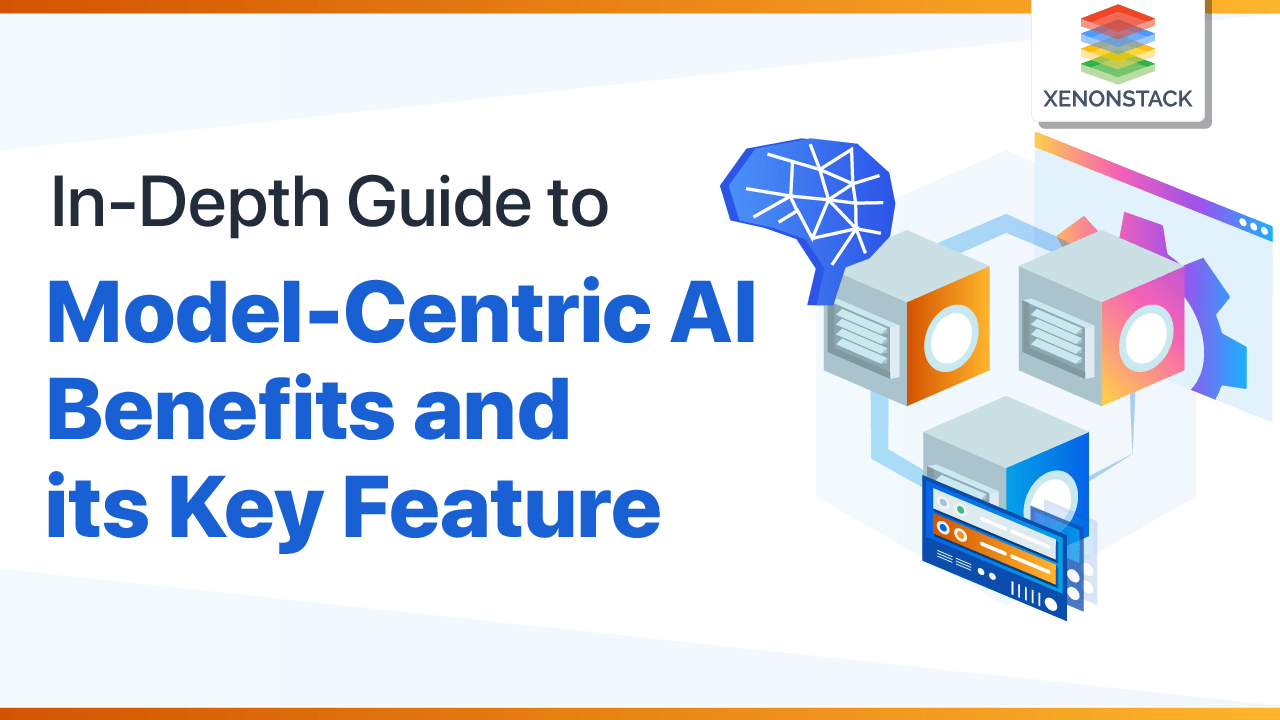
Overview of Model-Centric AI
To achieve a good AI solution, a careful balance between Model-Centric a Data-Centric perspective is fundamental. AI System = (Model Centric AI) Code + Data (Data-Centric AI).
The model-centric approach requires experimental research to increase the performance of the ml model. This involves choosing the appropriate model architecture and training technique from various options. Organizations can maintain their data while improving the code or model design in this manner. The primary goal of this method is to work on code. Currently, most AI applications are model-centric. One possible reason is that the AI industry is paying great attention to academic research on models. More than 90% of the research literature in this field focuses on models. Indeed, it isn't easy to generate large datasets that can become a widely accepted standards. Therefore, the AI community believes that model-focused machine learning holds more promise.
Data-centric Artificial Intelligence can help improve the performance of AI services through extrapolation, augmentation, and interpolation. Click to explore about our, Data-Centric AI
Model-centric Approach to AI
A model-centric approach to AI focuses on creating high-quality models using suitable machine-learning algorithms, programming languages, and AI platforms. This approach has significantly advanced machine learning/deep learning algorithms.
The focus on building powerful models has spawned many AI/machine learning/deep learning frameworks using various programming languages such as Python, R, etc.
These popular frameworks include Python Sklearn, Tensorflow, Pytorch, and others. Besides, almost all cloud service providers are developing AI/ML services focused on building machine learning models.
Key Points
- Improve the model while keeping the data the same.
- Optimize model performance.
- Keep data static through the model development workflow process.
Why is it important?
In ML project life, Model Centric AI can improve the model (or “code”) and the performance rather than the data to make high-quality results through all the stages.
Industries like as:-
- Media and advertising
- Healthcare
- Manufacturing
A manufacturing company with several products cannot employ a single machine learning system to identify production errors across all of its products, in contrast to the media and advertising businesses. Instead, every manufactured product would need a uniquely trained ML model.
While media firms can afford to have a whole ML department working on every little optimization challenge, a manufacturing company that needs several ML solutions cannot fit into such a size template.
AI and data together come with patterns and help to make decisions. Click to explore about our, Ethics of Artificial Intelligence
What are the benefits of Model-Centric AI?
The following are some advantages of this approach:-
- The most common AI challenges may be handled by using methods.
- Improvements in AI technology result from the emphasis on creating better models.
- Organizations with data and wish to apply AI to address business challenges can employ this strategy.
- ML is an iterative process that involves designing empirical tests around a model to improve performance.
- It involves finding the exemplary model architecture and training process in many possibilities to achieve a better solution.
- Model-centric AI collects all the data you can collect and develop a good enough model to handle noise in the data.
- The established process requires keeping the data constant and improving the model over and over until the desired result is achieved.
Difference Between Model-centric AI and Data-centric AI
Model-centric AI and data-centric AI are two different approaches to AI.
- A model-centric approach focuses on creating high-quality machine-learning models using suitable algorithms, programming languages, and AI platforms. This approach has significantly advanced machine learning/deep learning algorithms. In contrast, a data-centric approach to AI is focused on getting the correct data that can be used to build high-quality, powerful machine learning models.
- Unlike model-centric AI, the focus shifts to obtaining high-quality data to train the model rather than the model. There are many different approaches to AI, but a hybrid or balanced approach that employs model-centric and data-centric models is the most effective way to design intelligent machines.
The self-explanation capability of Explainable AI increases accountability. It also enhances the trust of customers and stakeholders. Click to explore about our, Explainable AI in Insurance Industry
What are the key features of model-centric AI?
The key features for model-centric AI is listed below:
Data manipulation is a one-time operation
Traditionally, data science teams download static data from a source. It is then processed, cleaned, and stored in the database. After that, it got little attention because data collection and processing was considered a one-time thing.
Quantity of data outweighs data quality
This approach dramatically emphasizes the amount of data to make the models work. So even though we have a lot of data to work with, the benefits could be more commensurate with the effort.
Focus on the model
The main focus is on algorithms and models to improve the predictive performance of AI models. Various algorithms are tested, and the hyperparameter is gradually adjusted to achieve the required improvement.
Future Trends
AI systems are made up of code, and data code, which refers to the model created using frameworks in languages like Python, C++, R, etc. All research labs worldwide aim to develop a model architecture that would perform better and advance the state-of-the-art for a specific dataset, such as the COCO dataset. It is known as a "model-centric strategy" to maintain the data constantly while tweaking the model's settings to enhance performance.
This is beneficial for ML engineers to access updated and improved models efficiently and to develop the best model possible for ML projects. The unique feature of this period is that data gathering was a one-time effort carried out at the beginning of the project to grow the dataset over time but with little consideration of its internal quality. The concept was developed for small-scale deployments when a single server or device could manage the whole load, and monitoring wasn't an issue. The main challenge was that everything had to be done manually, including data cleaning, model training, validation, deployment, storage, and sharing.
Conclusion
A data-centric approach to AI aims to collect the correct kind of data that can be utilized to create machine learning models of the highest magnitude and performance. This approach is expensive as it requires a lot of data to train the models. In contrast, in model-centric AI, the emphasis now turns to obtaining high-quality data for training models.
- Discover more about Artificial Intelligence (AI) Solutions on Edge
- Explore here about Responsible Artificial Intelligence in Government