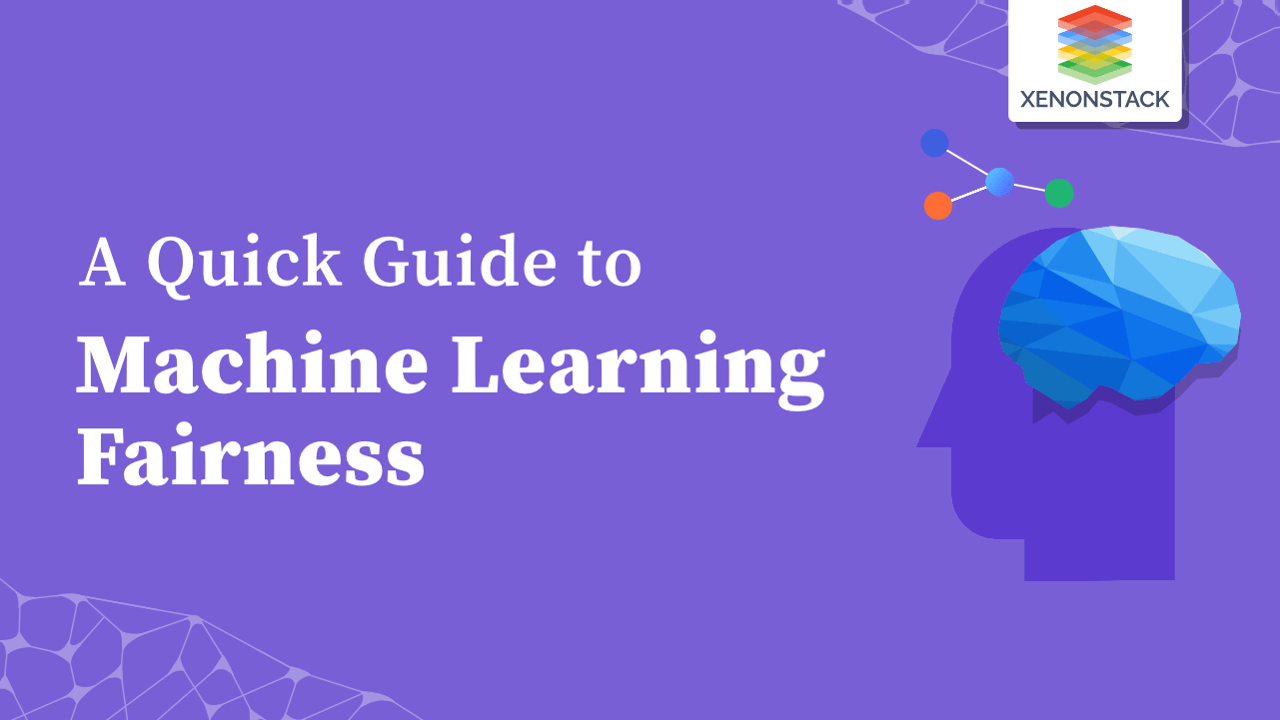
Introduction to Machine Learning (ML)
Machine Learning (ML) has proven to be one of the most transformative technological advances in the last decade. In today's highly competitive business landscape, ML is helping firms accelerate digital transformation and enter the age of automation. Some claim that AI/ML is essential to remain relevant in some industries, such as digital payments, fraud detection in banking, and product suggestions.
The ultimate acceptance and pervasiveness of machine learning algorithms in corporations are well documented, with several companies using machine learning at scale across sectors. Machine learning is now used in some way or another by every other tool and software on the Internet. Machine Learning has become so prevalent that it is now the go-to method for businesses to handle various issues.
Deep Learning algorithms mimic human brains using artificial neural networks and progressively learn to accurately solve a given problem. Click to explore our, Deep Learning: Guide with Challenges and Solutions
What does fairness mean for Machine Learning systems?
Fairness in Machine Learning refers to numerous initiatives to address algorithmic bias in machine learning-based automated decision systems. Definitions of fairness and prejudice, like many other ethical ideas, are constantly contentious. Fairness and prejudice are regarded as necessary anytime a choice affects people's lives, particularly when a collection of sensitive characteristics, such as gender, race, sexual orientation, handicap, and so on, are involved.
The algorithmic bias in machine learning is well-recognized and thoroughly investigated. Outcomes may be skewed by various circumstances and hence be regarded as unfair about specific groups or people. One example is how social media sites give customized news to users.
Why is fairness important in Machine Learning (ML)?
Fairness in data and machine learning algorithms is essential for designing safe and responsible AI systems from the start. Both technical and corporate AI players constantly strive for fairness to handle issues such as AI bias effectively. While accuracy is one metric for assessing a machine learning model's performance, fairness allows us to comprehend the practical consequences of deploying the model in a real-world context.
The act of recognizing bias provided by your data and ensuring your model produces equal predictions across all demographic groups is known as fairness. Rather than considering fairness as a distinct project, it is critical to use fairness analysis across the whole ML process, constantly assessing your models from the standpoint of fairness and inclusiveness. This is especially crucial when AI is used in critical business operations influencing various end-users, such as credit application evaluations and medical diagnoses.
ModelOps helps organizations to implement AI solutions and manage and monitor their performance at scale. Discover about ModelOps vs MLOps
Why do we care about fairness in Machine Learning?
Our world would not be better if we made judgments based on preconceptions and qualities unrelated to the decision-making process. If such a world existed, it would only be a matter of time before we, as people, were victims of prejudice.
Here are some recent instances of incidents in which ML systems were not intended to be biassed, but when put into practice, they proved to be discriminatory and damaging to the public:
-
COMPAS is a case management and decision support tool used by US courts to estimate the possibility of a defendant becoming a repeat offender. According to ProPublica, the COMPAS algorithm incorrectly projected that black offenders were more likely to recidivate than they were.
-
The Amazon Hiring Algorithm In 2014, Amazon worked on a project to automate the candidate resume evaluation process. However, Amazon discontinued its experimental ML recruiting tool after it was discovered to be discriminatory toward women.
-
The Apple Card is a credit card provided by Goldman Sachs that Apple Inc designed. It was released in August of 2019. After a few months of protest, customers began to protest that the card's algorithms were biased against women.
What causes bias in ML?
Because decision-making solely depends on facts, machine learning algorithms appear objective. In a normal workflow, an algorithm is provided with a significant quantity of representative data to learn from, and what it sees defines its decision-making process. However, whatever data we provide, the algorithm describes, directly or indirectly, societal decisions that have already been made. If black defendants are already mistakenly found to be more dangerous than white defendants, an algorithm will learn this from the data as if it were true. This biased, inaccessible training data creates a feedback loop in which the algorithm makes unjust conclusions based on what it has learned, perpetuating more social inequality and tainting future data.
Too much uniformity in the data can also lead to unfairness. A typical example is Nikon's facial recognition technology, which automatically recognises blinking in photographs. However, this system incorrectly identified Asians as blinking at a far greater rate than other groups. Although Nikon did not specify the specific explanation, this circumstance illustrates what might happen when an algorithm only provides data from a subset of the population. The algorithm would not have been able to accurately understand what an Asian person's blinking looks like without seeing numerous samples of Asian individuals.
Human-centered AI approach is to always think about the people behind the data, whose data is, how we are using it, that data identifies people, or if they consent to it. Click to explore our, What is Human-Centred AI Design Principles?
How to eliminate bias in Machine Learning?
Eliminating data bias in machine learning is a never-ending task. Near-constant data cleansing and machine learning bias are required to construct reliable and meticulous data-gathering systems. Machine learning bias may be avoided with education and proper governance. Eliminating data bias necessitates first determining where the prejudice exists. Once found, the bias in the system may be eliminated. (See Automation: What Does the Future Hold for Data Science and Machine Learning?)
However, it is frequently challenging to determine whether the data or model is skewed.
Nonetheless, specific procedures may be performed to handle this type of circumstance. These are some examples:
-
Testing and verifying machine learning system findings guarantees that algorithms or data sets do not cause bias.
-
Ensure a varied mix of data scientists and data labellers.
-
Setting specific standards for data labelling expectations so that data labellers know what procedures to take when annotating.
-
Bringing several source inputs together to provide data diversity.
-
Analyzing data regularly and keeping track of faults so they may be resolved as soon as feasible.
-
A domain expert will be used to examine the gathered and annotated data. Someone outside the team may see unchecked prejudices.
-
Examining and inspecting ML models using external resources such as Google's What-if Tool or IBM's AI Fairness 360 Open Source Toolkit.
-
Implementing multi-pass annotation for any project where data perfection is likely skewed.
Fairness in machine learning is about more than simply preventing a model from injuring a protected group; it may also assist in focusing attention where it is most needed. Models might be used to provide translation services in regions where in-person translators are uncommon, medical knowledge in areas where experts are scarce, and even enhance diagnostic accuracy for rare disorders frequently misdiagnosed. We can ensure that all patients benefit from this technology by prioritizing fairness in building, deploying, and assessing the models.
- Discover here about MLOps Roadmap for Interpretability.
- Explore the ML Platform for Training and Serving
- Read More Deep Learning vs Machine Learning vs Neural Network
Next Steps in Machine Learning Systems
Talk to our experts about implementing advanced machine learning systems and how industries utilize Agentic workflows and Decision Intelligence to drive decision-centric operations. Machine learning helps automate and optimize IT support, improving efficiency and responsiveness across departments.