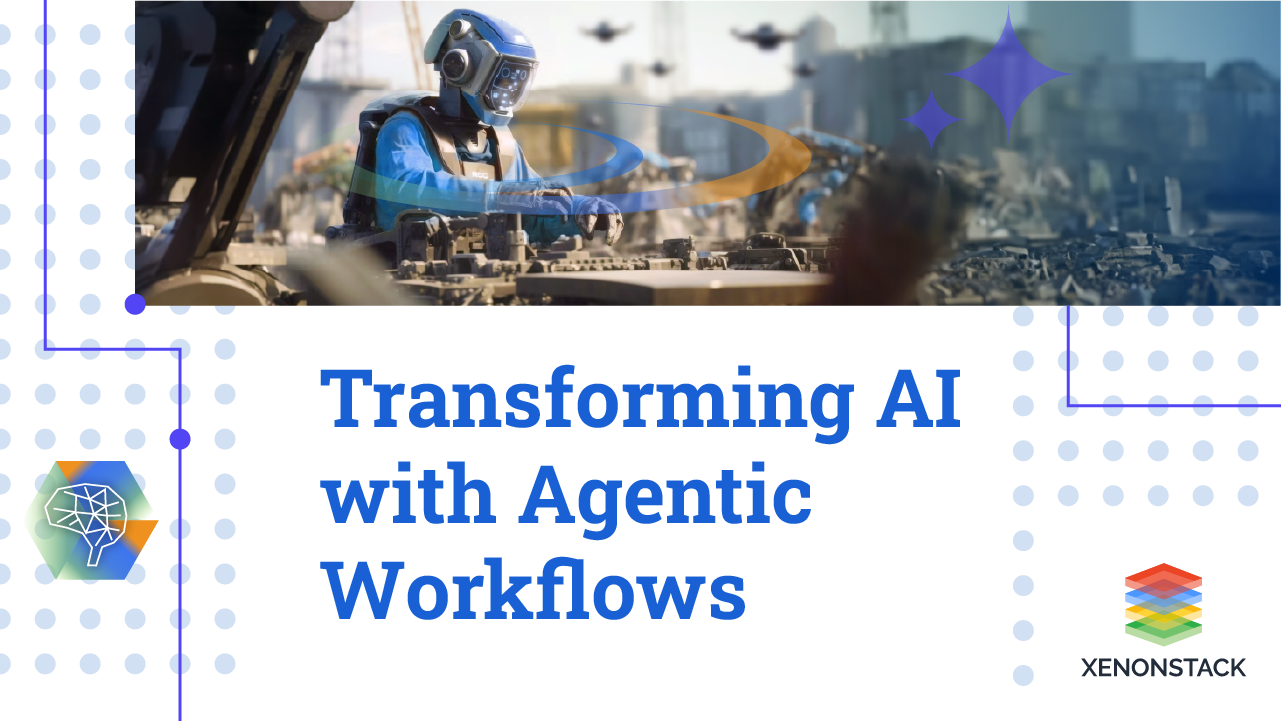
Introduction
Agentic workflows represent not just an evolution but a revolution in artificial intelligence. Imagine a future where technology doesn't just wait for direction but takes the initiative, akin to a trusted advisor or an innovative partner. Unlike their predecessors, these systems don't just react to commands or follow a predetermined path. Instead, they can understand their environment, set their objectives, and take the necessary steps to achieve these goals without needing someone to oversee every move.
This leap from the conventional, reactive AI to the proactive decision-making capabilities of agentic workflows is akin to the difference between a chess piece and a chess master. While the former can only move where it's told, the latter sees the entire board, strategizes, and makes moves based on understanding the game, not just instructions.
In essence, agentic workflows could redefine our interaction with technology, turning our tools into collaborators capable of independently navigating the complexities of their environments to make informed, autonomous decisions. This is not just a step forward in artificial intelligence; it's a giant leap towards a future where technology understands and acts with a level of autonomy that was once thought to be the exclusive domain of human intelligence.
Distinguishing Features from Conventional AI Approaches
-
Autonomy: Agentic workflows are designed to initiate and execute actions independently, surpassing traditional AI systems in self-sufficiency.
-
Reasoning: These workflows have sophisticated decision-making abilities, enabling them to contextually analyze situations, assess alternatives, and strategically plan their actions based on current conditions.
-
Adaptability: Agentic workflows display remarkable flexibility and responsiveness when faced with fluctuating and unpredictable environments. They adeptly adjust their objectives and strategies to align with the prevailing circumstances, setting them apart from the more static traditional AI systems.
-
Language Understanding: With a superior capacity for natural language comprehension, agentic workflows can accurately interpret complex instructions, significantly enhancing their ability to manage intricate tasks.
-
Workflow Optimization: Beyond merely completing tasks, agentic workflows strive for optimization. They do so by strategically establishing subgoals, selecting the most efficient routes, and seamlessly transitioning between subtasks and applications to ensure the most effective execution of processes.
Core Elements of Agentic Workflows
Agentic workflows are powered by AI agents—dynamic problem solvers who evolve and refine their capabilities over time, enabling them to tackle complex and ever-changing tasks. These agents are autonomously driven, making decisions based on their interactions and understanding of the world, thereby playing a pivotal role in task automation and efficiency enhancement within agentic workflows.
Essential Components of Agentic Workflows
AI Agents
At the heart of agentic workflows lie AI agents, sophisticated software programs that leverage artificial intelligence and machine learning algorithms to conduct tasks and engage with users. It is capable of a broad spectrum of functions, from content creation and editing to customer support and medical diagnostics, these agents are continuously trained through advanced machine-learning techniques, enhancing their ability to respond to user queries over time.
Empowering Agents with Appropriate Tools
To augment the capabilities of AI agents, it's crucial to provide them with the necessary tools, integrations, databases, external triggers, and distinct characteristics. This empowerment facilitates the execution of complex workflows, such as streamlining order processes or managing insurance claims, through state-of-the-art software tools, seamless system integrations, and a keen responsiveness to external triggers, ensuring flexible task management.
Continuous Learning and Enhancement
As user interactions increase, AI agents progressively enhance their understanding and task execution capabilities. Each task performed contributes to their growing intelligence, making the learning and improvement aspect a fundamental feature of agentic workflows. This adaptability allows agents to meet evolving requirements and improve performance over time.
Goal-Directed Approach
AI agents are inherently goal-oriented, capable of quickly devising solutions to complex problems. Their rapid information processing enables them to address issues swiftly, showcasing exceptional critical thinking and problem-solving skills.
Intelligent Task Planning
Specializing in the orchestration of workflow automation from planning through to execution, AI agents provide comprehensive support across the board. Their versatility spans many tasks, enabling them to efficiently manage processes ranging from order streamlining to meticulous insurance claim handling.
Execution of Agentic Workflows
Implementing tasks in an "Agentic" manner results in a speed of execution that far surpasses traditional methods, all thanks to the capabilities of an autonomous agent acting as a dynamic force. This method capitalizes on cutting-edge technology to forge a self-reliant entity capable of performing tasks with extraordinary agility and precision.
Integrations, Tools & Triggers
Agents employ a mix of tools, integrations, and external triggers, all supported by Agent Memory, to carry out workflows flawlessly. This strategy combines advanced software tools with seamless system integrations and responsiveness to external triggers, guaranteeing task management adaptability.
Agent Personality
The persona of the agent can be customized to reflect an organization's specific communication style for every scenario. Whether the goal is to convey a friendly, professional, or empathetic tone, the agent's character can be adjusted to blend seamlessly with the team's approach, allowing for the most effective engagement with the intended audience.
The Role of Generative AI Networks in Agentic Workflows
Generative AI networks are at the forefront of enabling autonomous task completion within agentic workflows. These networks, which include generative adversarial networks (GANs), large language models, variational autoencoder models (VAEs), and transformers, are instrumental in generating diverse content types. By mimicking the human brain's learning patterns, these networks can produce new data that closely aligns with the training set, whether it be images or context-based text generation.
In practical scenarios like customer service or content creation, generative AI networks significantly enhance agent efficiency by automating routine tasks like summarizing tickets or calls. This automation not only speeds up response times by providing quick access to conversation summaries but also aids in the onboarding process of new agents by offering training material and suggestions for response phrasing, thereby boosting overall workforce productivity.
Generative AI networks revolutionize agentic workflows through automation, enhanced capabilities, and process acceleration, leading to superior customer service, streamlined operations, and heightened industry efficiency.
Agentic Design Patterns and Self-Improvement
Agentic design patterns emphasize the importance of self-reflection for AI agents. AI agents can identify and rectify errors, inefficiencies, and areas needing clarification by critically analyzing their generated outputs, whether it be code, text, or other forms of answers. This self-analytical approach improves the quality of outputs and fosters a culture of continuous enhancement and learning within AI systems.
Moreover, integrating various tools, such as web search, code execution, and data processing, expands AI agents' capabilities, allowing them to tackle a broader range of tasks with increased precision and efficiency. However, the responsible and secure utilization of these tools is paramount to prevent misuse and ensure the safety of generated outputs.
Advanced Techniques in AI Planning and Collaboration
Advanced planning techniques, including Forward State Space Planning (FSSP) and Backward State Space Planning (BSSP), are utilized by AI agents to navigate complex tasks effectively. These planning methodologies enable AI agents to map out actions leading to goal achievement, enhancing decision-making and problem-solving capabilities.
Furthermore, the concept of multi-agent collaboration underscores the potential of AI systems when agents work in concert. By leveraging diverse capabilities and fostering cooperative control, multi-agent systems can address complex challenges more efficiently than solitary agents, highlighting the importance of teamwork in artificial intelligence.
Addressing Risks and Challenges in Agentic AI Deployment
Implementing agentic AI systems within enterprises introduces several critical risks and challenges that necessitate careful deliberation:
Enhancing Explainability and Transparency
A significant hurdle is the "black box" nature of these AI systems, where users do not easily understand the decision-making process. The intricate algorithms behind these systems often need to be more transparent, complicating efforts to predict or comprehend AI behaviour. This obscurity questions the AI's decision credibility and may obstruct its broader acceptance and audibility. Enterprises must focus on developing agentic AI systems that feature explainable and transparent decision-making processes to foster trust and ensure accountability.
Balancing Oversight and Supervision
The capability of agentic AI systems to autonomously execute dynamically generated workflows could pose challenges across various enterprise scenarios. These systems are likely to necessitate human intervention for creating workflows and, frequently, for their execution and the management of exceptions. Enterprises must find an equilibrium between the autonomy of AI and human oversight to mitigate risks and align with organizational goals.
Navigating Ecosystem Complexity
The intricate web of products and solutions within enterprises means creating and implementing workflows customized to specific ecosystems, which demands specialised training and tools. Agentic AI systems should be crafted to integrate smoothly with the existing enterprise infrastructure and workflows, thereby minimizing disruption and enhancing operational efficiency.
Ensuring Governance and Security
Like any form of automation, agentic AI systems require stringent governance and solid privacy, security, and audit frameworks. Organizations must establish precise guidelines and policies for deploying and using these systems to adhere to regulatory standards and reduce risks, including data breaches, algorithmic bias, and unforeseen outcomes.
Guaranteeing Scalability and Reliability
Assessing the scalability and reliability of agentic AI systems within complex, real-life environments presents considerable challenges. Enterprises must meticulously evaluate system performance across diverse conditions, including unexpected events and interactions with humans and other AI entities. Intensive testing and continuous monitoring are crucial to confirm the system's dependability and safety on a large scale.
By focusing on safety, accountability, and ethical development, enterprises can tap into the transformative power of agentic AI, minimizing risks and ensuring its moral and advantageous deployment.
Real-world applications of agentic workflows
Agentic workflows, a novel approach to AI collaboration, have diverse real-world applications across various sectors. Here are some examples based on the provided sources:
1. HR Management: AI agents in workflow automation can streamline HR processes, such as recruitment, onboarding, and performance management, enhancing efficiency and accuracy in these tasks.
2. Project Management: Agentic workflows can be utilized in project management to automate task allocation, progress tracking, and resource management based on team members' skills and availability, improving overall project efficiency.
3. Business Management: AI agents can revolutionize business operations by automating tasks like customer service, sales, marketing, and financial management. They can analyze data, optimize processes, and provide personalized recommendations, enhancing productivity and customer experience.
4. Customer Support: AI agents can be deployed in customer support to handle inquiries, provide personalized recommendations, and escalate complex issues to human agents when necessary, ensuring 24/7 support and improved service standards.
5. Finance: In the finance sector, AI agents can automate tasks like data analysis, decision-making, and risk assessment with high reliability and precision, minimizing errors and optimizing financial processes.
6. Supply Chain Automation: Agentic workflows can enhance supply chain automation by enabling real-time responses to changes, adaptive learning, and specific goal achievement, leading to efficient and streamlined supply chain operations.
7. Document Management: AI agents can be integrated into document management systems to automate tasks like data extraction, classification, and organization, improving document handling efficiency and accuracy.
8. Order Management: In order management, AI agents can automate order processing, tracking, and fulfilment, ensuring timely and accurate order management processes.
Potential challenges or limitations of implementing agentic workflows
There are several potential challenges and constraints to implementing agentic workflows with AI agents:
1. Complexity and Overhead: Agentic workflows introduce additional complexity compared to traditional single-prompt interactions with AI models. Orchestrating the interactions between multiple agents, managing memory streams, and implementing self-reflection mechanisms adds overhead that must be carefully designed and optimized.
2. Alignment and Coherence: Ensuring that multiple agents working together maintain alignment with the overall goal and produce coherent outputs can be challenging. Inconsistencies or contradictions between agents' contributions must be identified and resolved.
3. Scalability and Performance: As the number of agents and the complexity of tasks increase, scalability and performance can become issues. Efficient management of computational resources and parallelization of agent workflows are necessary to handle large-scale applications.
4. Interpretability and Explainability: The iterative and collaborative nature of agentic workflows can make it more challenging to interpret and explain the reasoning behind the agents' decisions and outputs. Transparency and explainability are crucial for building trust and accountability in AI systems.
5. Robustness and Reliability: Agentic workflows rely on the robustness and reliability of the underlying AI models and tools. Errors or biases in the models can propagate and amplify through the workflow, leading to suboptimal or incorrect results.
6. Ethical Considerations: As AI agents become more autonomous and capable, there are increasing concerns about their alignment with human values and the potential for unintended consequences. Ethical principles and guidelines are considered carefully when designing and deploying agentic workflows.
7. Data and Privacy: Agentic workflows may involve the processing and exchange of sensitive data, raising concerns about data privacy and security. Appropriate measures must be taken to protect data and ensure compliance with relevant regulations.
Impact of agentic workflows on traditional business processes and operations
Intelligent workflows powered by AI and automation are transforming traditional business processes and operations in several keyways:
1. Increased efficiency and productivity: Intelligent workflows automate repetitive, mundane tasks, allowing employees to focus on higher-value, strategic work. This leads to faster process completion and improves overall productivity.
2. Adaptability to changing conditions: Unlike rigid, predefined traditional workflows, intelligent workflows can adapt to evolving circumstances by learning from data patterns and optimizing processes on the fly. This agility is crucial in today's fast-paced business environment.
3. Enhanced decision-making: Intelligent workflows provide critical data insights to employees at crucial moments, enhancing their decision-making capabilities. AI-powered recommendations and automated decisions further optimize processes.
4. Reduced errors and improved consistency: Intelligent workflows maintain process consistency, eliminating mistakes that can occur with manual, repetitive tasks. This leads to higher-quality outputs and better customer experiences.
5. Streamlined integration with legacy systems: While implementing intelligent workflows presents integration challenges, solutions that seamlessly connect with existing infrastructure help businesses get more value from their data. This allows for a gradual, pragmatic transition.
6. Empowerment of non-technical employees: Intelligent workflows enable non-technical staff to implement advanced automation, boosting their confidence and ability to work efficiently. This democratization of automation is a significant shift from traditional IT-driven process improvement.
Conclusion
Agentic AI is poised to revolutionize enterprise operations by enhancing efficiency, decision-making, and adaptability, ultimately reshaping how organizations operate. Integrating AI agents into enterprise systems brings benefits such as increased efficiency, improved decision-making, scalability, and adaptability to changing business needs. This transformative impact is driving a shift in the future of work, particularly in HR processes, where agentic AI streamlines workplace processes and provides predictive analytics. The potential of agentic workflows to advance AI progress is significant, encouraging further exploration and research in this dynamic and evolving field.
-
Click to know about Robotic Process Automation in Cybersecurity
-
Explore the Machine Learning Trends for Businesses