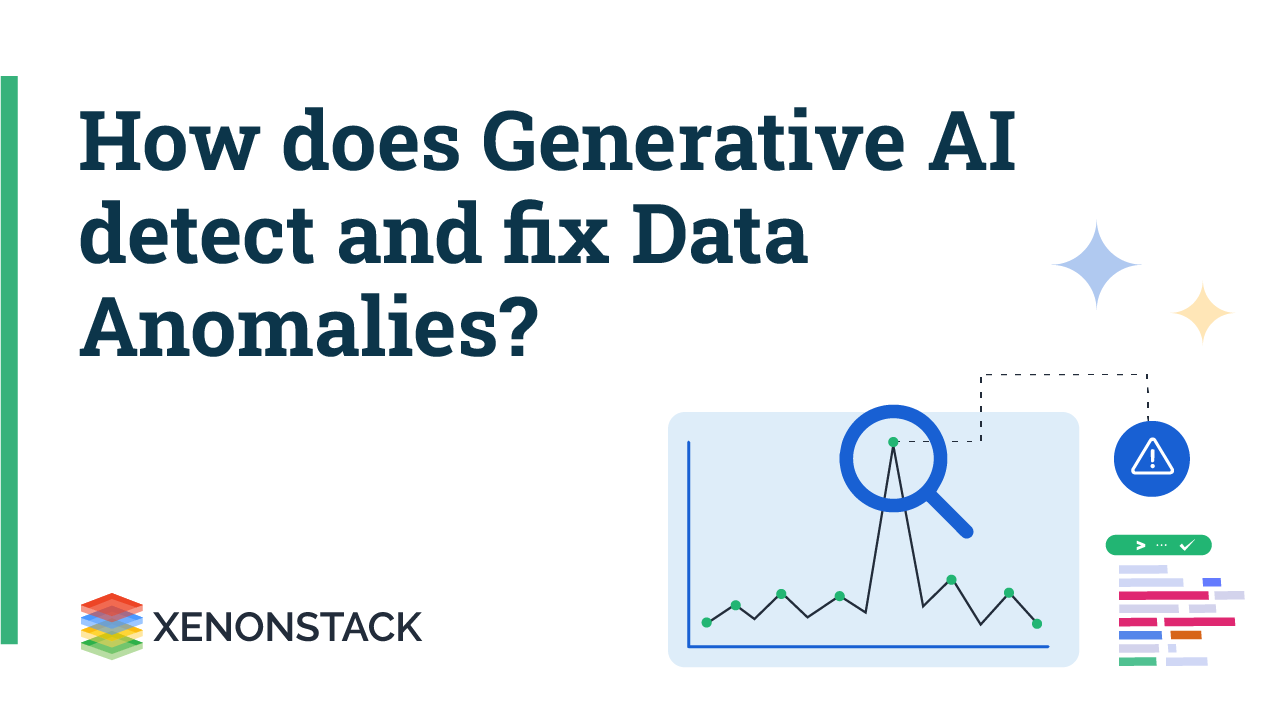
What is Generative AI?
Generative AI is a group of AI algorithms and models designed to create new content, like pictures, videos, text, or audio, based on the data they have been trained on. Generative algorithms can generate creative and original output by learning from large datasets of patterns and structures. Art, Entertainment, Design, and Technology are just a few industries that can benefit from the advances in AI.
Generative AI use cases
Banking & Financial Services: - Generative AI algorithms effectively detect and prevent fraudulent activities within the B&FS sector. By analyzing large volumes of data, Generative AI applications can identify anomalies indicating fraudulent behavior and help financial institutions detect fraudulent transactions, identity theft, and money laundering activities with greater accuracy and speed.
By analyzing customer data, including transaction history, browsing behavior, and demographic information, generative AI can recommend personalized offers, product recommendations, and tailored financial advice. This level of personalization helps banks and financial institutions build stronger customer relationships, improve customer satisfaction, and drive customer loyalty. Moreover, virtual financial advisors or chatbots driven by generative AI can augment the customer experience further by providing real-time assistance and personalized guidance.
Artificial intelligence (AI) technologies can analyze large amounts of financial information, market cycles, and historical data to predict risk and future market trends. By training the application on historical data, financial institutions can generate models to assess credit risk, predict market fluctuations, and optimize investment strategies. This can assist banks and financial institutions in making informed decisions, reduce losses, and enhance overall risk management processes.
Healthcare: - With the help of Generative AI models, researchers generate synthetic medical images that resemble actual patient scans to augment limited datasets, improve training models, and ultimately enhance the accuracy of diagnostic systems. Furthermore, Generative AI techniques enable high-resolution images from low-resolution inputs, improving the quality of medical imaging across different modalities and enhancing early detection of diseases, such as cancer, with more precise visualization and more accurate interpretation of medical images.
Manufacturing: - Generative AI models can analyze vast amounts of data and generate synthetic data representing different operating conditions to simulate and predict the performance of manufacturing processes, optimize production schedules, and identify potential bottlenecks or quality issues. It can enable rapid prototyping and design iteration. By generating realistic and diverse design alternatives, generative AI applications will assist engineers from early in the design phase.
What are Anomalies?
Anomalies, in data analysis and processing context, refer to peculiar data points that deviate significantly from a dataset’s expected or normal behavior. These deviations, whether large or small, can appear as a sudden spike or dip in activity, an error in the text, or an unusual change in temperature.
What is Anomaly Detection in AI?
Anomaly detection is a vital aspect of data science that centers on identifying unusual patterns that do not conform to expected behavior.
An anomaly detection system works by assessing and comparing data points within a dataset, singling out those that stand out from the regular pattern. The significance of detecting these anomalies isn’t merely about finding statistical quirks; it’s about uncovering valuable insights, underlying problems, or opportunities that might otherwise go unnoticed.
Why is Anomaly Detection essential?
1: - Responding to cybersecurity threats: - Modern firms often engage in complex, networked operations involving continuous data flow and exchange. In such a dynamic ecosystem, promptly identifying and reacting to anomalies becomes paramount, especially in the face of potential cybersecurity threats. Anomalies may signal intentional attacks, system flaws, or other vulnerabilities, and detecting them early can be the key to preventing or mitigating possible damage.
2: - Managing expanding datasets: - The sheer volume and complexity of data generated in contemporary business operations make manual management and evaluation practically infeasible. The task becomes even more challenging when the data constantly changes, and the definition of normal behavior continually evolves. Anomaly detection offers an automated and sophisticated solution to this challenge, enabling organizations to handle vast and intricate datasets effectively.
3: - Proactive approach to abnormal behavior: - Traditional methods that rely on human intervention are often reactive and may fail to catch issues in time. Anomaly detection provides a proactive approach, leveraging algorithms and technologies to monitor various components within dynamic systems. This continuous scrutiny ensures that deviations from the norm are promptly identified and addressed, even as the “normal” behavior baseline shifts over time.
How does Generative AI detect and fix Data Anomalies?
Data Anomaly Detection
- Autoencoders: Utilize autoencoder neural networks, an unsupervised learning algorithm, to learn the representation of routine data. Anomalies can then be detected by identifying data points that do not reconstruct well.
- GANs (Generative Adversarial Networks): Train GANs to generate synthetic data like the actual data. Any deviation between accurate data and generated data can indicate anomalies.
- Variational Autoencoders (VAEs): VAEs can learn the underlying distribution of regular data and identify data points that do not conform to this distribution as anomalies.
Data Anomaly Fixing
- Imputation Techniques: Use generative models to impute missing or anomalous data points. Generative models can generate plausible values for missing data points based on the learned patterns in the dataset.
- Data Synthesis: Generate synthetic data to replace anomalous data points. This is especially useful when dealing with sensitive data, as you can maintain privacy while ensuring the dataset's quality.
- Conditional GANs: Train conditional GANs where the condition is the surrounding data context. Generate data points conditioned on their neighboring points, ensuring coherence and realism.
Challenges and Considerations
- Quality of Generated Data: Ensure that the generated data is high quality and does not introduce new anomalies.
- Evaluation Metrics: Define appropriate evaluation metrics to measure the effectiveness of anomaly detection and data fixing. Common metrics include precision, recall, F1-score, and Mean Absolute Error (MAE).
- Training Data: A diverse and representative training dataset is crucial for the generative model to learn the underlying patterns effectively.
- Iterative Process: Anomaly detection and fixing might need an iterative approach. After fixing anomalies, re-check the data to ensure no new anomalies have been introduced.
Implementation Steps
- Data Preprocessing: Clean the data, handle missing values, and normalize the features before feeding them into the generative models.
- Model Training: Train the generative models (autoencoders, GANs, VAEs) on the preprocessed data.
- Anomaly Detection: Use the trained models to detect anomalies in the dataset.
- Data Fixing: Apply imputation techniques or generate synthetic data to replace or fix the anomalous data points.
- Validation: Validate the fixed data to ensure anomalies are resolved effectively without introducing new issues.
Continuous Monitoring
- Feedback Loop: Establish a feedback loop where the performance of the generative models is continuously monitored. Re-train the models as new data becomes available or as the data distribution changes over time.
- Human-in-the-Loop: Involve domain experts who can validate the results and provide insights, especially in complex scenarios where automated methods might fall short
Conclusion
By combining the power of Generative AI with careful preprocessing, validation, and domain expertise, you can effectively detect and fix data anomalies in various applications, ranging from finance and healthcare to manufacturing and beyond. As technology advances, AI’s role in anomaly detection will undoubtedly become even more integral, further solidifying its importance in shaping a smarter, more responsive future.
- Read about Generative AI for Energy Sector and its Benefits.
- Use of Generative AI Solutions for Cyber Security.
- Real-Time Anomaly Detection for Cognitive Intelligence.