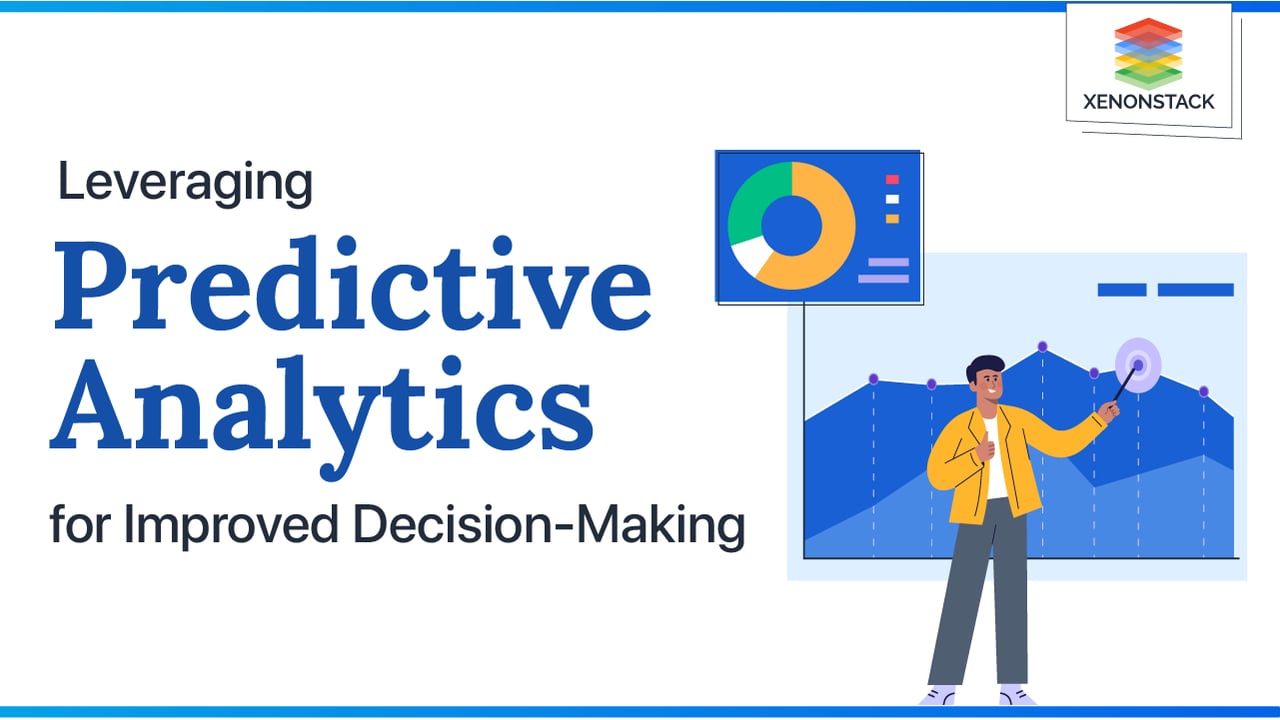
Introduction to Predictive Analytics
Technological advancements have made collecting and storing data more accessible for businesses. The challenge now lies in effectively analyzing the data to gain meaningful insights and make informed decisions. This is where predictive analytics comes into play.
Predictive analytics leverages technology and analytical techniques to extract insights from data and generate predictive models. These models allow businesses to forecast future outcomes, identify patterns, and make data-driven decisions.
Because technology has advanced, it is now possible to evaluate various scenarios. Technological pillars such as futurism, artificial intelligence, data science, and machine learning can assist in understanding the past and constructing possibilities for the future when utilized by skilled professionals.
Neither predictive analytics nor forecasting the future are magical. Data is used to make predictions about possible future scenarios in predictive analytics. It is a method for mapping probabilities based on what has already occurred, using the present and past to project the future.
The sub-branch of Advanced Analytics, the key area of Data Science that uses Statistical concepts to output an event's maximum likelihood. Taken From Article, Predictive Analytics Tools and its Benefits
Why do we need Predictive Analytics in Decision-Making?
Predictive analytics is crucial in decision-making by providing valuable insights and predictions about future outcomes.
Improved Accuracy and Confidence
Predictive analytics leverages advanced algorithms and statistical models to analyze historical data and make predictions. By incorporating data-driven insights, decision-makers can make more accurate and confident decisions than relying solely on intuition or experience.
Anticipating Trends and Patterns
Predictive analytics enables organizations to identify trends, patterns, and relationships in their data. By analyzing historical and real-time data, businesses can gain insights into customer behavior, market dynamics, and other relevant factors. This helps decision-makers anticipate future trends, identify emerging opportunities, and proactively respond to changing market conditions.
Risk Mitigation and Opportunity Identification
Forecasting helps organizations identify and mitigate risk. Businesses can identify risks and impacts by analyzing historical data and using predictive models. This enables them to develop strategies to mitigate risks, allocate resources efficiently, and mitigate adverse impacts. In addition, forecasting helps uncover new opportunities for growth and innovation, allowing business organizations to capitalize on business opportunities and stay ahead of the competition.
Enhanced Resource Allocation
Predictive analytics assists in optimizing resource allocation. Organizations can optimize inventory levels, production planning, and supply chain management by analyzing historical data and predicting future demand. This ensures that resources are allocated efficiently, reducing costs and improving overall operational efficiency.
Customer Segmentation and Personalization
Predictive analytics enables organizations to segment their customer base and personalize their offerings. Businesses can identify distinct customer segments by analyzing customer data and understanding their preferences, behaviors, and needs. This allows for targeted marketing campaigns, personalized product recommendations, and tailored customer experiences, leading to higher customer satisfaction and loyalty.
Strategic Decision-Making
Predictive analytics provides insights that support strategic decision-making. Organizations can make more informed strategic choices by evaluating different scenarios and assessing the potential outcomes of various decisions. This includes market expansion, product development, pricing strategies, and resource allocation decisions. Predictive analytics empowers decision-makers to make data-driven decisions aligned with organizational goals.
Continuous Improvement
Predictive analytics facilitates a culture of continuous improvement within organizations. By monitoring and analyzing key performance indicators (KPIs), organizations can identify areas for improvement, measure the impact of interventions, and make data-driven adjustments. This iterative process helps organizations refine their decision-making processes and enhance overall performance.
Role of Predictive Analytics in improving Decision-Making
Predictive analytics uses advanced algorithms and techniques, including machine learning, to analyze past and present data and make more accurate predictions. By understanding how certain events will affect the future, businesses can make informed decisions that often improve performance.
For the inexperienced, it is helpful to think of estimation as an extension of the traditional process. Rather than relying on hunches or hunches that can be unreliable, predictive models allow decision-makers to validate their assumptions using historical evidence and complex statistical methods.
Predictive models act as consultants to assist organizations in difficult situations where they need reliable guidance but may need access to experts, professionals, or businesses.
Additionally, using predictive analytics makes the decision-making process more accurate and consistent. This results in higher efficiency and long-term cost savings. By using predictive analytics, organizations can reduce uncertainty about business and customer behavior and enable them to make strategic decisions confidently.
Predictive maintenance is critical in diverse application areas, such as manufacturing industry, information, and technology. Taken From Article, Predictive Maintenance Services and Solutions
How does Predictive Analytics work?
Predictive analytics is a sophisticated process that utilizes data, algorithms, and technology to analyze past trends and behaviors to make predictions. This powerful decision-making tool has found successful applications across various industries, including SaaS, eCommerce, and finance.
The strength of predictive analytics lies in its ability to accurately forecast events and trends by examining a wide range of data sources, such as customer information and market activity. By harnessing the potential of predictive analytics, businesses can gain valuable insights into customer behavior and strategically adjust their operations accordingly.
Data analysis is at the core of this process. It involves collecting relevant information from multiple sources and feeding it into advanced models that transform raw numbers into meaningful insights for informed decision-making. Predictive models employ algorithms, including machine learning techniques, to generate precise forecasts and uncover hidden patterns within the dataset.
Machine learning algorithms such as Random Forest and Support Vector Machines are specifically designed to analyze large volumes of data efficiently. These models accurately identify correlations between variables than traditional methods, making them valuable for solving problems across different timeframes.
In the SaaS industry, predictive tools have been successfully deployed to monitor user engagement with online products and identify sales opportunities based on past experiences, all while reducing costs. For instance, Amazon utilizes an AI-based recommendation engine to suggest items related to user searches, resulting in higher conversion rates and increased customer satisfaction.
What are the Applications of Predictive Analytics?
Similarly, E-commerce merchants leverage advanced analytical capabilities to implement personalized recommendation systems, delivering superior user experiences and achieving better investment returns than manual forecasting techniques.
In the finance sector, predictive analytics has expanded beyond traditional use in banks. Financial institutions now employ advanced analytic engines to build profitable portfolios even in highly volatile market conditions, enabling them to generate higher investment returns.
How does Predictive Analytics contribute to better Decision-making?
Predictive analytics enhances decision-making by providing organizations with valuable insights and data-driven guidance. Rather than relying on intuition or guesswork, businesses are now leveraging predictive analytics to make more informed and strategic decisions. This approach helps improve efficiency, risk management, and profitability across various areas, such as inventory management, pricing solutions, and talent acquisition.
By employing predictive analysis models, organizations can make faster and more fact-based decisions supported by data. This leads to improved operational efficiency, increased customer satisfaction, and more robust profit and revenue levels. Studies have shown that data-driven organizations that embrace predictive analytics are more likely to acquire and retain customers, resulting in higher profitability.
In today's customer-centric landscape, organizations can access vast amounts of consumer information and data. To stay competitive, businesses must leverage this data to shape their products, solutions, and buying experiences. Strategic utilization of predictive consumer behavior insights has shown significant benefits, with organizations outperforming their peers regarding sales growth margins and gross margins.
For instance, a telecom company can use predictive analytics to reduce customer churn and assess the effectiveness of marketing campaigns. Similarly, an online retailer can analyze market share online by examining new versus returning visitors, bounce rate, and average session duration. Such insights provide valuable information about consumer preferences and help optimize content and channels to maximize impact on key consumer segments.
Managers must embrace two essential perspectives to navigate the rapidly changing data-driven business landscape. Firstly, they must identify high-risk yet rewarding opportunities, such as entering new markets or adopting new business models. Secondly, they should integrate analytics into their core decision-making processes. By incorporating predictive analysis models into their strategy, managers can streamline internal processes, identify emerging consumer trends, monitor risks, and drive continuous improvement. This analytical transformation enables companies to gain a competitive edge and thrive amidst digital disruption.
Enterprises reducing the need of manual decision making, make operational analytics actionable in real-time. Improve cross-department collaboration. Taken From Article, Enterprise Operational Analytics Services
How are Predictive Analytics and Decision-making used in the software-as-a-service (SaaS) industry?
In recent years, predictive analytics and decision-making have gained significant importance in the software-as-a-service (SaaS) industry. These tools enable SaaS companies to extract valuable insights from data, optimize marketing strategies, and anticipate customer needs. SaaS companies can use predictive analytics and decision-making processes to make well-informed decisions that drive competitiveness and customer acquisition and maximize customer lifetime value.
One example of how predictive analytics and decision-making are utilized in the SaaS industry is through customer segmentation. By leveraging predictive analytics, companies can analyze data to segment their customer base into specific groups, such as target markets or customer segments. This segmentation allows companies to personalize the user experience and concentrate their marketing efforts on users more likely to become high-value customers. For instance, a SaaS company can employ predictive analytics to identify customers more inclined to purchase certain products or services. Armed with segmentation data, the company can tailor its marketing efforts to cater to the specific needs of those customer groups.
Predicting user behavior is another compelling application of predictive analytics and decision-making in the SaaS industry. Companies can employ predictive analytics to anticipate user actions and develop models that help determine how users might respond to specific activities or changes. This data enables companies to create personalized campaigns for each user, thus maximizing the effectiveness of their messaging. For instance, an e-commerce SaaS company can utilize predictive analytics to identify which products a customer is likely to purchase and when they are likely to do so. With this information, the company can create targeted campaigns tailored to the customer's buying behavior, increasing the chances of conversion.
What are the steps for implementing predictive analytics for decision-making?
Key steps to implement predictive analytics for decision-making are below:
Understanding the Data
It is crucial to have a clear understanding of the data set being used for predictive analytics. This involves knowing the data's source, collection method, and structure. The predictive model can maintain high accuracy and relevance by establishing reliable data sources.
Model Selection
Choosing the right model is essential for accurate predictions. Instead of using a one-size-fits-all approach, selecting a model that aligns with the specific business needs and data characteristics is essential. This approach ensures optimized results for the predictive model.
Testing, Validation, and Refinement
To ensure the reliability of prediction models, they must be appropriately tested, validated, and improved. Models should be adequately trained and their results evaluated. Testing the model on various datasets and adjusting the results helps improve its performance. Keeping up with new data research methods and trying different tools and techniques can lead to improvements.
Monitoring Model Performance
Continuous monitoring of the model's performance is crucial. Regularly testing it with new data sets and being aware of any shifts in the data are essential practices. As data evolves, the predictive analytics model should be adjusted accordingly. This approach enables organizations to stay informed about trends and seize opportunities.
Ensuring Accuracy
Accuracy is a critical factor in meaningful predictive models. Employing best practices in data modeling and consistently evaluating the model's accuracy and performance are necessary. Cross-validation techniques can be utilized to ensure that the patterns observed in the training data apply to real-world scenarios. Testing different scenarios and analyzing the results helps improve the accuracy of predictions.
Conclusion
Integrating predictive analytics and decision-making is crucial for data scientists to develop effective solutions. By combining these technologies, businesses can achieve higher levels of accuracy and gain deeper insights into historical, current, and future scenarios. Predictive analytics plays a vital role in processing data to enhance decision-making accuracy. It enables organizations to leverage historical data and statistical algorithms to anticipate outcomes and make more informed choices. On the other hand, decision-making processes provide structured approaches to reaching optimal solutions for various problems or situations.