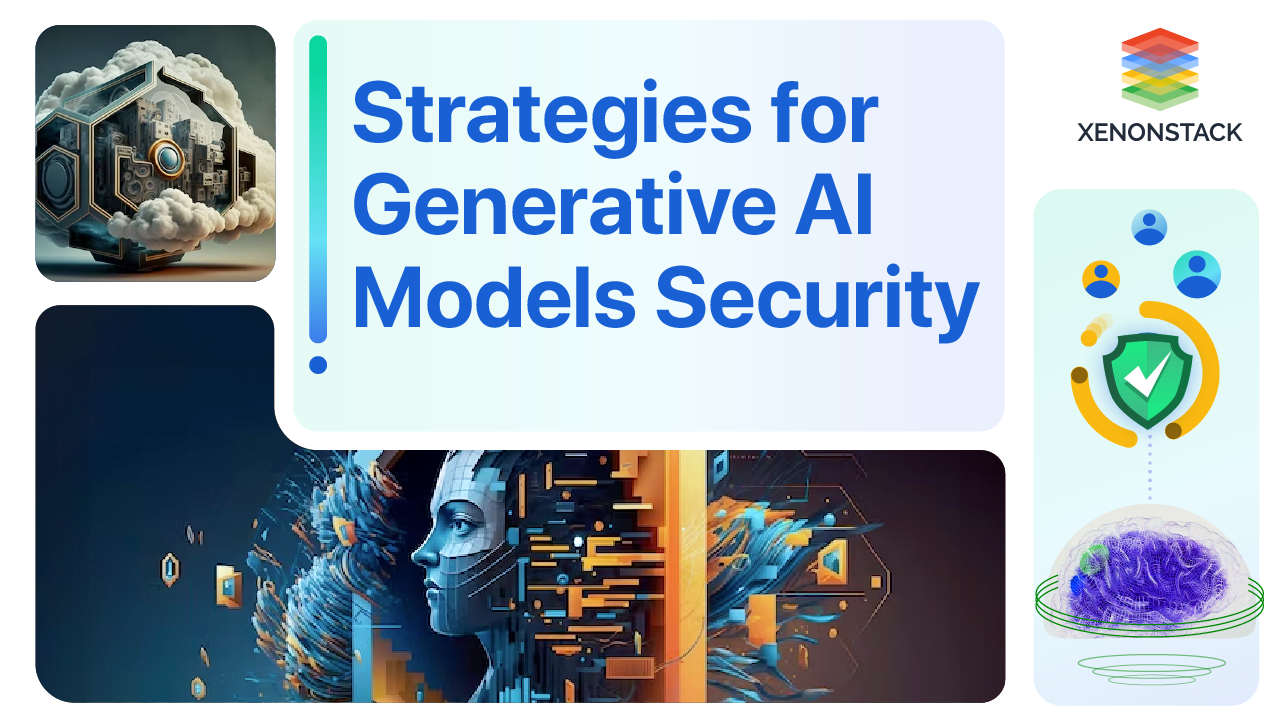
1. Privacy
-
Data Protection: AI systems often process vast amounts of personal data. Ensuring this data is kept secure from unauthorized access or leaks is crucial for maintaining individual privacy.
-
Informed Consent: It is important for users to be informed about the data that is being collected and how it will be used. This respects their autonomy and allows them to make informed decisions about their participation.
2. Surveillance
-
Abuse of AI for Surveillance: There exists a possibility that AI might be employed to augment surveillance capabilities, thereby potentially encroaching upon the liberties and privacy rights of individuals.
-
Transparency and Regulation: Clear regulations and transparency are crucial for governing the use of AI in surveillance, preventing potential misuse, and safeguarding individuals' fundamental rights.
3. Accountability
-
Responsibility for AI Actions: It is of utmost importance to ascertain the individual or entity accountable for the actions carried out by an AI system, particularly in cases involving security breaches or misuse.
-
Auditing and Oversight: Regular audits and oversight mechanisms can ensure AI systems are used ethically and by established norms and laws.
4. Implications of Inadequate Security
-
Misuse of AI: Insecure AI systems can be exploited for malicious purposes, from spreading misinformation to conducting cyber-attacks.
-
Loss of Public Trust: Frequent compromises of AI systems can greatly diminish trust in their potential advantages.
5. Societal Impact
-
Economic Disruptions: Security breaches in AI systems can have significant financial repercussions, affecting businesses and consumers.
-
Social Manipulation: Unsecured AI could manipulate public opinion or interfere in democratic processes.
6. Ethical Guidelines for AI Development and Use
-
Principles of Ethical AI: Establishing principles such as fairness, transparency, non-maleficence, and accountability is vital.
-
Participatory Design: Involving stakeholders, including marginalized groups, in the AI design process ensures diverse perspectives and needs are considered.
-
Continuous Ethical Education: AI developers and users should be continuously educated on the ethical implications of AI, ensuring they remain aware of evolving challenges and standards.
7. Global Collaboration
-
International Standards and Regulations: Collaboration at an international level is necessary to establish and enforce standards for AI security and ethical use.
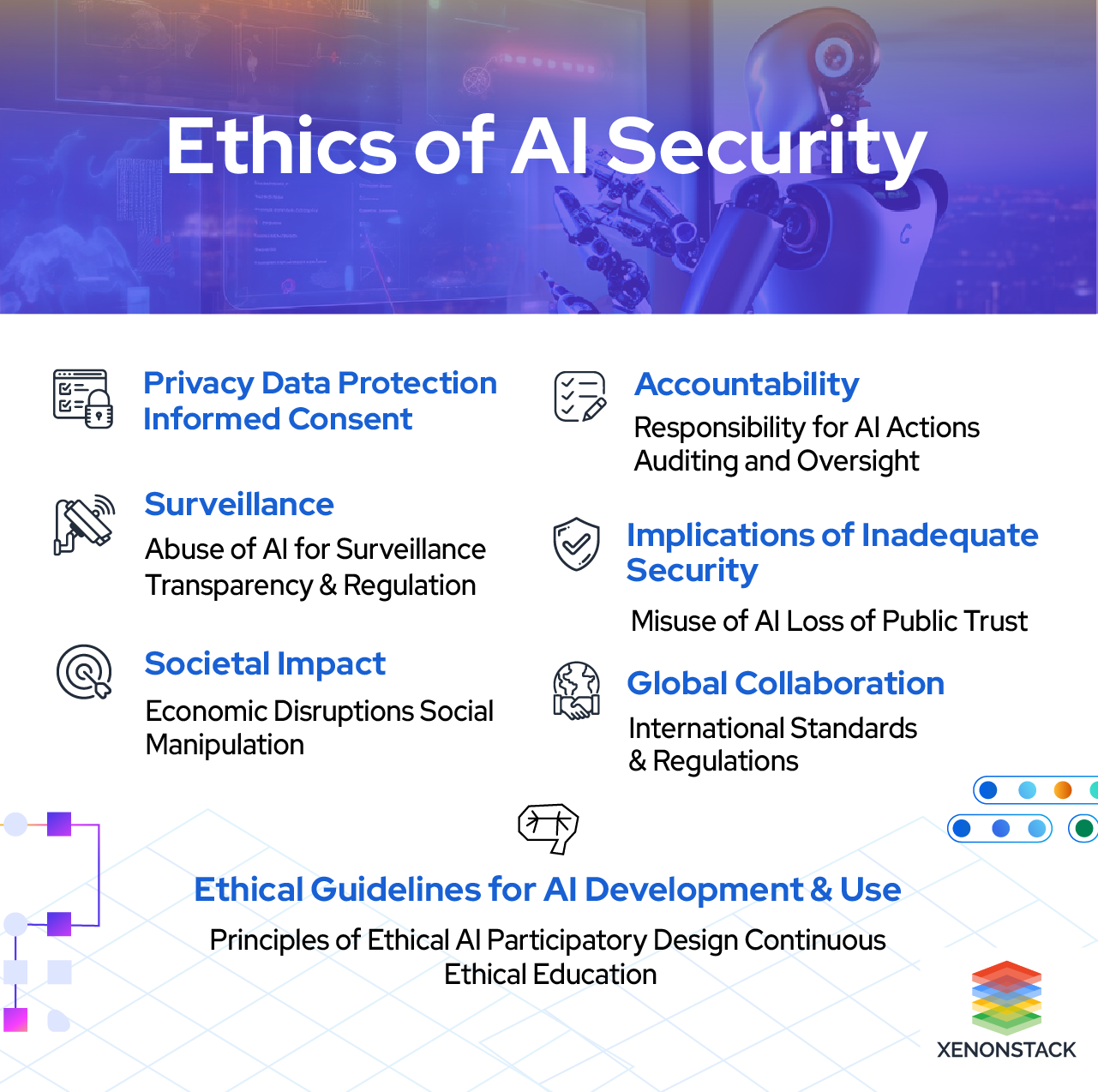
Securing AI Models in Healthcare
Integrating AI models in healthcare presents unique security challenges, balancing the need for innovation with stringent security and ethical standards. Here's an exploration of these challenges, the importance of data protection, ensuring model accuracy and reliability, potential benefits, and strategies for maintaining this balance
1. Protecting Patient Data
-
Data Sensitivity: The protection of healthcare data is crucial, as it often contains sensitive personal information. It's important to ensure its security and safeguard the privacy of individuals.
-
Compliance with Regulations: Healthcare professionals must comply with strict data protection laws in the U.S., like HIPAA, which set high standards for handling patient data.
2. Ensuring AI Model Accuracy and Reliability
-
Model Validation: AI models in healthcare must be rigorously validated for accuracy, given that errors can have severe consequences for patient health.
-
Bias and Fairness: Models must be tested for bias to ensure they work effectively across diverse patient populations and not inadvertently perpetuate health disparities.
3. Potential Benefits of AI in Healthcare
-
Improved Diagnostics and Treatment: GenerativeAI analyze medical data to identify patterns not easily seen by humans. This can result in upgrade diagnostic precision and the development of personalized treatment strategies.
-
Operational Efficiency: AI has the potential to optimize administrative tasks, allowing healthcare professionals to dedicate a greater amount of time and attention to patient care.
-
Advancements in Research: AI has the potential to expedite medical research by swiftly analyzing extensive datasets, resulting in expedited discoveries and advancements.
4. Security Challenges
-
Data Breaches: The healthcare industry's reliance on AI systems to process sensitive data makes them a prime target for cyber-attacks.
-
Model Tampering: There's a risk that AI models could be tampered with, leading to incorrect diagnoses or treatment recommendations.
5. Balancing Security with Innovation
-
Robust Security Guidelines: By Executing robust security measures like encoding and access controls protect data.
-
Ethical AI Frameworks: Instituting ethical frameworks for AI in healthcare ensures balancing tech progress with patient safety.
-
Stakeholder Collaboration: Partnership among healthcare providers, AI developers, regulatory bodies, and patients is vital for understanding potential risks and establishing trust.
6. Continuous Monitoring and Adaptation
-
AI systems in healthcare should be continuously monitored for performance, security vulnerabilities, and ethical implications, adapting as necessary to maintain safety and efficacy.
7. Public and Professional Education
-
Educating the public and healthcare professionals about the capabilities and limitations of AI in healthcare can help manage expectations and encourage responsible use.
-
Addressing these challenges and focusing on security, privacy, and ethical considerations, AI in healthcare can be harnessed to its full potential, leading to significant improvements in patient care, efficiency, and medical research.
Conclusion
In conclusion, securing generative AI models from adversarial attacks and ensuring their ethical application, particularly in sensitive fields like healthcare, is a complex but essential task. The strategies discussed in this blog highlight the multi-faceted approach required to protect AI models from security threats while adhering to ethical standards.
-
Discover here Generative AI in Customer Experience
-
Explore here the Future of Generative AI for Enterprises