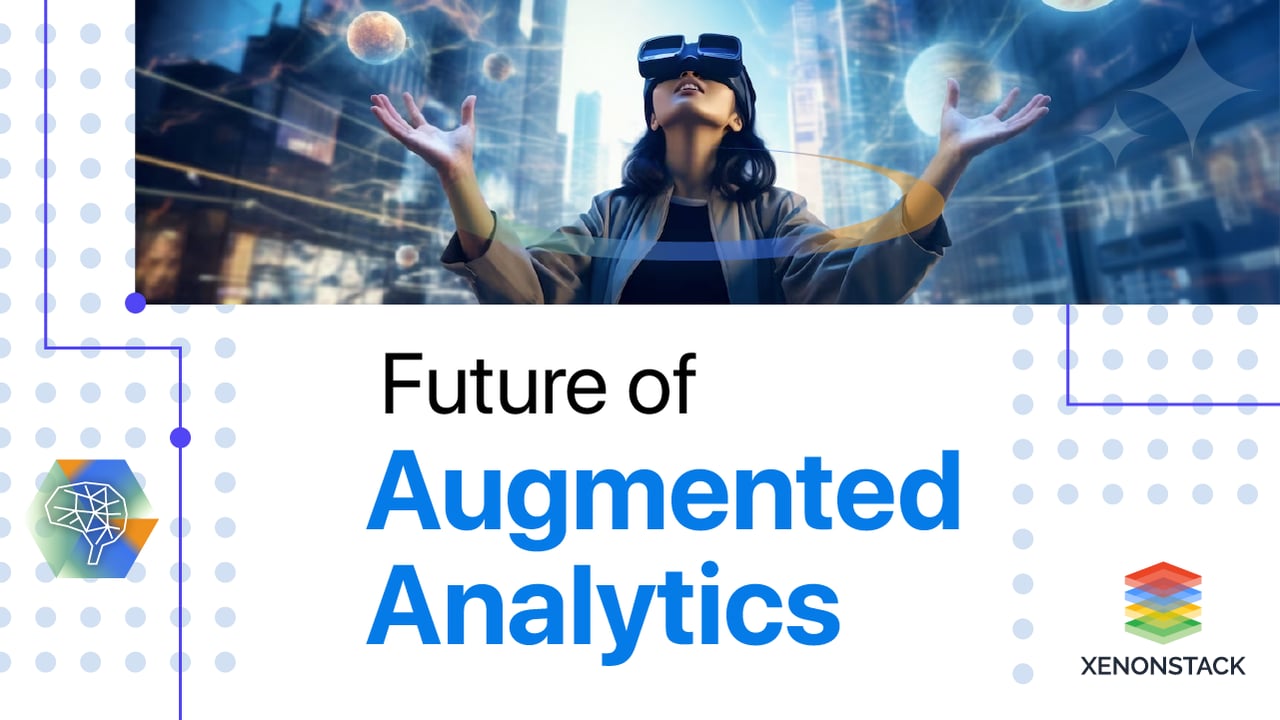
What is Augmented Analytics?
Augmented analytics automates Data Analytics using machine learning and natural language processing. It minimizes the need for specialized skills and makes data exploration accessible to a broader audience. Users possess the capability to streamline tasks like data preparation and produce valuable insights that can result in significant business outcomes.
Augmented analytics offers context-based insights, explanations, and recommendations. It democratizes data-driven decision-making with natural language interfaces, such as chatbots and voice assistants, making analytics more efficient and inclusive.
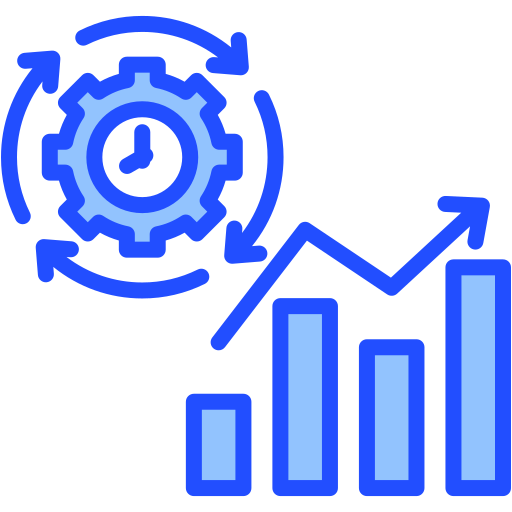
Market Growth and Valuation
In 2023, the global augmented analytics market achieved a significant milestone, reaching a valuation of USD 15.84 billion. Projections suggest a substantial increase, with the market expected to rise from USD 18.68 billion in 2023 to USD 66.54 billion by 2030, reflecting a strong compound annual growth rate (CAGR) of 19.9% during the forecast period
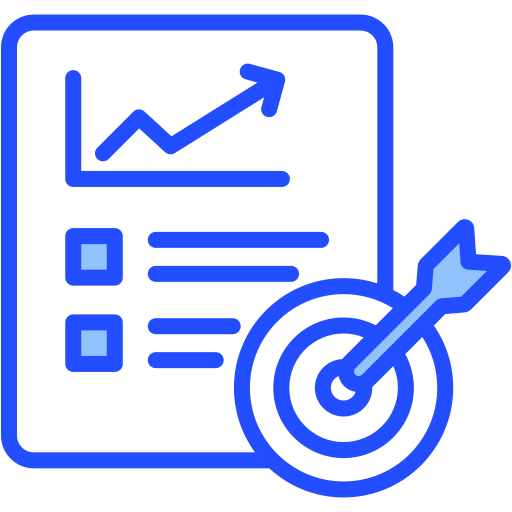
Drivers of Market Expansion
The robust growth of the augmented analytics market is driven by several factors, including the increasing complexity and volume of data, a growing demand for data-driven decision-making, and the need to democratize analytics across organizations of all sizes. These elements are expected to fuel market demand throughout the forecast period
What is the workflow of Augmented Analytics?
The workflow is highlighted below:
Prepare Data
-
The algorithm detects schemas, profiles, and catalogues and recommends enrichment, data lineage, and metadata
Finds Pattern in Data
-
Natural-Language queries: Using NLP, users can query their doubts
-
The algorithm helps find all relevant patterns in the data
-
Models are auto-generated according to datasets and user requirements
Share and Operational Finding
-
Insights are narrated in natural language or visualization to focus the user on what is important and actionable
-
Can it be embedded in apps or conversational UI?
What are the technologies involved?
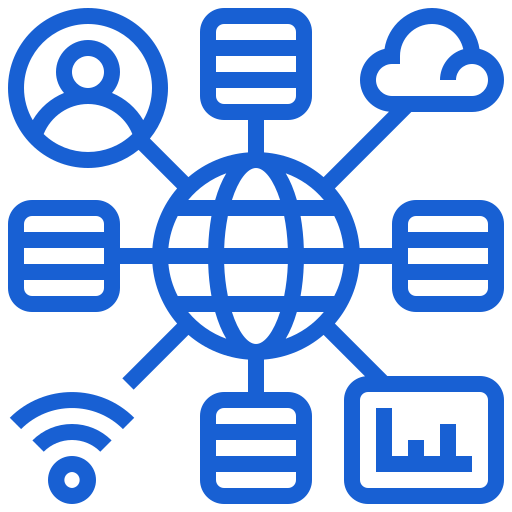
Big Data
Refers to large volumes of structured and unstructured data, characterized by the 3Vs—Volume, Velocity, and Variety. It helps in storing, managing, and analyzing data beyond the capabilities of traditional database tools
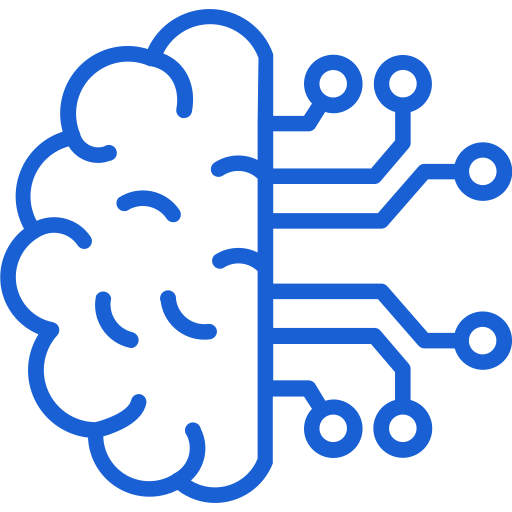
Machine Learning
Used to analyze Big Data, machine learning enables systems to learn from data without human intervention. It continuously adapts to new data, refining operations and providing a competitive edge
.png?width=512&height=512&name=artificial-intelligence%20(3).png)
Artificial Intelligence
AI enable machines to perform tasks similar to humans, such as understanding speech and solving problems, by analyzing large datasets and identifying patterns
.png?width=512&height=512&name=natural-language-processing%20(2).png)
Natural Language Processing
NLP allows analytics systems to interpret and manipulate human language, using statistical methods and machine learning to convert text into actionable insights
Why are Augmented Analytics important?
-
Data as a Key Asset
Recognizing data as a critical asset in the modern economy acknowledges its central role in shaping business strategies and outcomes. However, the vastness and complexity of data pose challenges for human analysis alone.
-
Automation and Enhancement through AI and Machine Learning
AI and machine learning are used in augmented analytics to automate and improve the analytics process, including data collection and generating insights, to handle large amounts of data efficiently.
-
Reducing Dependence on Scarce Data Scientists
Augmented analytics can mitigate the scarcity and cost associated with data scientists. These tools automate mundane tasks, freeing data scientists and empowering other roles to perform better.
-
Faster Discovery of Deeper Insights
In a data-dominated landscape, businesses can secure a competitive advantage by leveraging augmented analytics to derive timely and strategic insights, enabling them to make informed decisions.
The Evolution of Analytics
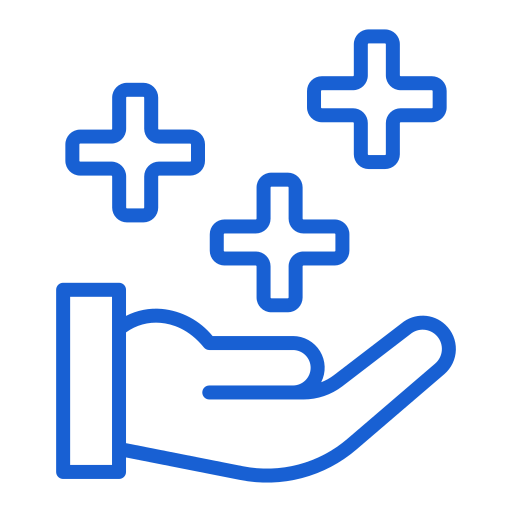
Traditional Analytics
Traditional analytics relies heavily on IT departments or specialists to manage complex data and tools. Users are dependent on experts for data exploration and analysis, and the tools are typically advanced, catering to data professionals. The output of traditional analytics is often large-scale reports covering various metrics and topics
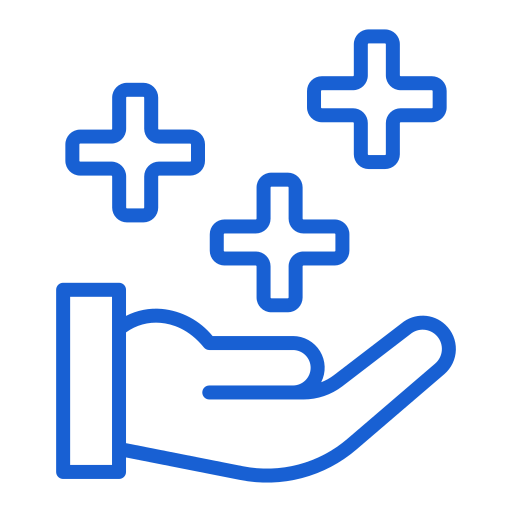
Self-Service Analytics
Self-service analytics empowers business users to take a more active role in the analytics process. With user-friendly tools, individuals can independently perform analytics tasks without needing IT or data experts. The focus is on enabling users to generate insights and make informed, data-driven decisions
Key Components of Augmented Analytics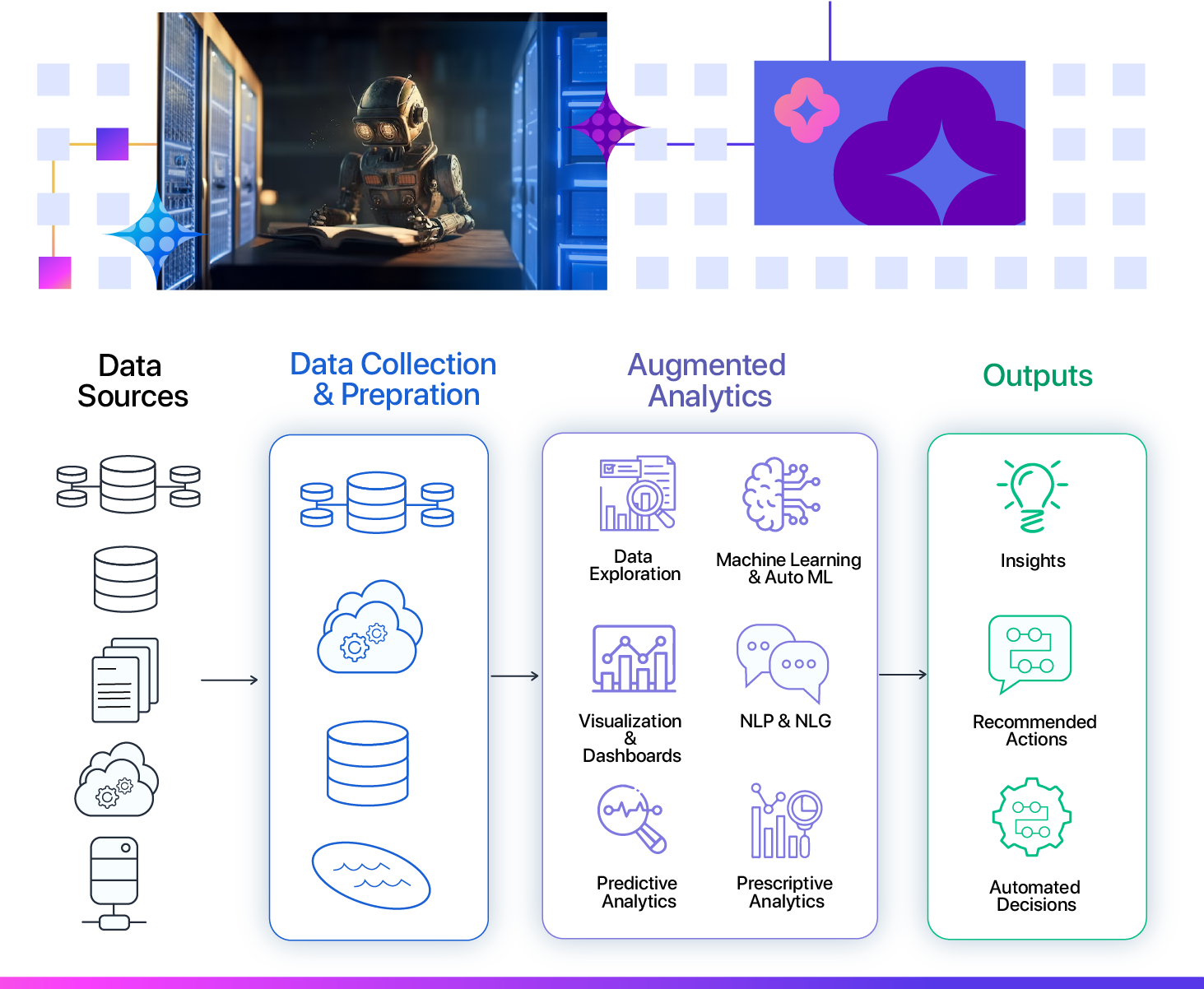
The cloud-based platform of augmented analytics, also known as AI analytics, effectively merges data and analytics. By streamlining data management and empowering various types of analytics, it offers a simplified approach to data analysis.
-
Data Collection and Preparation: AI automates data collection, cleaning, and integration from diverse sources. It handles big data sets, identifies potential errors, and recognizes specific attributes. AI's automated nature ensures rapid and accurate data processing, improving overall efficiency. It minimizes manual effort for routine tasks, allowing data professionals to focus on more complex aspects of analysis and decision-making.
-
Data Exploration and Visualization: Incorporating sophisticated algorithms for analysis and visualization greatly enhances the process of data discovery, mining, and representation. Utilizing artificial intelligence (AI) techniques such as clustering and anomaly detection allows for identifying hidden patterns and anomalies within the data. AI-driven visualization tools enhance data exploration by providing interactive and user-friendly visual displays. These tools allow users to understand data and extract actionable insights comprehensively.
-
Machine Learning and Statistical Analysis: Machine learning (ML) constructs models by analyzing historical data to forecast patterns and make informed choices with limited human involvement. These models are used in AI data analytics applications, such as recommendation systems and search-based analytics in natural language processing. The best-augmented analytics tools are equipped with AutoML capabilities, enabling custom ML models to be created without requiring extensive training.
-
Natural Language Processing (NLP) and Natural Language Generation (NLG): NLP and NLG are crucial tools for data analysis. They help computers and humans communicate effectively, extract critical information from large amounts of text-based data, and perform sentiment analysis to detect emotional tone. With NLG, analytics tools can provide easy-to-understand responses and generate reports in your language. This automated process enhances efficiency and conserves valuable resources, enabling compliance teams to efficiently extract crucial information from organized data. Sophisticated natural language generation (NLG) tools equipped with machine learning capabilities have the ability to provide comprehensive responses to intricate inquiries.
-
Predictive Analytics: AI allows for the development of precise prediction models that handle complex data, nonlinear relationships, and intricate features. Algorithms like ensemble learning and deep neural networks improve the accuracy and reliability of these models even further.
-
Prescriptive Analytics: Prescriptive analytics uses advanced techniques to analyze data and provide specific recommendations for the optimal action or strategy to take in the future. It helps companies optimize inventory levels, pricing strategies, marketing campaigns, or customer service. Based on data and rules, it can also suggest the best actions in other applications, such as CRM or ERP software. It answers the question, "What is the best course of action?" using mathematical models, algorithms, and simulations.
1. Agility: AI-powered augmentation streamlines the search space, presents relevant data, and suggests productive analysis paths. This agility lets users answer data questions faster, freeing time for more strategic tasks.
The Benefit of Augmented Analytics and augmented analytics tools
2. Accuracy: Machine learning and AI technologies ensure accuracy by efficiently handling repetitive tasks. Augmented analytics provides a comprehensive view, reducing the risk of confirmation bias and enabling users to make informed decisions based on thorough analysis.
3. Efficiency: Augmented analytics leverages machine learning and AI to automate specialized, repetitive tasks in data preparation, discovery, and statistical analyses. This automation enhances efficiency, saving users time and energy.
4. Confidence: Augmented analytics tools are designed to be user-friendly, making data analysis more approachable for a broader audience. Integration into business workflows allows seamless exploration of specific questions. The context-aware nature of augmented analytics instills confidence in conclusions, leveraging users' industry expertise
Challenges of augmented analytics tools
Adopting Augmented Analytics is a significant step in transforming the data and analytics market, offering the potential for a competitive advantage. However, several challenges and resistances may impede its integration. Key challenges include:
Adoption Challenges
-
Outcome Expectations: Organizations often expect quick and favourable results from augmented analytics. However, technology has its lifecycle, utilizing AI computation, analytics, and automation techniques. Maturation and improvement in analysis and outcomes require time.
-
"Black Box" Image: Augmented analytics is perceived as a "black box," lacking transparency in decision-making. Without clear explanations, trust in recommendations and insights is hindered, impacting user confidence.
-
Dependency on Legacy Platforms: Organizations may face challenges due to their reliance on legacy analytics platforms, which can act as barriers to adopting augmented analytics.
-
Job Security Concerns: Automation in analytical operations raises concerns about job security as traditional workloads and processes undergo significant revamping.
-
Resistance from Business Leaders: Business leaders may resist adapting to changing scenarios, preferring intuition and traditional decision-making practices over augmented analytics.
-
Misconception of Linear Progression: There's a misconception that augmented analytics maturity follows a linear, progressive path, implemented once a robust foundation is established.
Governance Challenges
-
Need for AI Governance: There's a critical need to emphasize AI and analytics governance and collaboration between analysts and data scientists to support the growing presence of citizen data scientists within business units.
-
Governance for Analytic Content: Leaders must establish rules to govern analytic content and insights generated by augmented analytics. This ensures accuracy and validity and manages bias levels in findings and recommendations.
Use cases for Augmented Analytics
Finance
-
Financial Forecasting: Augmented analytics leverages historical data and external factors to forecast revenue, expenses, cash flow, and profitability. It facilitates scenario and what-if analysis to evaluate diverse outcomes and risks.
-
Financial Reporting: Augmented analytics generates interactive, dynamic reports and dashboards to concisely present financial performance and key metrics. It also offers natural language explanations and narratives.
-
Financial Risk Management: Augmented analytics identifies and monitors financial risks like credit, market, liquidity, and operational risks. It provides alerts and suggestions for mitigating or preventing potential losses.
Human Resource
-
Turnover and Engagement: Identify turnover and engagement factors for informed retention strategies.
-
Talent Acquisition: Analyze resumes, skills, and job fit to find optimal candidates. Automate tasks for streamlined hiring processes. Learning and Development: Use data analytics to assess workforce skills gaps, guiding targeted learning programs.
-
Diversity and Inclusion: Detect and reduce bias in HR decisions with AI, fostering workplace diversity and inclusion.
-
Team member Performance: Utilize data analytics to set SMART goals for employees.
-
Feedback and Recognition: Provide timely, personalized feedback and recognition for enhanced team member performance
Retail
-
Optimize Pricing and Promotions: Utilize augmented analytics to optimize pricing and promotions, considering customer behaviour and demand patterns.
-
Customer Segmentation and Personalization: Segment customers and personalize marketing using data-driven insights from augmented analytics.
-
Demand Forecasting and Inventory Management: Forecast demand, manage inventory, and reduce stockouts with predictive analytics powered by augmented analytics.
-
Fraud Detection and Prevention: Employ machine learning and anomaly detection from augmented analytics to detect and prevent fraud and anomalies.
-
Customer Loyalty and Retention: Increase customer loyalty and retention by leveraging sentiment analysis and natural language processing from augmented analytics.
Health
-
CareForecasting Health Outcomes: Augmented analytics utilizes patient data and AI algorithms to forecast health outcomes, enabling healthcare professionals to tailor treatment plans to individual patient needs.
-
Enhancing Patient Care: Augmented analytics analyses data trends and provides healthcare providers deeper insights into patient health, facilitating better decision-making and proactive care.
-
Predicting Disease Outbreaks: Augmented analytics, which apply data analysis and natural language processing techniques, contribute to predicting disease outbreaks and enhancing clinical trials.
Sales and Marketing
-
Optimizing Pricing Strategies: Augmented analytics analyzes customer behaviour and demand patterns to optimize pricing strategies, increasing profitability and competitiveness.
-
Enhancing Customer Segmentation: Augmented analytics segments customers based on preferences, needs, and potential value, enabling personalized and targeted marketing and sales efforts.
-
Predicting Customer Churn and Retention: Augmented analytics predicts customer churn and retention rates, allowing personalized interventions to retain valuable customers and improve satisfaction.
-
Improving Lead Generation and Qualification: Augmented analytics, employing natural language processing and sentiment analysis, enhances lead generation and qualification for more accurate targeting based on customer sentiments and needs.
-
Measuring Marketing Campaign Effectiveness: Augmented analytics provides insights into measuring marketing campaign effectiveness, allowing for data-driven improvements and strategic adjustments.
Manufacturing
-
Enhanced Logistics and Resource Management: Improves logistics and resource management by estimating shipping timings, production capabilities, and warehouse space availability through data analysis from multiple sources.
-
Proactive Decision-Making with Sensor and Machine Data: Augmented analytics utilizes data from sensors and machines to optimize production processes, enabling proactive decision-making and minimizing downtime.
-
Improved Manufacturing Operations: Quality Control Enhancement: Augmented analytics enhances quality control processes, ensuring products meet specifications.
-
Predictive Maintenance: Predicts maintenance needs, allowing for proactive scheduling and reduced downtime.
-
Optimized Production Schedules: Data-driven insights in production scheduling enhance efficiency and reduce lead times. Margin Increase: Supports cost optimization, resource efficiency, and strategic decision-making, leading to higher margins.
Here are some real-world use cases for augmented analytics
-
Helping first responders navigate dangerous situations
An IEEE grant, Karen Panetta, started a project In 2020 with the Boston Fire Department to use AI to recognize objects, allowing firefighters to find out where the fire is, what is burning, and what is burning before they arrive on the scene, provided firefighters with real-time data on potential hazards. The technology uses data from sensors worn by firefighters to detect objects that can be navigated during a fire. Advanced analytics capabilities instantly interpret, categorize, and contextualize new data as the emergency evolves.
-
Enhancing targeted advertising using image recognition
In 2017, Coca-Cola tested it to promote its Gold Peak iced tea brand. The company used image recognition technology to identify those who posted photos of glasses, teapots, or competitors' tea products, such as Lipton, Snapple, and Honest, on its Facebook, Twitter, and Instagram. They then targeted those users with Coca-Cola ads.
-
Empowering everyday people with information to enhance their safety
Citizen is a mobile app founded in 2017 that sends real-time, location-based notifications to its users. The app uses data from 911 alerts, social media posts, and police scanners to inform users about crime, fires, and natural disasters. It works behind the scenes, interpreting unstructured data from multiple sources and giving users a "newsfeed" about what's happening in their space.
Conclusion
The functionalities of augmented analytics have transformative applications across all sectors, streamlining business operations and improving access to valuable data. Successful adoption requires a holistic approach, addressing challenges in strategy, people, process, data, and technology components.
Read more about Top Real-Time Analytics Use Cases
Learn more about Real-time Analytics: Transforming Data into Instant Insights
Explore more about Augmented Analytics in Healthcare and its Use Cases