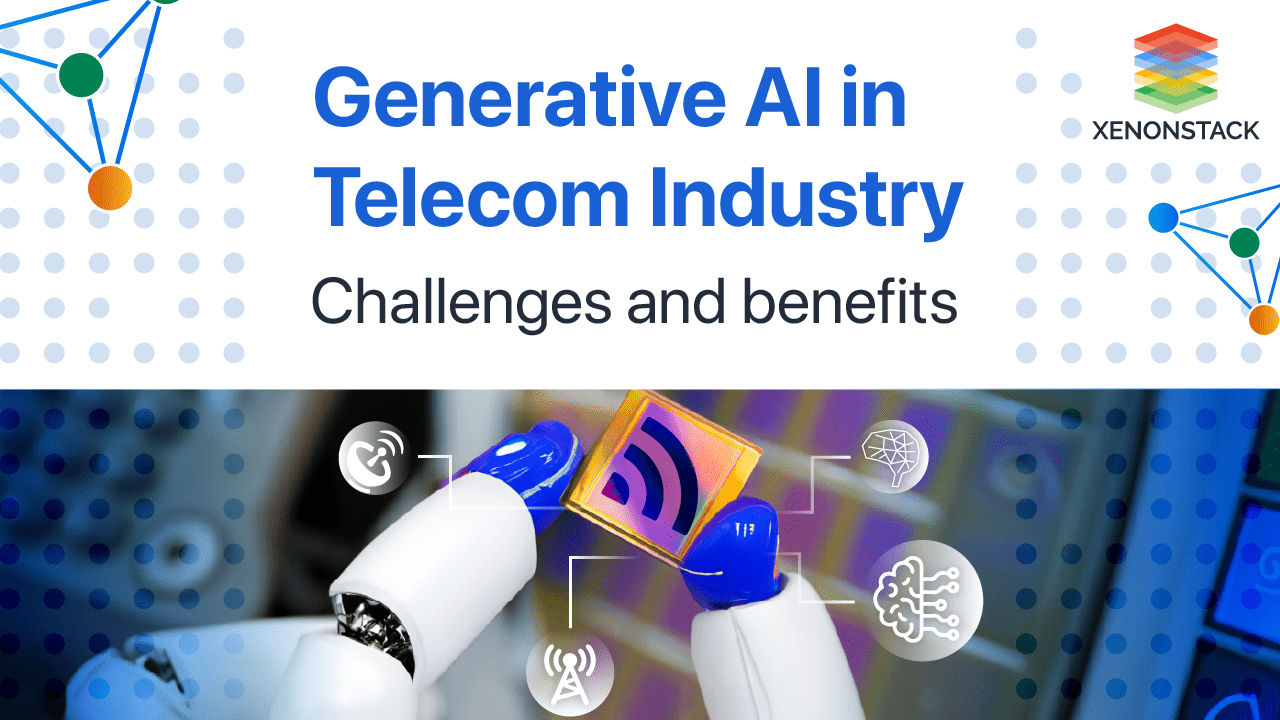
Introduction to Generative AI in Telecom
The market for generative artificial intelligence in the telecommunications sector, which amounted to 150.81 million US dollars in 2022, is projected to reach USD 4,883.78 million by 2032 at an annual growth rate of 41.59% from 2023 to 2032.
The telecommunications industry has experienced a rapid transformation in recent years, and the advent of generative AI is set to bring about even more dramatic changes.
Generative AI is an advanced technology with the potential to transform telecom companies' operations, customer interactions, and service offerings.Through the implementation of generative AI techniques, telecom companies can harness the power of innovation, optimize their network infrastructures, and enhance their operations and customer experiences.This blog post explores generative AI, its functionality, and its potential uses in the telecom industry.
Applications of Generative AI in Telecom
Generative AI transforms the telecommunications industry by revolutionising operations, customer engagement, and service optimisation. Here are the key benefits of using generative AI in telecom:
1. Network Security and Fraud Mitigation
Description: A proactive and robust approach to safeguarding network integrity and mitigating fraudulent activities for seamless and secure connectivity.
Issue/Opportunity: In the ever-evolving landscape of digital connectivity, a proactive and robust approach to safeguarding network integrity and mitigating fraudulent activities is imperative for ensuring seamless and secure connectivity. As networks become increasingly complex and interconnected, the potential vulnerabilities and risks also escalate. Hackers and malicious actors continuously devise sophisticated strategies to exploit weaknesses, threatening sensitive data confidentiality, integrity, and availability.
-
Proactive Prevention Utilizing predictive analysis, Generative AI assists organizations in foreseeing and proactively mitigating security risks and patterns of fraud.This proactive strategy reduces the chances of security breaches, ensuring that users have a digital experience that is both secure and reliable.
-
Adaptive Security Measure Hackers constantly evolve their tactics, posing challenges to traditional security systems. Generative AI's dynamic adaptation capabilities allow security measures to stay ahead of emerging threats. This ensures ongoing protection for end-users and their data.
-
Fraud Prevention Generative AI is used to create realistic fraud scenarios that support the creation of strong fraud detection systems by utilising past fraud data.This protects end-users from falling victim to various fraudulent activities in the digital space.
-
Enhanced Threat Detection Generative AI excels in identifying patterns and anomalies in network behaviour, enabling early detection of potential security threats and fraudulent activities. This leads to a more secure environment, safeguarding sensitive information and ensuring user data protection.
-
Reliable System Testing Generative AI enables the creation of synthetic datasets to thoroughly test security systems. This ensures that security measures are reliable and well-prepared to handle diverse scenarios, contributing to a more resilient and dependable digital infrastructure for end-users.
-
Maintained Data Privacy Generative AI facilitates the generation of synthetic datasets for testing without compromising real user data. This ensures that the testing processes are conducted in a privacy-preserving manner, aligning with ethical standards and providing users with confidence in the security of their information.
2. Enhanced customer engagement
Description: Implementation of advanced technologies and customer-centric practices to manage relationships effectively.
Issue/opportunity: Telecom companies use customer data to create personalized and adaptive experiences. This helps them identify and solve problems before they become more significant issues. Reliability and quality of service are key components that telecom firms may deliver while optimising earnings.
-
Customer Journey Personalization: Generative AI analyses vast datasets to understand individual customer preferences, behaviours, and needs. This information is then utilized to personalize the customer journey, offering tailored services and experiences.
-
Predictive Analytics: By employing generative models, telecom companies can predict potential issues or service disruptions before they occur. This proactive approach allows for pre-emptive problem-solving, reducing downtime and customer frustration.
-
Dynamic Pricing Optimization: Generative AI is able to dynamically optimise pricing strategies by analysing market conditions and client usage trends. Customers can rest easy knowing that their price will be reasonable, competitive, and customised to meet their unique demands and usage habits thanks to this guarantee.
-
Intelligent Chatbots: AI-powered chatbots that are powered by generative models offer individualised, real-time support to clients. These chatbots provide instant solutions, understand natural language, and improve customer service in general.
-
Feedback Analysis: Generative AI is used to analyze customer feedback, reviews, and sentiments. Telecommunication companies can utilize this tool to gain insights into customer satisfaction levels, pinpoint areas that require improvement, and proactively make decisions to elevate the overall quality of their services.
-
Multi-dimensional Insights: Generative AI processes diverse data sources, providing telecom companies with multi-dimensional insights into customer behaviours and preferences. This comprehensive understanding enables targeted marketing efforts and the development of customer-centric services.
-
Service Quality Optimization: Through continuous monitoring and analysis, generative AI contributes to optimizing service quality. It helps identify and address network issues, ensuring customers experience reliable, high-performance telecom services.
3. Automated Monitoring Solutions
Description: Leveraging advanced technologies, including Gen AI, for continuously monitoring and managing various network components.
Issue/Opportunity: Automated Monitoring Solutions in the telecom sector require real-time performance monitoring, fault detection and diagnostics, security monitoring, scalability, predictive analytics, automation of routine tasks, interoperability, customizable alerts and reporting, compliance and reporting, and a user-friendly interface.These ensure efficient, reliable, and proactive network management, allowing optimal service delivery and minimizing disruptions.
-
Synthetic Data Generation: Generative AI can create synthetic datasets that mimic real-world scenarios, providing diverse data for testing and training automated monitoring systems.
-
Signal Enhancement and Noise Reduction: Generative AI models can be trained for recognizing and filter out noise from signals, improving the quality of data transmissions and aiding in more accurate monitoring.
-
Predictive Analytics: In order to anticipate potential problems and to proactively address them, preventing interruptions and ensuring continuous network monitoring, predictive analytics can be used.
-
Anomaly Detection: Generative AI models can learn normal network behaviour and generate predictions for expected performance. When anomalies occur, the AI can quickly identify and alert operators for prompt resolution.
-
Content Generation for Reports: Automated monitoring often involves generating reports and insights. Generative AI assists in creating detailed and comprehensible reports, providing valuable insights for network management.
-
Continuous Learning and Adaptation: Gen AI models can constantly acquire knowledge from fresh data, adjust to evolving network circumstances, and enhance the precision of monitoring forecasts as time progresses.
4. Manage Dynamic Networks - Energy Infrastructure Optimization
Description: Intelligent AI systems are leveraged to optimize energy infrastructure in dynamic networks, improving performance and resource utilization.
Issue/Opportunity: Energy Infrastructure Optimization (EIO) is a set of technologies and strategies designed to improve energy consumption, improve energy infrastructure reliability and reduce environmental impacts in the telecommunications industry. This includes intelligent energy management (IEM) systems, energy efficient network equipment and real time energy consumption monitoring and optimization via advanced analytics. To do that, intelligent energy management systems, energy efficiency network equipment and Realtime Energy Use Monitoring and Optimisation Through Advanced Analytics will be needed. The goal is to balance network performance, operational efficiency, and environmental sustainability.
-
Churn Prediction: Generative AI can predict potential energy infrastructure issues and proactively address them, minimizing downtime and disruptions.
-
Hyper-Personalization: AI helps tailor energy optimization strategies to specific network conditions and requirements by understanding usage patterns and demand variations.
-
New Product and Service Analytics: Generative models assist in identifying opportunities for introducing new energy-efficient products and services that align with sustainability goals.
-
Intelligent Chatbots: AI-driven chatbots can provide real-time information on energy consumption, network status, and optimization measures, improving user engagement and satisfaction.
-
Customer Retention: Analyzing data with gen AI helps to identify areas where energy infrastructure improvements can enhance customer experience, leading to better retention rates.
-
Advanced Analytics: Generative AI facilitates advanced analytics for ongoing monitoring, identifying trends, and optimizing energy usage in response to changing network dynamics.
5. Improve Network Performance
Description: Telecommunications network performance is measured by metrics and parameters that evaluate its quality, reliability, and responsiveness in delivering services to users.
Issue/Opportunity: Network Performance management faces challenges such as congestion, scalability, quality of experience, security, technological evolution, dynamic traffic patterns, legacy infrastructure, interoperability, remote network management, and data privacy compliance. These challenges arise due to the increasing complexity and demands of modern telecommunications networks, including the proliferation of IoT devices and 5G networks and the need to ensure backward compatibility while integrating new technologies.
-
Predictive Analytics: By predicting congestion, pinpointing vulnerable areas, and foreseeing equipment failures, operators can take proactive measures to address issues and enhance overall network efficiency
-
Automated Troubleshooting: In order to identify patterns associated with common network problems, genetic AI can be trained. This enables automated troubleshooting, where the AI system can suggest or implement solutions for issues such as connectivity disruptions, signal degradation, or equipment malfunctions.
-
Dynamic Network Optimization: Genetic AI models can dynamically adapt to evolving network conditions in real-time, optimizing parameters by tasks such as adjusting route configurations, allocating bandwidth on demand, and dynamically optimizing network resources. This ensures the achievement of maximum performance.
-
Security Threat Detection: Intelligent artificial intelligence plays a crucial role in enhancing network security by identifying anomalies that may signal security threats, unauthorized access, or cyber attacks. Proactive threat detection provided by AI is instrumental in preventing security breaches and safeguarding the integrity of the network.
-
Resource Allocation: Gen AI models can actively analyze the patterns of network use and intelligently allocate resources to meet demand. This includes optimising the distribution of bandwidth, prioritising critical services and ensuring effective use of network infrastructure.
-
Capacity Planning: On the basis of historical data and expected growth, generative AI helps to plan capacity and predict future network requirements. In order to cope with increased demand and avoid congestion and service degradation, this will help telecommunications operators scale up their infrastructure.
-
Fault Prediction and Prevention: By learning from past incidents Generative AI predicts potential network infrastructure problems . This enables the adoption of precautionary measures to avoid interruptions, reduce down time and improve overall network reliability.
-
Network Documentation and Visualization: Generative AI can contribute to creating dynamic network documentation and visual representations. This aids network operators in understanding complex network architectures, identifying bottlenecks, and streamlining decision-making processes.
-
Automation of Repetitive Tasks: In the realm of network management, advanced AI intelligence has the potential to liberate time for engaging in more intricate and strategic activities by automating routine or redundant tasks. This encompasses tasks such as configuration management, regular maintenance, and performance monitoring.
-
Adaptive Learning: The evolution of the network environment allows genetic AI systems to keep learning and adapting This adaptability is necessary in a rapidly evolving telecommunications environment where new technologies, services and user behaviour continue to determine network requirements.
6. Network Orchestration
Description: Automated coordination and management of network elements and services ensures efficient operation by integrating and optimizing resources, services, and processes.
Issue/Opportunity: Telecom networks face several challenges that require effective network orchestration. These challenges include real-time adaptability, legacy system integration, security concerns, service assurance, complexity in hybrid environments, compliance and regulatory requirements, analytics, and a shortage of human resources. Effective network orchestration must address these challenges to maintain optimal performance and ensure a positive user experience.
-
Automated Network Configuration: Generative AI algorithms can analyse network configurations and automatically generate optimized settings. This helps streamline the configuration process, reduces manual errors, and enhances overall network efficiency.
-
Predictive Analytics: Generative models can be trained on historical network performance data to predict potential issues or bottlenecks. Network Orchestration systems proactively optimize resources and prevent service disruptions by foreseeing challenges.
-
Dynamic Resource Allocation: Generative AI enables intelligent algorithms to allocate network resources dynamically based on real-time demands and changing conditions. This ensures optimal resource utilization, minimizes latency and enhances user experience.
-
Security Response Planning: Generative models can simulate various cyber threats and security breaches, helping create robust response plans. This proactive approach enhances network security by preparing for potential vulnerabilities before they are exploited.
-
Service Quality Optimization: Generative AI analyzes patterns of service quality degradation and recommends adjustments to maintain optimal performance. This includes dynamically orchestrating services to meet quality standards and user expectations.
-
Intelligent Network Auto-Discovery: Generative models can assist in automatically discovering and mapping the network topology. This is valuable for managing complex, evolving network architectures, especially when deploying new services or devices.
-
IoT Connectivity Management: In the era of the Internet of Things (IoT), where numerous devices are connected to telecom networks, generative AI can aid in orchestrating seamless connectivity, ensuring efficient communication between devices and the network.
-
Real-Time Network Inventory Management: Gen AI is capable of playing a role in the dynamic maintenance of network inventories that are constantly updated.It continuously updates and manages the status and location of network assets, thereby enabling the effective utilization of resources.
-
Network Operation Center (NOC) Support: Generative models can assist network operators in decision-making by generating insights and recommendations. This support enhances the capabilities of Network Operation Centers to manage and troubleshoot network issues effectively.
- Adaptive Service Orchestration: Generative AI allows for adaptive service orchestration, where services can dynamically adjust based on user demands, environmental factors, or specific events. This flexibility ensures that the network remains responsive and aligned with changing requirements.
Challenges to generative AI adoption in the telecom industry
Generative AI adoption in the telecom industry faces several challenges that need careful consideration for successful implementation:
- Unclear Objectives: Defining clear and measurable objectives for AI initiatives is crucial. Telecom companies may encounter challenges aligning AI efforts with business goals, emphasizing the need for well-defined objectives to measure success.
- Skill Shortage: The successful implementation of generative AI faces a challenge due to the requirement for more proficient AI professionals. To overcome this obstacle, it is crucial to invest in training programs and recruit data science experts, as this will aid in bridging the skill gap within the industry.
- Data Quality and Analysis:Generative AI is intricately dependent on data. Ensuring the quality of data and implementing resilient data analysis practices are imperative for the effective utilization of AI in the telecommunications sector.
- Security Concerns: Ensuring security and privacy, building customer trust, and complying with regulations are crucial for successful AI adoption in telecom.
- Integration Complexity: Integrating generative AI into existing systems can be complex. Adopting a modular approach and ensuring compatibility with current infrastructure can streamline the integration process.
- Pilot Projects and Scalability: Starting with smaller pilot projects allows telecom companies to test the feasibility of generative AI before scaling up. This approach helps mitigate risks and ensures a more effective and controlled adoption process.
- Cultivating Innovation Culture: Fostering an innovation-friendly culture within the organization is crucial. Encouraging employees to adapt to new technologies and fostering an environment conducive to AI adoption can contribute to successful implementation.
Conclusion of How Gen AI Reshaping the Telecom Industry
Generative AI is transforming the telecom industry. It offers a range of applications, including personalized content creation, efficient network optimization, improved customer service, and predictive maintenance enhancement. By partnering with a generative AI development company, telecom businesses can confidently leverage this technology's full potential and navigate the dynamic landscape. Adopting generative AI solutions allows the telecommunications industry to embark on a path of innovation and excellence, reshaping its future prospects.
- Read here about Generative AI Use Cases for DataOps
- Click to explore Cybersecurity Predictions in Telecom