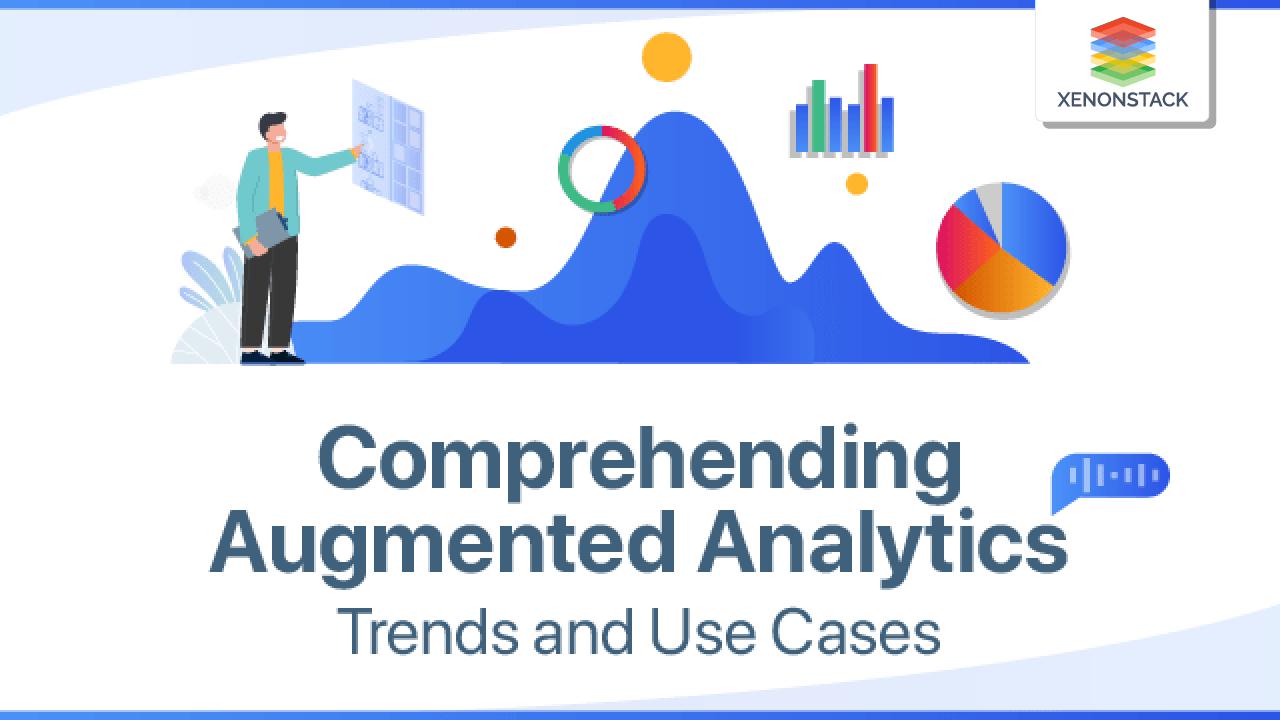
Introduction to Augmented Analytics
With the rising demands and importance of data, data processing tools and advanced-level technologies are more readily available to industries or companies regardless of the organization's size. Nowadays, for many brands or industries, data-driven is a key priority. To remain in competition in the market, industries need to adopt advanced data processing technologies like Augmented Analytics.
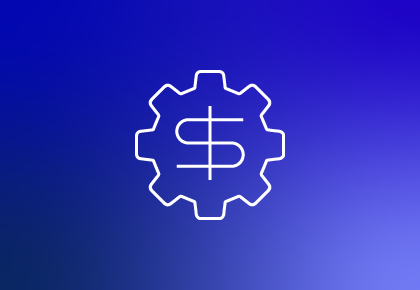
Transformation Impact
90% of business owners believe augmented analytics will transform operations similarly to how the internet did
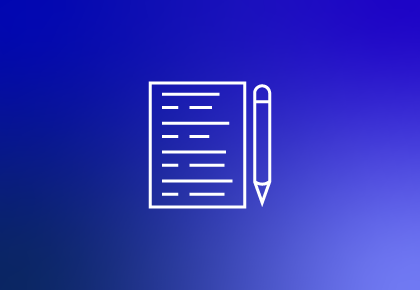
Projected Global Demand
Global demand for augmented analytics is expected to reach $30 billion by the end of 2024, driven by increasing adoption of advanced technologies
Augmented Data Management applies artificial intelligence (AI) to improve or automate data management processes. Taken From Article, Augmented Analytics Benefits and its Future
What is Augmented Analytics?
It facilitate data assessment by using Artificial Intelligence (AI) and machine learning to improve human efforts. As it automates data insights and offers better information, the process is more powerful than conventional analysis methods.
In the banking, financial services, and insurance sectors, Enhanced Analytics is currently being introduced. The worldwide market promotes business understanding of the value of consuming emerging data streams from multiple sources. There is a growing need to improve productivity and democratize analytics and data, and business managers need to make the job stress-free.
What is the workflow of Augmented Analytics?
The workflow is highlighted below:
Prepare Data
- The algorithm detects schemas, profiles, and catalogs and recommends enrichment, data lineage, and metadata.
Finds Pattern in Data
- Natural-Language queries:- Using NLP, users can query their doubts
- The algorithm helps find all relevant patterns in data
- Models are auto-generated according to datasets and user requirements.
Share and Operational Finding
- Insights are narrated in natural language or visualization to focus the user on what is important and actionable.
- Can it be embedded in apps or conversational UI?
Artificial Intelligence mimics human action; therefore, it is lightning the burden of humanity. Big Tech giants are researching the new capabilities of AI. Click to explore about our, Ethics of Artificial Intelligence
What are the technologies involved?
The technologies used for Augmented Analytics are described below:
Big Data
Big data indicates a large amount of structured and unstructured data. Some big data is summarised as 3Vs: Volume, Velocity, and Variety. McKinsey defines datasets whose size is beyond the potential of traditional database software tools to collect, store, manage, and analyze. In this data is stored from various data sources, and big data helps keep and manage data.
Machine Learning
To get the most out of Big Data, machine learning techniques are mostly preferred by the organization. Machine learning is a way of analyzing data that allows computer systems to learn from input data without human interference. With the help of specially programmed algorithms in Big Data tools, Analytics through machine learning systems can continuously adjust to the new data and improve themselves, helping the organization refine its operations and achieve a competitive advantage.
Artificial Intelligence
Artificial Intelligence is another significant technology that goes on par with ML. Artificial Intelligence, abbreviated as AI, is a computer science branch that produces a machine capable of performing tasks similar to humans, such as comprehension of speech and text, understanding, and problem-solving. It can perform complex tasks using AI-driven approaches by analyzing enormous data and recognizing these recurrent patterns.
Natural Language Processing
Natural Language Processing (NLP) technology enables Analytics systems to view and manipulate natural languages as people do. Embedded NLP systems can analyze terms, phrases, sentences, paragraphs, and entire texts using statistical methods and machine learning algorithms, translating knowledge into readable human language and vice versa.
Business Increasingly relies on AI (Artificial Intelligence) to make important decisions and embraces AI in the business workflow. Taken From Article, Challenges and Solutions in AI Adoption
What are the benefits of Augmented Analytics?
The key benefits are:
The Democratization of Data
- It helps everyone if some person is new in this field. Augmented analytics has pre-built models and algorithms so that to do this job, data scientists or IT do not have that much burden on them, and they can work towards creative things more.
- With user-friendly interfaces, these models are out-of-the-box(easy to understand) so that business users and executives can access the tools quickly.
Faster Decision-Making
- It would recommend which datasets should be used in the tests, warn users when upgrading datasets, and suggest new datasets if users do not get the expected results.
- With just one click, all users receive reliable forecasts and projections based on historical data.
Automated Recommendations
- Augmented analytics solutions feature NLP, allowing non-technical users to ask source data-related questions quickly.
- With intelligent suggestions, natural language generation (NLG) then automates converting complex data into text, thus accelerating analytical insights.
- Anyone can quickly discover unseen patterns and forecast trends using automated suggestions for data enrichment and visualization to maximize the time it takes to go from data to insights to decisions.
- NLP technology also helps drive the ability to make sense of vast quantities of data for non-expert users.
Become a Truly Data-Driven Company
- Although companies are rapidly adapting to change, the value of data and business understanding is more important than ever.
- Analytics has become essential in this age of big data to do everything from understanding sales patterns to segmenting customers based on their online habits, "Using data".
Use Case for Augmented Analytics
Use Case are describe below:
Marketers
For in-depth research, planning, and reporting, marketing executives, entrepreneurs, and others who fall under the marketing umbrella mainly depend upon analytics professionals.
XenonStack provides various features to marketers like missing value imputation, target leakage detection, time-series feature generation. Marketing-based professionals can perform their daily work more efficiently through augmented analytics and makes it layman-friendly. It also put the main control under marketing professionals.
Manufacturing
Industries like manufacturing can make more accurate predictions and help enhance performance. It also help analyze various equipment data in real-time and predict when services of an asset are required. XenonStack has automatically generated data insights such as exceptions, segments, links, and predictions.
It provides an embedded AI-powered system in it that helps process and analyze a large amount of company external and internal data, revealing trends, discovering patterns, and forecasting future outcomes. By using analytics, manufacturers can move from repair-or-replace current maintenance models to predict and fix models.
E-Commerce
A few years ago, without human supervision, it was impossible to generate business insights automatically and automatically conduct data analysis. But now, with the help of augmented analytics, it is possible. XenonStack provides features like automatically chooses algorithms, selects features, deploys models, and monitors deployed models to identify when models need to be refreshed proactively. Through informative presentation makes it smart.
Using tables, charts, numbers of descriptions, and other visuals visualizes data related to sales, inventory customers that help e-commerce companies make more data-driven decisions. It creates fully personalized and exclusive shopping experiences. Through NLP, users can query in natural language and receive answers in the same form that makes it more effective and engaging for customers and employees.
Healthcare
To a certain degree, the quality of medical care and patient outcomes rely on medical practitioners' suitability. It fills a crucial gap in providing more people with higher-quality training at a lower cost. Routine analytical activities in a hospital include hospital staffing, operational expenses, patient turnover, waiting times, and overall experience.
Analytics systems offer instant responses to searched questions, along with responses to additional questions workers didn't realize they were asking. Our AI-powered engine offers straightforward insights to discover hidden inefficiencies and key areas to be tackled.
Insurance
By matching all new insurance claims against the profiles of previous fraudulent claims, insurers can now enhance insurance fraud identification through analytics and XenonStack predictive modeling. It will help inspectors of insurance claims become data-driven decision-makers and progress through hundreds of claims from the conventional method of sifting, seeking to identify fraud using 'gut 'feeling.'
It is also possible to use Augmented Analytics to automate alleged fraud cases' flagging for further investigation. Finally, this strategy would sanitize the insurance industry from false claims and provide those who need it the most with insurance services.
Data Scientists
Its platforms like XenonStack gives data scientists and analysts the greatest advantage as they need not run routine analysis and prepare basic reports. It also helps data scientists to solve complex problems and data science projects through advanced AI and machine learning techniques. Data analytics and scientists can move forward in the right direction with it.
Here are some real-world use cases for augmented analytics.
-
Helping first responders navigate dangerous situations
An IEEE grant, Karen Panetta, started a project In 2020 with the Boston Fire Department to use AI to recognize objects, allowing firefighters to find out where the fire is, what is burning, and what is burning before they arrive on the scene , provided firefighters with real-time data on potential hazards. The technology uses data from sensors worn by firefighters to detect objects that can be navigated during a fire. Advanced analytics capabilities instantly interpret, categorize, and contextualize new data as the emergency evolves.
-
Enhancing targeted advertising using image recognition
In 2017, Coca-Cola tested it to promote its Gold Peak iced tea brand. The company used image recognition technology to identify those who posted photos of glasses, teapots, or competitors' tea products, such as Lipton, Snapple, and Honest, on its Facebook, Twitter, and Instagram. They then targeted those users with Coca-Cola ads.
-
Empowering everyday people with information to enhance their safety
Citizen is a mobile app that sent real-time, location-based notifications to its users, founded in 2017. The app uses data from 911 alerts, social media posts, and police scanners to inform users about crime, fires, and natural disasters. It works behind the scenes, interpreting unstructured data from multiple sources and giving users a "newsfeed" about what's happening in their space.
Explainable AI achieves transparency in AI systems, such as a black-box deep learning algorithm explained by Explainable AI. Click to explore about our, Real-Life Ethical Issues of Artificial Intelligence
Traditional BI and Augmented Analytics
In traditional BI, users manually connect data sources and clean data(where might be chances of error occur), which is a very painstaking task. Then they pick data sources that they need to analyze. After selecting data sources, they use the platform to perform analysis, receive insights, reports and share them across the organization through emails, messages, or within the platform if it's sufficiently advanced.
AI systems help ensure that data collection and access to the system are effortless. Once the information is there, the intelligent system allows human users to select the correct datasets based on the associations detected when the data is brought in. "The AI allows the human "run" when it is time for real analysis, but will also suggest analyses that the human may not even know they want as they set up the "drive."The human user can easily share these insights through a broad range of integrated functions once the insights have been gleaned: in-app messaging, mobile apps, chatbots, AI assistants, and more.
What are the best tools?
The best Augmented Analytics tools are listed below:
IBMMicrosoft
Oracle
Pyramid Analytics
Salesforce
Conclusion
Accurate and up-to-date data is more critical than ever in a rapidly changing business environment. Traditional approaches to business intelligence are needed in today's business environment. A new approach, Augmented Analytics, combines human wisdom with artificial intelligence to provide unprecedented insight. Many of the world's leading companies are already using this technology to gain a competitive advantage.
Read here about Predictive Analytics Tools and its Benefits
Know more about Integrating Real Time Analytics with Continuous Intelligence