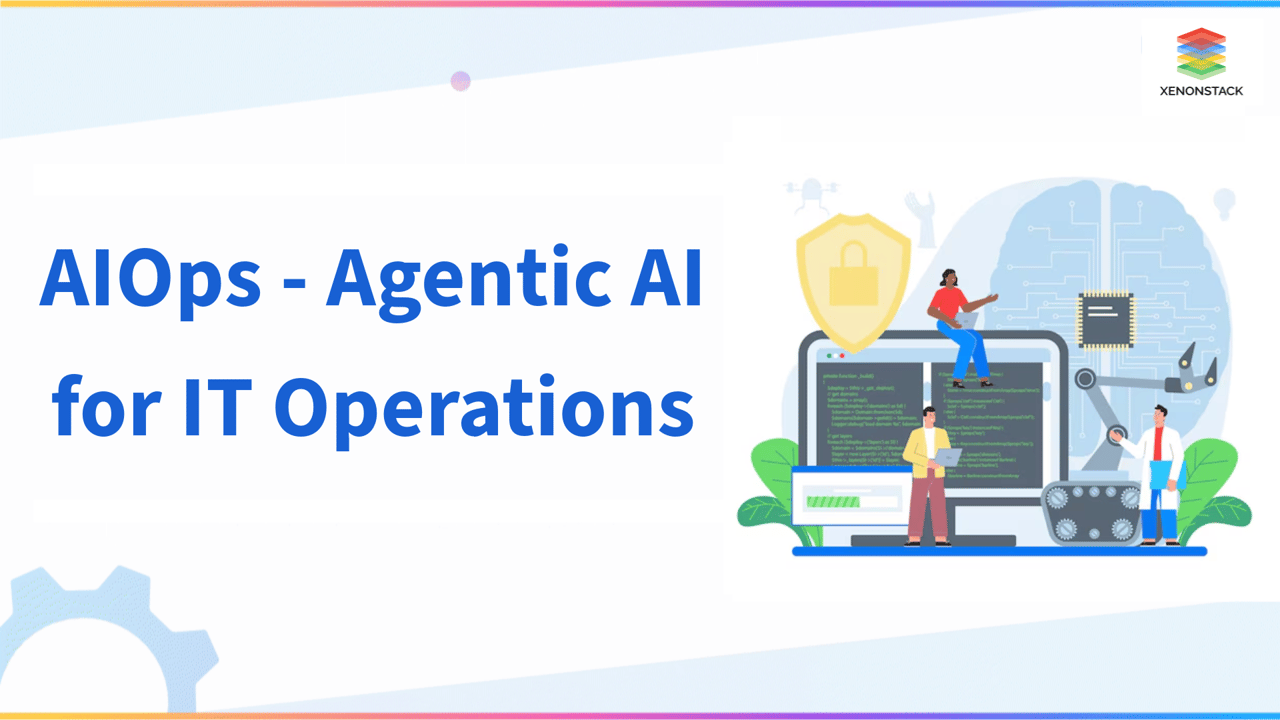
In an age of rapid digital transformation, businesses face unprecedented technological complexity. The need for efficient, intelligent, and responsive IT operations has never been greater. Enter AIOps (Artificial Intelligence for IT Operations). This innovative solution leverages the power of AI, machine learning (ML), big data analytics, and automation to optimize IT operations and enhance the efficiency of IT teams. AIOps is not just a tool or a trend; it represents a profound shift in how organizations manage and interact with their IT infrastructure.
Initially coined by Gartner, AIOps is becoming a cornerstone of modern IT operations, helping organizations manage increasingly complex IT systems. This blog delves deep into AIOps's transformative capabilities, historical evolution, integration of generative AI, real-world applications, and future trajectory.
The Evolution of AIOps
AIOps is an evolution of traditional IT operations that emerged from the growing complexity of IT systems and the overwhelming amount of data generated by digital technologies. Initially, the term "AIOps" was used to refer to "Algorithmic IT Operations," but it has since evolved into a more sophisticated system. The core idea behind AIOps is simple: harness the power of AI and ML to automate and optimize operations, mitigate risks, and drive efficiencies.
Early IT operations relied heavily on manual intervention, monitoring, and troubleshooting. As businesses expanded their digital footprints, these processes became too complex and resource-intensive. The rise of cloud computing, microservices, hybrid environments, and IoT (Internet of Things) technologies resulted in exponentially more data, making manual monitoring and incident response difficult to manage effectively.
AIOps was born out of the necessity to address these challenges. AIOps platforms can identify patterns, predict issues, and even automate resolutions by applying machine learning and AI to vast quantities of operational data. This shift enables organizations to move from reactive troubleshooting to proactive, predictive management.
What is AIOps?
At its core, AIOps is a comprehensive strategy that blends several cutting-edge technologies:
Fig 1: AIOps
-
Artificial Intelligence (AI): To make intelligent, real-time decisions.
-
Machine Learning (ML): For automated analysis and continuous learning.
-
Big Data Analytics: To process vast amounts of IT data and generate insights.
-
Automation Technologies: To ensure swift action and problem resolution.
Key Components of AIOps
-
Data Selection: Filters out irrelevant data, highlighting the critical pieces that can indicate potential problems.
-
Pattern Discovery: Identifies patterns in data relationships and provides deeper insights into system behaviours.
-
Inference: Pinpoints the root causes of issues, helping detect and resolve recurring incidents.
-
Collaboration: Improves communication across teams, even those spread out globally.
-
Automation: Automates responses to incidents, ensuring rapid and accurate resolutions.
Who Uses AIOps?
Companies with extensive IT environments and working on multiple technologies are having difficulty expanding and issues while scaling. So, for them, AIOps can prove to be a life saviour. It can play a massive role in bringing success to the company. All organizations now want to scale rapidly and increase their growth, so they, in turn, create more demand for agility in IT.
-
DevOps Teams: AIOps helps align Dev and Ops teams by ensuring Devs have clear insights into the environment. At the same time, Ops can track and control changes and deployments, boosting agility and project success.
-
Cloud Computing: AIOps simplifies managing hybrid cloud platforms, reducing risks and streamlining the complexities of scaling IT infrastructure.
-
Digital Transformation: AIOps supports faster and more efficient delivery of digital transformation projects by eliminating IT bottlenecks, enabling businesses to meet agility and speed requirements.
Agentic AI Integration: Elevating AIOps
Generative AI has introduced a new level of sophistication to AIOps. While traditional AIOps focused on monitoring, identifying patterns, and automating responses, the integration of generative AI takes this a step further by enabling the system to anticipate problems and suggest proactive actions. This predictive capability is a game-changer, allowing organizations to prevent issues before they occur and optimize their IT operations in real time.
Fig 2: Benfits of Agentic AI Integration
-
Automated Incident Response: Agentic AI models can analyze historical data and recommend context-aware actions for incident management. Instead of simply alerting IT teams about issues, AIOps systems can now automatically generate action plans based on past incidents. These suggestions can include specific remedial actions, configuration changes, or system optimizations.
-
Predictive Maintenance: AIOps platforms can forecast potential system failures or performance issues before they occur. This allows organizations to schedule preventive maintenance or take preemptive actions, avoiding downtime and ensuring continuous operations. Predictive maintenance also helps extend IT infrastructure's lifespan, improving cost efficiency.
-
Intelligent Log Analysis: Logs are a critical data source for IT teams to diagnose issues and understand system behaviour. With generative AI, AIOps systems can create synthetic logs and use advanced algorithms to detect anomalies more accurately. This can significantly reduce the time it takes to detect issues and improve the quality of insights gathered from log data.
Practical Applications of AIOps
AIOps is not a one-size-fits-all solution. Its applications span various industries and use cases, offering tailored benefits depending on the specific needs of each organization. Below are some key applications of AIOps across different sectors:
1. DevOps Integration
Challenges in DevOps
-
Complex Workflow Coordination: DevOps requires close collaboration between development and operations teams. Misalignment or communication gaps can lead to inefficiencies, errors, and delays in software deployment.
-
Manual Monitoring and Incident Response: DevOps teams often rely on manual intervention to monitor systems and resolve issues, which can be time-consuming and prone to human error.
-
Scalability Issues: As DevOps environments scale, managing many services, containers, and deployments becomes increasingly challenging.
How Agentic AI Enhances DevOps
Fig 3: AIOps in DevOps Integration
-
Automated Incident Management: Agentic AI in AIOps platforms automates incident detection and resolution by analyzing historical data and identifying recurring issues. AI-driven systems can provide actionable insights in real-time, reducing the need for manual intervention.
-
Continuous Feedback Loops: With AI-powered analytics, Agentic AI enables continuous monitoring, offering predictive insights and automated responses that help streamline deployment processes and avoid errors.
-
Collaboration Enhancement: AI can improve communication between development and operations teams by providing a unified view of system performance, application health, and incident trends, promoting faster collaboration.
Benefits of AIOps in DevOps
-
Increased Efficiency: By automating monitoring, incident response, and workflow optimization, DevOps teams can reduce time-to-resolution and improve productivity.
-
Faster Time-to-Market: Automation and AI-driven insights lead to quicker deployments and fewer errors, reducing the cycle time for releasing new features and updates.
-
Improved Collaboration: With AI facilitating communication and offering a holistic view of the deployment process, teams can collaborate more effectively, minimizing the risk of operational silos.
2. Cloud Computing
Challenges in Cloud Computing
-
The complexity of Hybrid Cloud Environments: Many businesses operate hybrid cloud environments, which combine on-premise data centres with public and private cloud services. Managing resources across these different platforms can be complex and difficult to optimize.
-
Dynamic Resource Allocation: Cloud resources are often underutilized or overburdened, leading to inefficiencies. Manual resource scaling based on demand is inefficient and prone to human error.
-
Performance and Cost Optimization: It can be challenging to optimize both performance and costs in cloud environments. Mismanagement of cloud resources can lead to increased expenses without improving performance.
How Agentic AI Enhances Cloud Computing
-
Automated Resource Scaling: Agentic AI uses predictive analytics and machine learning to monitor cloud resource usage patterns. It can automatically scale resources up or down depending on demand, ensuring optimal resource allocation and preventing over-provisioning.
-
Cost Optimization: By analyzing usage trends, Agentic AI can suggest cost-saving measures, such as reducing idle resources or recommending better instance types, improving performance and cost efficiency.
-
Predictive Performance Monitoring: AI continuously analyzes data from cloud environments, predicting potential performance bottlenecks or failures before they occur. It can trigger automated remediation processes or alert teams, allowing proactive management.
Benefits of AIOps in Cloud Computing
-
Optimized Resource Utilization: Dynamic resource scaling helps ensure that cloud infrastructure is continually optimized for cost and performance, eliminating wastage and enhancing overall efficiency.
-
Reduced Operational Costs: By providing cost-saving insights and automating resource allocation, businesses can lower their cloud expenses significantly.
-
Proactive Problem Solving: Predictive monitoring reduces downtime by catching issues before they impact services, ensuring consistent performance and a smooth user experience.
3. Kubernetes and Serverless Environments
Challenges in Kubernetes and Serverless Environments
-
Dynamic Resource Management: Kubernetes and serverless environments involve complex, dynamic resource allocation. Ensuring that resources are efficiently allocated and scaled to meet changing demands can be tricky.
-
Scaling Complexity: Kubernetes clusters and serverless systems require automatic scaling of resources, which can sometimes lead to inefficiencies, such as over-provisioning or underutilization.
-
Visibility Issues: Gaining visibility into the health and performance of applications in containerized or serverless environments can be difficult due to the ephemeral nature of these environments.
How Agentic AI Enhances Kubernetes and Serverless Environments
Fig 4: AIOps in Kubernetes and Serverless Environments
-
Intelligent Load Balancing: Agentic AI leverages machine learning to optimize load balancing across Kubernetes clusters and serverless functions. By predicting the right amount of resources needed for each service or function, AI ensures that resources are allocated in real-time based on traffic and performance needs.
-
Automated Resource Scaling: AI can monitor the health and usage patterns of containers and serverless functions and automatically adjust the resources (CPU, memory, etc.) allocated to each, ensuring optimal performance without manual intervention.
-
Advanced Monitoring and Observability: AI-driven platforms provide advanced observability into Kubernetes and serverless environments, offering insights into microservices and application performance at a granular level, even across distributed systems.
Benefits of AIOps in Kubernetes and Serverless Environments
-
Improved Resource Efficiency: Automated resource allocation and load balancing lead to better resource utilization, preventing overuse or waste of computing power.
-
Enhanced Performance: Proactive scaling and real-time monitoring ensure optimal performance in highly dynamic environments.
-
Reduced Operational Overhead: By automating many resource management tasks, AIOps reduces the operational burden on IT teams, allowing them to focus on more strategic initiatives.
4. Incident Management
Challenges in Incident Management
-
Manual Incident Handling: Traditional incident management processes involve manual logging, triaging, and ticketing of issues, which can be slow and error-prone.
-
Lack of Proactive Monitoring: Many organizations rely on reactive incident management, addressing issues only after they have caused significant disruption. This leads to increased downtime and service interruptions.
-
Coordination Issues: In large organizations with geographically dispersed teams, coordinating incident response efforts can be slow, leading to delays in resolution.
How Agentic AI Enhances Incident Management
-
Automated Ticket Generation and Resolution: Agentic AI can automatically detect incidents, categorize them based on severity, and create tickets for the appropriate team. In some cases, it can even provide initial diagnostic information and suggest possible solutions, speeding up incident resolution.
-
Predictive Incident Detection: AI-driven predictive models can identify patterns in historical data to forecast potential issues before they occur, allowing teams to address them proactively. This reduces the number of incidents and minimizes their impact on service availability.
-
Collaborative Incident Management: By centralizing incident data and automating communication, Agentic AI improves collaboration among support teams. It provides real-time updates on incident status and integrates with communication tools to ensure that everyone involved is on the same page.
Benefits of AIOps in Incident Management
-
Faster Incident Resolution: Automation and predictive analytics streamline the incident management process, reducing time-to-resolution and minimizing downtime.
-
Proactive Problem Prevention: Predictive insights allow IT teams to address issues before they become incidents, significantly reducing the number of incidents and their severity.
- Improved Collaboration and Efficiency: Automated communication and ticketing improve team coordination, ensuring incidents are handled promptly and accurately.
Technological Foundation of AIOps
AIOps is underpinned by a robust set of technologies that work together to manage large volumes of data and generate actionable insights. These technologies include:
-
Data Sources: AIOps platforms rely on various data sources, including IT logs, metrics, events, and monitoring data, to analyse and make decisions. These data sources provide the raw material for the AI and ML models to process and analyze.
-
Big Data Processing: AIOps platforms are designed to handle both historical and real-time data. Big data processing allows organizations to analyze massive datasets, identify trends, and generate predictive insights. Real-time analysis is critical for incident detection, while historical analysis helps identify recurring patterns and trends.
-
Machine Learning Algorithms: AIOps utilizes a variety of machine learning algorithms, including unsupervised learning, anomaly detection, and predictive analytics, to identify trends, detect anomalies, and predict future events. These algorithms are key to making AIOps systems more intelligent, as they enable the platform to learn from past data and continuously improve its predictions and decisions.
How are Observability and AIOps transforming the world?
Observability and AIOps are revolutionizing organizations' management of complex IT environments by providing real-time insights and intelligent automation. Their combined power drives greater efficiency, reliability, and responsiveness across industries.
-
Enhanced Incident Response: Real-time monitoring and AI-powered automation help detect and resolve issues faster, minimizing downtime.
-
Full-Stack Visibility: MELT (Metrics, Events, Logs, Traces) provides deep insights into system behaviour for better root cause analysis and performance tracking.
-
Proactive Monitoring and Predictive Analytics: Organizations can anticipate capacity needs and prevent issues before they occur using predictive insights from AIOps.
-
Automation of Routine Tasks: AIOps automate repetitive IT operations, improving efficiency and allowing teams to focus on strategic work.
-
Improved Resource Optimization: AI-driven analysis helps in allocating resources more effectively, reducing waste and improving performance.
-
Cross-Industry Impact: From finance and healthcare to retail, these technologies enhance reliability, customer experience, and operational resilience.
Together, Observability and AIOps are setting a new standard for intelligent, data-driven IT operations. As systems grow more complex, their importance will only continue to rise.
Challenges in AIOps Adoption
While AIOps offers numerous benefits, its adoption can be challenging. Here are some of the key obstacles organizations face when implementing AIOps:
-
Integration Complexity: Integrating AIOps tools with existing IT systems and workflows can be complex. Many organizations struggle with inconsistent or poor-quality data, which can hinder the effectiveness of AIOps platforms. Furthermore, integrating AIOps with legacy systems may require significant effort and resources.
-
Organizational Resistance: The introduction of AI into IT operations often leads to resistance from employees who fear job displacement or feel uncomfortable with the cultural shift required to adopt AI-driven systems. Additionally, organizations may face challenges in upskilling their workforce to work effectively with new technologies.
-
Technical Limitations: AIOps platforms are powerful, but they are not foolproof. False positives, AI model biases, and difficulties in interpreting automated decisions can limit the effectiveness of AIOps. In complex environments, human expertise is still necessary to manage the nuances of certain IT systems and workflows.
Strategies for AIOps Implementation
For successful AIOps adoption, organizations should approach the implementation process strategically. Some key strategies include:
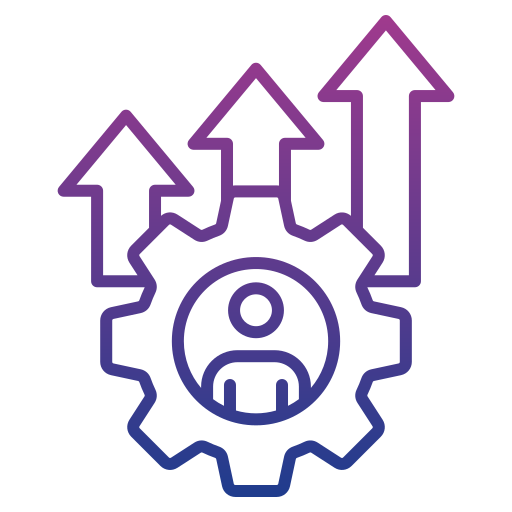
Gradual Adoption
Instead of implementing AIOps across the entire organization, start small with specific, well-defined use cases. This allows teams to learn and adapt to the new system while demonstrating the value of AIOps.
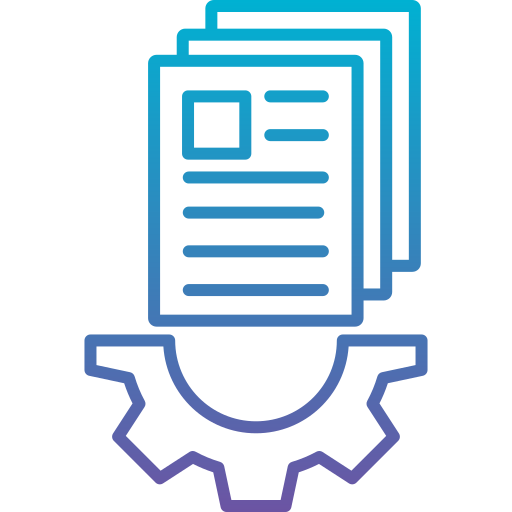
Data Preparation
For AIOps to be effective, data quality is paramount. Organizations should invest in cleaning and normalizing their operational data and creating robust data pipelines to ensure the success of AIOps initiatives.
Open-Source Tools for AIOps
Open-source tools play a significant role in AIOps and MLOps ecosystems, providing essential functionalities for monitoring, automation, visualization, machine learning workflows, and version control. Let’s explore some of the most popular open-source tools for AIOps and MLOps, breaking down their key features and uses.
Fig 5: Tools For AIOps
1. Prometheus
Prometheus is an open-source monitoring system designed for collecting and storing time-series data. It is widely used for its efficiency and flexibility in monitoring and alerting infrastructure and application performance.
Key Features:
-
Time-Series Data Monitoring: Prometheus excels in storing metrics data in a time-series format. It efficiently collects and stores data points associated with timestamps, such as CPU usage, memory utilization, or network throughput. This is especially valuable for monitoring dynamic systems that generate large amounts of time-series data.
-
Robust Querying Capabilities: Prometheus offers a powerful query language called PromQL (Prometheus Query Language), which allows users to extract detailed insights and perform complex queries on the collected data. It enables users to calculate averages, rate of change, and other metrics for deeper operational performance analysis.
2. Grafana
Grafana is an open-source data visualization platform that allows users to create rich, interactive dashboards to visualize time-series data and other metrics.
Key Features:
-
Advanced-Data Visualization: Grafana allows users to create highly customizable dashboards and charts. It supports various visualization options, including graphs, histograms, pie charts, heat maps, and more. This enables organizations to represent complex data in a user-friendly and actionable format.
-
Flexible Dashboarding: Grafana’s dashboard creation tools are extremely flexible. They enable users to combine data from multiple sources (e.g., Prometheus, InfluxDB, Elasticsearch) and display it in a unified view. Dashboards can be customized to focus on key metrics, trends, and operational insights.
Use Case:
-
Grafana is often paired with Prometheus to create visually intuitive dashboards that display time-series data. It's commonly used for monitoring cloud infrastructure, application performance, and network health. Combining data from different sources into a single view helps teams quickly identify performance bottlenecks and resolve issues.
3. OpenNMS
OpenNMS is an open-source network management platform designed to help organizations monitor and manage their IT networks, applications, and services.
Key Features:
-
Network Management: OpenNMS provides tools for managing network infrastructure, including device discovery, network monitoring, and performance tracking. It supports SNMP, JMX, and other protocols to ensure comprehensive visibility into network health.
-
Comprehensive Event Correlation: One of the key features of OpenNMS is its ability to correlate events from different network components, identifying patterns and root causes of problems. This event correlation is essential for reducing noise and focusing on high-priority issues.
Use Case:
-
Network operations teams typically use OpenNMS to monitor the health and performance of IT networks and services. It’s especially valuable in large, complex environments with multiple devices, offering centralized monitoring, alerts, and insights into network performance.
4. Loglizer
Loglizer is an open-source machine learning-based log analysis tool that is specifically designed to perform automated anomaly detection in log data.
Key Features:
-
Machine Learning-Based Log Analysis: Loglizer applies machine learning techniques to analyze logs, detect anomalies, and identify patterns that might indicate operational issues or security threats. It can classify logs, extract meaningful insights, and detect outliers or unusual behaviour.
-
Automated Anomaly Detection: By leveraging machine learning, Loglizer automates the detection of anomalies within logs. It uses algorithms like unsupervised learning to discover unexpected patterns without the need for predefined rules.
Use Case:
-
Loglizer is useful for IT operations teams who need to monitor logs for potential issues such as security breaches, performance bottlenecks, or configuration errors. It is particularly valuable in high-volume environments where manual log analysis is impractical. By automating anomaly detection, Loglizer can reduce the time spent on log analysis and quickly highlight critical issues.
Best Practices for AIOps Implementation
-
Start Small
Begin with explicit, manageable use cases to demonstrate value and ensure that AIOps tools align with business goals. Gradual expansion will allow for smoother integration and better adoption across teams. -
Invest in Training
Ensure that IT teams are well-equipped with the necessary skills to leverage AIOps effectively. This will foster better adoption and enable teams to maximize the value of AI-driven tools. -
Ensure Tool Compatibility
Choose AIOps tools that integrate easily with existing systems and workflows. Seamless compatibility will improve efficiency and enable better automation and collaboration between teams.
ALOps vs. MLOps vs. DevOps
Aspect |
AIOps |
MLOps |
DevOps |
Primary Focus |
Optimizing IT operations and infrastructure |
Managing the machine learning model lifecycle |
Automating and integrating software development and IT operations |
Key Objective |
Automate IT operations, incident management, and performance optimization |
Streamline model development, deployment, and monitoring |
Improve collaboration between development and operations teams |
Core Technologies |
AI, machine learning, automation, event correlation |
Machine learning, data versioning, model deployment |
Continuous integration, continuous deployment, automation |
Data Sources |
Logs, metrics, events, monitoring data |
Training data, model performance data |
Application code, configuration, infrastructure |
Main Users |
IT operations teams, site reliability engineers |
Data scientists, machine learning engineers |
Development and operations teams (Dev and Ops) |
Use Cases |
Predictive maintenance, anomaly detection, automated incident response |
Model versioning, model deployment, performance monitoring |
Automated testing, continuous deployment, infrastructure management |
Key Challenges |
Complex system integrations, data quality, false positives |
Model drift, reproducibility, scaling models |
Communication gaps, manual deployment processes, lack of automation |
Tool Examples |
Prometheus, Grafana, Splunk, OpenNMS |
Kubeflow, MLflow, DVC |
Jenkins, Docker, Kubernetes, GitLab |
Outcome |
Improved system performance, reduced downtime |
Faster model development, better model governance |
Faster software delivery, improved collaboration, operational efficiency |
Future Trends of Agentic AI in AIOps
As the field of AIOps continues to grow and mature, its capabilities will expand to meet the increasing complexity of IT operations and the need for faster, more efficient responses. Looking ahead, there are several key trends and advancements shaping the future of AIOps:
- More Sophisticated AI-Driven Automation: AIOps will increasingly use advanced AI to automate complex tasks like incident detection and remediation. This will improve efficiency, reduce manual workloads, and enable faster issue resolution.
- Enhanced Predictive Maintenance Capabilities: AI will improve the ability to predict system failures before they happen, allowing proactive maintenance. Addressing potential issues early will minimize downtime and optimize system reliability.
- Greater Cross-Platform Integration: AIOps will integrate more seamlessly across various platforms, providing a unified view of the entire IT ecosystem. This will enhance system-wide insights and improve coordination between different tools and platforms.
- Optimized Resource Management Across Complex Systems: AIOps will enhance the management of resources across dynamic environments like hybrid and multi-cloud systems. This will allow for more efficient allocation, scaling, and utilization of IT resources, reducing waste and improving performance.
Next Steps with AIOps
Talk to our experts about implementing AIOps with Agentic AI for IT Operations. Learn how industries and different departments can leverage Agentic Workflows and Decision Intelligence to become decision-centric. Utilize AI to automate and optimize IT support and operations, enhancing efficiency, predictive maintenance, and responsiveness.