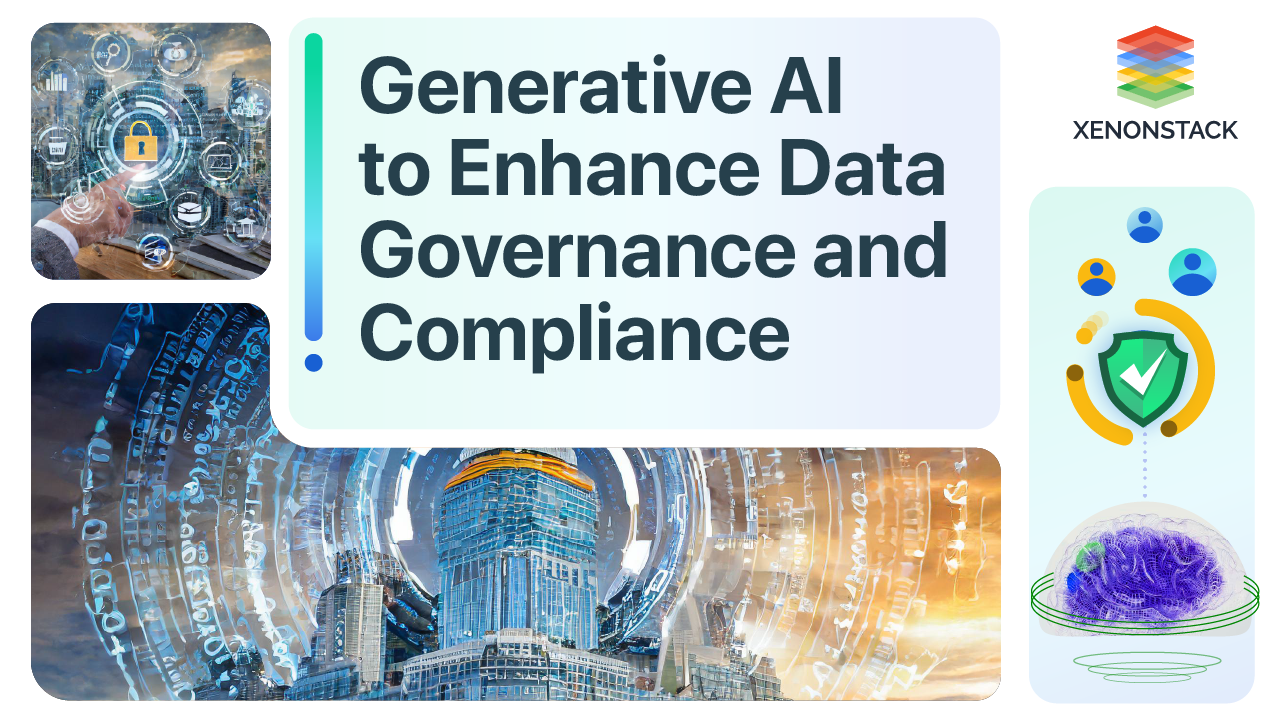
Latest Developments in AI-Powered Data Governance Solutions
The latest developments in AI-powered data governance solutions, as observed in 2023, reflect significant technological advancements and a shift in organizational strategies. These developments are primarily driven by integrating artificial intelligence (AI) and machine learning (ML) into data management processes, focusing on enhancing data quality, security, compliance, and democratization. Let's explore some of these key trends:
1. Automated Data Processing: AI and ML significantly streamline data processing tasks like cleansing and preparation, ensuring data accuracy and improving overall efficiency.
2. Predictive Analytics: Leveraging ML models, organizations can use predictive analytics to make decisions proactively based on anticipated trends or potential risks.
3. Personalized Insights: AI algorithms are adept at providing customized insights tailored to individual user needs and behaviours, enhancing the user experience.
4. Scalable Data Management: ML technologies enable the scaling of data management processes to handle extensive data sets effectively, facilitating real-time analysis and insights.
5. Compliance with Data Privacy Laws: Organizations are required to meticulously adhere to complex data privacy laws like GDPR, CCPA, and HIPAA, which demand frequent policy revisions and thorough risk mitigation measures.
6. Consumer Data Rights: Current regulations mandate that consumers have specific rights over their data, which calls for robust and effective data management practices to ensure compliance.
7. Consistency Across Data Sources: Implementing validation rules and standardizing data formats are essential for maintaining consistency and uniformity across diverse data sources.
8. Data Enrichment and Transformation: These processes augment the value of data, filling in gaps and converting data into readily usable and valuable formats.
9. Data Lineage Visualization: Tools that offer a visual representation of data flow aid in understanding the movement and transformation of data within an organization.
10. Efficient Metadata Management: Automated metadata collection ensures that information is current and accurate while minimizing manual effort.
11. Cloud Computing Benefits: Cloud-based solutions provide scalability and flexibility in data governance without necessitating significant capital investments.
12. Security and Compliance in the Cloud: Many cloud service providers include built-in security features and offer certifications that assist organizations in adhering to various regulatory requirements.
13. Decentralized Data Governance: This approach empowers individual departments, granting them more control and responsibility over their data, thereby enhancing governance at the departmental level.
Potential challenges from using Generative AI in Data Governance
Generative AI, while offering substantial benefits in data governance, also presents unique challenges. Identifying these challenges and proposing solutions is crucial for ensuring effective and responsible use of this technology. Here are some of the critical challenges and potential
1. Data Security and Privacy Risks: Generative AI models often require access to large datasets, including sensitive or personal information. There is a risk of this data being inadvertently exposed or misused. Data anonymization techniques, access controls, and encryption should be implemented before training and using AI models to prevent this.
2. Bias and Fairness: Artificial Intelligence (AI) models can pick up and even intensify biases in the data used to train them, which can result in unjust or unethical outcomes. To prevent this, the datasets used to train AI models should be varied and represent different groups, and the models themselves should undergo regular audits to detect any biases or preferences.
3. Regulatory Compliance: Complying with data protection and privacy regulations like GDPR or HIPAA can be challenging for AI-driven processes. The compliance requirements should be integrated into the AI model development design phase to ensure compliance with laws. Additionally, you should continuously monitor legal changes to stay current with the latest compliance requirements.
4. Data Quality and Integrity: AI-generated data must be validated and monitored to prevent inaccurate information from affecting decision-making processes the accuracy and integrity of the data.
5. Intellectual Property Concerns: Algorithms should screen AI-generated content for potential IP infringements, and AI developers and users should be educated about intellectual property issues to avoid infringement
6. Scalability and Integration: The integration of generative AI into established systems and workflows can present obstacles and financial implications. It's essential to develop scalable and compatible generative AI models that align with existing infrastructure and standards to facilitate this process.
Conclusion
The integration of Gen AI in data governance represents a significant shift towards more dynamic and intelligent data management. Organizations can unlock their full potential by addressing the unique challenges of Gen AI, such as security risks and the need for comprehensive governance. This ensures data integrity and compliance and paves the way for innovative and efficient use of data, thereby enhancing the overall decision-making process and strategic planning within organizations.
- What is the use of Generative AI in Banking Sector?
- Importance of Generative AI in Insurance Sector
- Role of Master Data Management in Manufacturing Industries