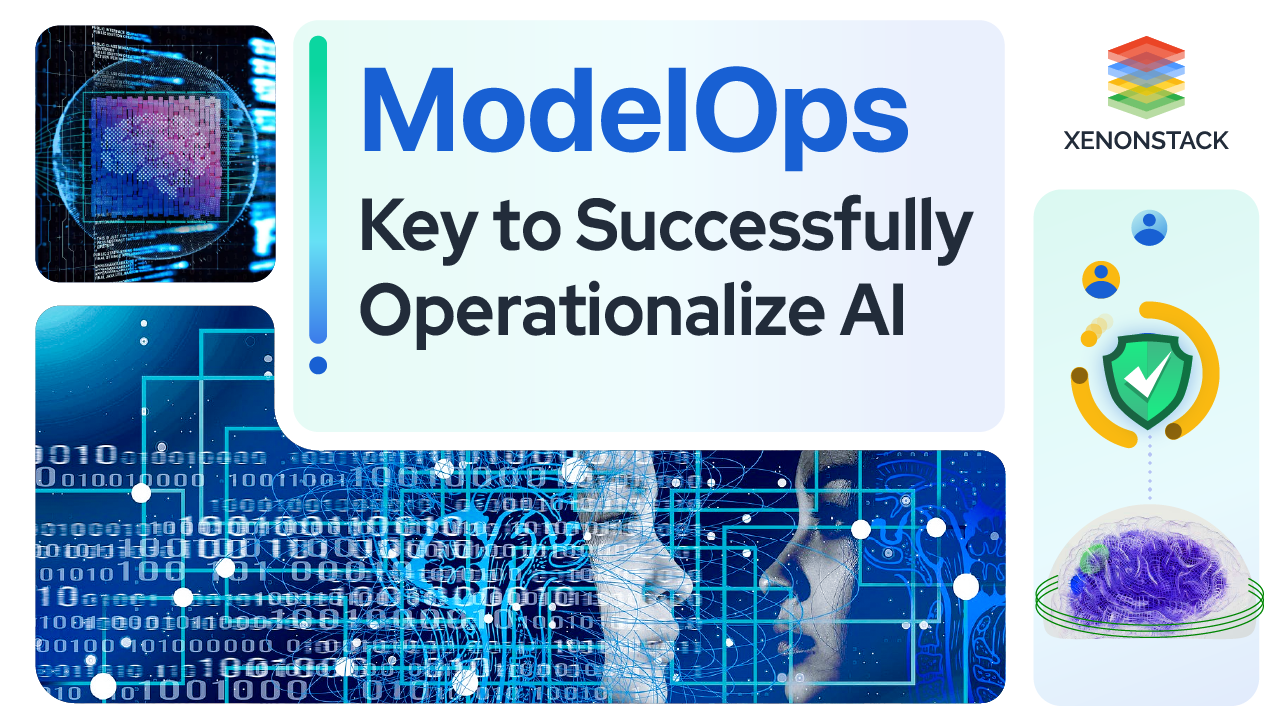
Introduction
Forrester's report on machine learning and predictive analytics vendors showed “Data scientists regularly complain that their models are only sometimes or never deployed. A big part of the problem is organizational chaos in understanding how to apply and design models into applications. But another big part of the problem is technology. Models aren’t like software code because they need a model management
What is ModelOps?
Gartner defines ModelOps as ModelOps (model operations), as defined by Gartner, "is focused primarily on the governance and life cycle management of a wide range of operationalized artificial intelligence (AI) and decision models, including machine learning, knowledge graphs, rules, optimization, linguistic and agent-based models" ModelOps is at the center of every organization's enterprise AI approach.
Three Core ModelOps capabilities that organizations must have if they’re going to succeed with AI at scale Identified by Forrester analysts Mike Gualtieri and Kjell Carlsson
-
For starters, Need to deploy and serve ML models
-
ModelOps provides monitoring capabilities to ensure ML models don’t go off the rails
-
ML lifecycle must be managed
Now you must be wondering how is ModelOps different from MLOps. Well, it's simple, MLOps focuses only on the Operationalization of ML models and ModelOps focuses on the operation of all AI and Decision Models. This we will cover in detail in the later half of the article.
Why ModelOps?
Organizations also struggle with analytics operationalization because they lack a formal system to organize resources through analytics, IT, and the organization. Since data alone doesn't drive the business, decisions do. They are making decisions that impact organizations each day. Since analytically driven decisions are smarter choices, it helps them make the right choices every time, even while making thousands or millions of them every day, by integrating analytics into the decision-making processes. This includes the operationalization of scale analytics.
-
The number of models - To account for business process variations, personalization, and specific customer groups, each organization will need to handle hundreds of models.
-
Technology Complexity - The fast and continuing innovation in data & analytics contributes to the unmanageable difficulty for even the most expert IT teams.
-
Regulatory Compliance - As the use of AI spreads across markets, adhering to strict and ever-increasing models, regulatory criteria becomes more challenging.
-
Organization Silos - Scaling AI in trends that are in businesses needs industry, technology, and data to come together. To ensure its free flow through the enterprise, the organizational data needs to be opened. This cannot occur in a siloed work culture, and organizations must develop an interdisciplinary team to push AI in the organization.
What are the Technologies Involved in ModelOps?
Multi-Cloud data and AI architecture: Invest in data-driven, cloud-based infrastructure and microservices will help the enterprises to enable the accelerated growth and rollout of software, as well as workload and model portability through several clouds. Think beyond machine learning models: To achieve the full benefits of a model-based approach, make use of AI models outside machine learning, such as organizational models, optimization models, and transformational models that will be helpful for the enterprises.
AI governance at scale: It will help to ensure that models are governed, trusted, and explainable and contribute to the creation of implementations that are consistent with market priorities, legislation relevant to compliance and are scalable to adapt to evolving business needs on a scale.
How to operationalize ModelOps?
Enterprises need to monitor the performance of the ModelOps software for the first phase of a ModelOps process. Since ModelOps is a growth, testing, rollout, and monitoring cycle, it will only be successful if it progresses against delivering the organization's size and accuracy. Then need to track each model's success at the organizational stage.
-
For models (or model classes), set accuracy goals and monitor them for dimensions such as drift and degradation through development, validation, and deployment.
-
Identify market indicators that are influenced in service by the model. Is a model designed to raise users, for instance, having a beneficial impact on subscription rates.
-
Track metrics such as the size of data and update frequency, positions, groups, and forms. Model performance concerns are often attributable to changes in the data and its sources, and these metrics can assist in your investigation.
-
Track the volume used by processing power or memory models.
Industry leaders use ModelOps to drive AI at scale
Enterprises drive AI into core processes at scale by focusing on three areas:
-
Model Centric Approach - Leaders manage models as first-class business properties, enabled by a model-centric infrastructure that facilitates long-term continuous implementation performance independent of data science workbench and execution platforms.
-
Operations First Mentality - Leaders recognize that deploying models to operation systems allows them to run 24×7 without delay, using the same operating controls, software, and automation that enable other technologies.
-
Automation - Leaders completely automate ModelOps processes, from implementation to monitoring and governance, to efficiently handle business model SLAs, eliminate manual execution, and mitigate risk, cost, and model time for business.
A Big Question? - ModelOps different from MlOps? How?
ModelOps performs model implementation and management during application creation and deployment lifecycle to operate models in production. In comparison to MLOps, which focuses exclusively on ML models' operationalization, and AIOps, which is AI for IT operations, ModelOps focuses on the operationalization of both AI and decision models.
In the past few years, we have seen how AI shifts from Data Science to build powerful AI models to figure out how to deploy models on production at scale. Many powerful tools can help train the models and use the right model for the specific use case. MLOps tools reduce the time to deploy models from months to a matter of hours. It provides powerful tools to monitor how the AI models perform in real-time, but there is still a missing link between the teams on building and deploying AI, where ModelOps come in. ModelOps provides transparency into how teams are deploying and using AI anywhere in the enterprise. This technology truly changes the current state of enterprises and provides explainability into AI-enabled outcomes.
ModelOps Solutions built to help large enterprises address these challenges
Major business companies have been speeding up their artificial intelligence and machine learning activities for the past few years with ModelOps. Organizations need to run their machine learning models around the enterprise to adapt models to hundreds of use-cases.
The companies were bringing an accumulating amount of "model debt" instead of real 24/7 production implementations of Enterprise AI adoptions. These are undeployed and unrefreshed models that leave businesses with millions on the table.
To compound the issue, as new capabilities such as AutoML arise, and industry demands further as market trends change, citizen data scientists have begun to implement bottom-line applications without the enterprise IT controls.
The lack of accountability in these systems will harm governance, not to mention costs. Large-scale models also need governance during implementation and close oversight to ensure that they comply with the regulations.
ModelOps aims to delineate these problems. The ModelOps Life Cycle can be a data science partner working with various technologies in specific business divisions to tap into specific data repositories to address business issues better. Models are mostly generated from historical knowledge and then deployed on production data to work. For the full data science project team, ModelOp aims to operationalize the analysis performed in all contexts through the ModelOps Lifecycle.
Conclusion
The ModelOps Life Cycle is relevant as more organizations are expanding their Enterprise AI initiatives. Probably soon, in the next few years, as more businesses use ModelOp to ramp up their Enterprise AI systems, companies will gradually see a major reduction in "model debt" across organizations. ModelOps - Your Key to Successfully Operationalize AI.
-
Explore more about Machine Learning Observability and Monitoring
-
Learn more about MLOps Roadmap for Model's Interpretability