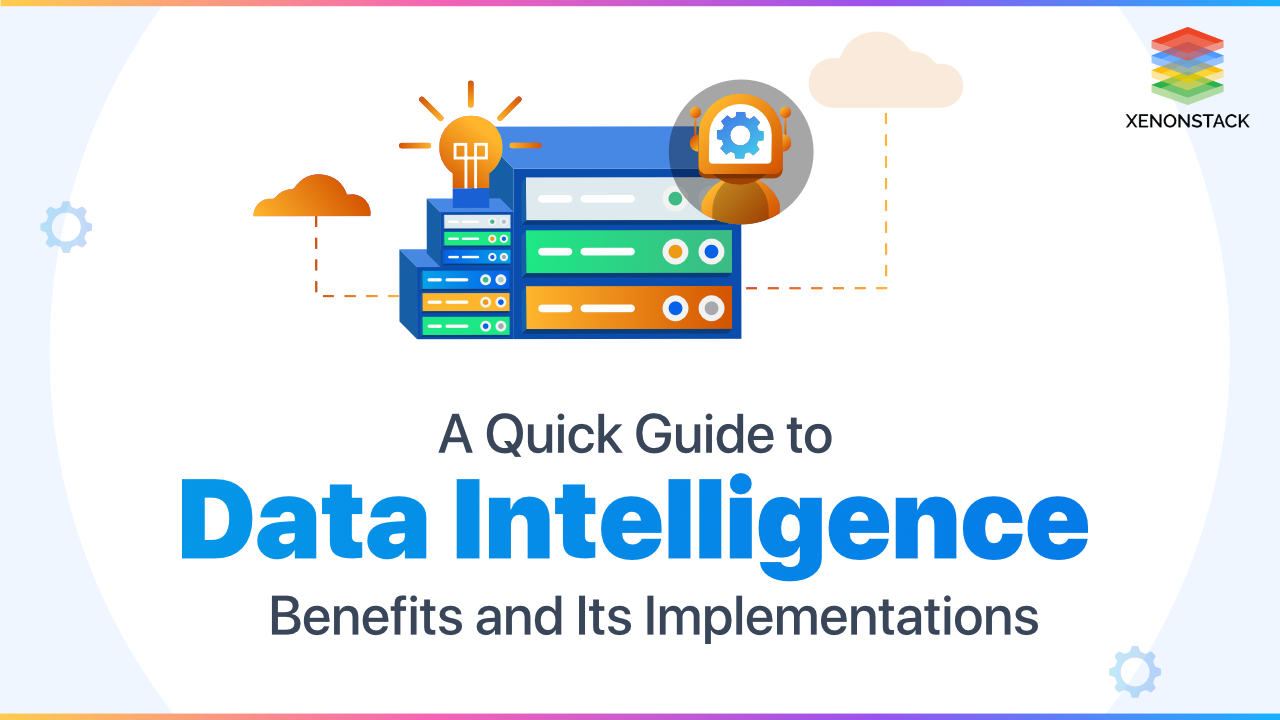
Introduction to Data Intelligence
We generate massive amounts of data every minute in today's digitalised world. The volume of data generated by large corporations grows at a pace of 40 to 60 per cent per year. It's difficult to keep, organize, use, and evaluate all data. As a result, organizations are scrambling to find methods to make this immense amount of data meaningful because we know that the value of information lies in the information itself and the actions that emerge from the knowledge. Simply storing this massive amount of data would be ineffective. Thus, businesses rely on data intelligence, analytics, and data intelligence technologies.
Continually analyze data and automatically improve development and operations techniques. Taken From Article, DevOps with Continuous Intelligence
What is Data Intelligence?
Data intelligence is a method of delivering trustworthy and reliable information. Insights about how a resource has been used in the past help educate how it might be used effectively. It includes intelligence about data or metadata. The concept was coined by IDC (International Data Corporation), which stated that "data intelligence helps enterprises answer six essential questions about data." These are the questions:
It also poses much wider issues that help to flesh out an organization's relationship with data:
- Why do we have data?
- Why should data be stored at all?
Answering these questions can increase operational efficiencies and support a variety of data intelligence use cases, such as data governance and self-service analytics.
Data intelligence first emerged to enhance search and data discovery, mostly to increase analyst productivity. For years, enterprise analysts had struggled to find the data they required to create reports. This issue was only exacerbated by the rapid increase in data collection and volume. The first Data intelligence use cases relied on metadata — popularity rankings indicating the most commonly used data — to uncover assets that would be most beneficial to others.
Finding data, however, is only the beginning. Analysts have questions before they have queries. They need to know:
- Who has previously used this data?
- How did they bring it to use?
- What should I do with it... if I'm permitted to use it at all?
As a result, data intelligence has grown to answer these challenges, and it now serves a wide range of use cases. The following are some examples of Data Intelligence use cases:
Furthermore, Data intelligence has evolved as a solution to the dilemma of garbage-in, garbage-out that has hampered AI and BI efforts for years. Data intelligence is a collection of categories that include:
Metadata Management
Metadata management is managing metadata about other data, which is sometimes referred to as content data. The term is most commonly associated with digital media, but previous forms of metadata include catalogues, dictionaries, and taxonomies.
Data Quality
The status of qualitative or quantitative information is referred to as data quality. There are numerous definitions of data quality, but typically, data is considered high quality if it is "suited for intended purposes in operations, decision making, and planning."
Data Governance
Data governance is a set of processes, responsibilities, policies, standards, and measurements that assure an organization's effective and efficient use of information to achieve its goals.
Master Data Management
Master data management is a technology-enabled discipline in which business and information technology work together to ensure the uniformity, accuracy, stewardship, semantic consistency, and accountability of the enterprise's official shared master data assets.
Data Profiling
Data profiling evaluates data from an existing information source (for example, a database or a file) and compiles statistics or instructive summaries about that data.
Data Curation
The organizing and integration of data acquired from multiple sources is referred to as data curation. It entails annotating, publishing, and presenting data so that its value is maintained throughout time and the data is available for reuse and preservation.
Data Privacy
Data privacy refers to protecting personal data from those who should not have access to it and individuals' power to control who has access to their personal information.
However, where does data analytics fit when data intelligence has been used to make raw data more useful?
What are the benefits of Data Intelligence?
Data Intelligence (DI) assists data experts in making better decisions. Its purpose as a system is straightforward: to make people in a company smarter about the organization and better suited to lead its growth.
The following are some of the essential high-level benefits:
Adaptive Decision Making
Leaders can stay ahead of the competition by making faster decisions at the moment when their BI dashboards include correct, up-to-date information. Businesses may adjust their strategies in real-time to better predict client wants and serve them.
Stronger Data Foundations
DI monitors processes regularly to streamline operations and better support key stakeholders. Furthermore, DI provides AI and BI use cases with trustworthy and reliable data by ensuring quality.
Operational Efficiencies
Data search and discovery connect people to the information they require. Previously, an analyst could spend up to six weeks merely looking for reliable data collection. DI separates the wheat from the chaff, highlighting the most trustworthy assets for wider use while increasing operational efficiency.
Augmented Analytics
DI enables analysts to apply augmented analytics to applications, allowing them to support predictive and prescriptive analytics use cases.
Transparency Supports Teamwork and Trust
DI unifies the minds behind an organization's guiding ideas and the process for crystallizing them by developing a framework for proving new truths.
What is Data Intelligence and Data Analytics?
Data intelligence presents data so that decision-makers and stakeholders can understand and analyze it. This procedure can be carried out either manually or automatically, utilizing AI, machine learning, or a mix of the two. On the other hand, data analytics refers to studying data to make predictions and take action based on what it tells you.
Use Cases of Data Intelligence
The Use Cases of Data Intelligence and Data Analytics are listed below:
Renewable Energy
A certain large organization is attempting to increase transparency and reduce the risk for investors in renewable energy, a critical task with worldwide implications. They employ data intelligence to build machine learning models that constantly compare the previous value of power companies with their current performance to provide up-to-date risk assessments for investors to use in their decision-making.
Deep learning is a critical component of data intelligence, and this use case also addresses a crucial part of data management security. Data access management is critical for businesses that handle a big volume of potentially sensitive data, such as investment data, and must keep access to it secure and closely controlled.
Manufacturing Corporation
Consider a manufacturing corporation that wants to reduce downtime caused by equipment breakage, repairs, faults, and part delivery wait times. Machine learning algorithms can use data like equipment make, model, and year, log entries, sensor data, error messages, and usage rates to create a system that can forecast when particular machines will need maintenance — before they fail.
This use of data analysis aids in optimizing maintenance schedules, reducing machinery downtime, and reducing lost profits due to production delays.
How to implement Data Intelligence?
Data leaders may integrate Data intelligence into their regular business activities by working with a data intelligence platform best suited to their goals.
Consider the following three basic steps for the best strategy:
Know Your Data
Find sensitive and critical data everywhere with transformational data discovery, which combines next-generation ML cataloguing, classification, cluster analysis, and correlation across all forms of data.
Action Your Data
Take action to safeguard data privacy, protection, and perspective with modular apps built on the first purpose-built app framework and for today's data-driven world.
Unleash the Value of Your Data
Automate data-driven projects to extract more value from your data, no matter where it resides. Accelerate data solutions by enabling the data fabric with an active metadata hub. With an extensible data intelligence platform, you may get flexible data solutions for regulatory and industry concerns.
An important part of Data Science, includes two concepts such as Data Cleaning and Feature Engineering. Click to explore about, Data Preprocessing and Data Wrangling in ML
What are the Data Intelligence Technologies?
Data scientists are continually looking for newer technology and techniques that can unlock the value of big data and distill it further to uncover additional insights that can alter productivity and give business distinction. The following technologies should be a part of every company’s data intelligence strategy.
Cloud data protection (CDP)
Cloud data protection (CDP) encrypts sensitive data before it is sent to the cloud, with the company (rather than the cloud provider) keeping the keys. Protects against unwanted government surveillance and aids in the removal of some of the most significant barriers to cloud adoption—security, compliance, and privacy concerns. Bitglass, CipherCloud, Cisco, Netskope, Skyhigh Networks, Symantec, and Vaultive are some examples of vendors.
Data Pipeline Management
Data pipeline management begins with data ingestion. Data flow mapping, change management, data lineage tracking, data availability, and data accuracy are supported by pipeline management solutions from ingestion to the point of application. The most significant aspect of pipeline management is that it allows operational data flow. Pipeline technologies assist BI and analytics designers, developers, and operators in creating, executing, monitoring, and managing data transit within the ecosystem.
Data Cataloging
Data cataloging keeps track of the inventory of data sets. Catalog tools help collect and maintain metadata, which is the key underpinning of the analytics ecosystem. A data catalog connects individuals to data by allowing them to search, evaluate, comprehend, and acquire the information they require. The fundamental goal of data cataloging is to assist data consumers – particularly self-service consumers – in finding and accessing the required datasets.
Tokenization
It uses a token to replace sensitive data such as credit card numbers, bank account numbers, and social security numbers. After tokenization, the token's mapping to its original data is saved in a secure database. Unlike encryption, there is no mathematical relationship between the token and its original contents; a hacker must have access to the mapping database to reverse the tokenization. CyberSource (Visa), Gemalto, Liaison, MasterCard, and MerchantLink are some examples of sample vendors.
Conclusion
The organization has a lot of data from various sources and procedures that everyone wants to turn into information like KPIs, product values, or customer insights. Every piece of information has the potential to be a critical success factor for an organization. While Business Intelligence mainly focuses on control, data intelligence uses data to identify valuable possibilities through appropriate analytic procedures.
We can combine data intelligence with enterprise architecture, business process, and data modelling capabilities to assist organizations in mitigating risks and achieving desired outcomes, such as regulatory compliance, agile innovation, and business transformation. It allows businesses to examine how data flows through and affects all of their business, technology, and data architectures.
- Explore about Augmented Intelligence Tools
- Read here about Data Intelligence vs Data Analytics