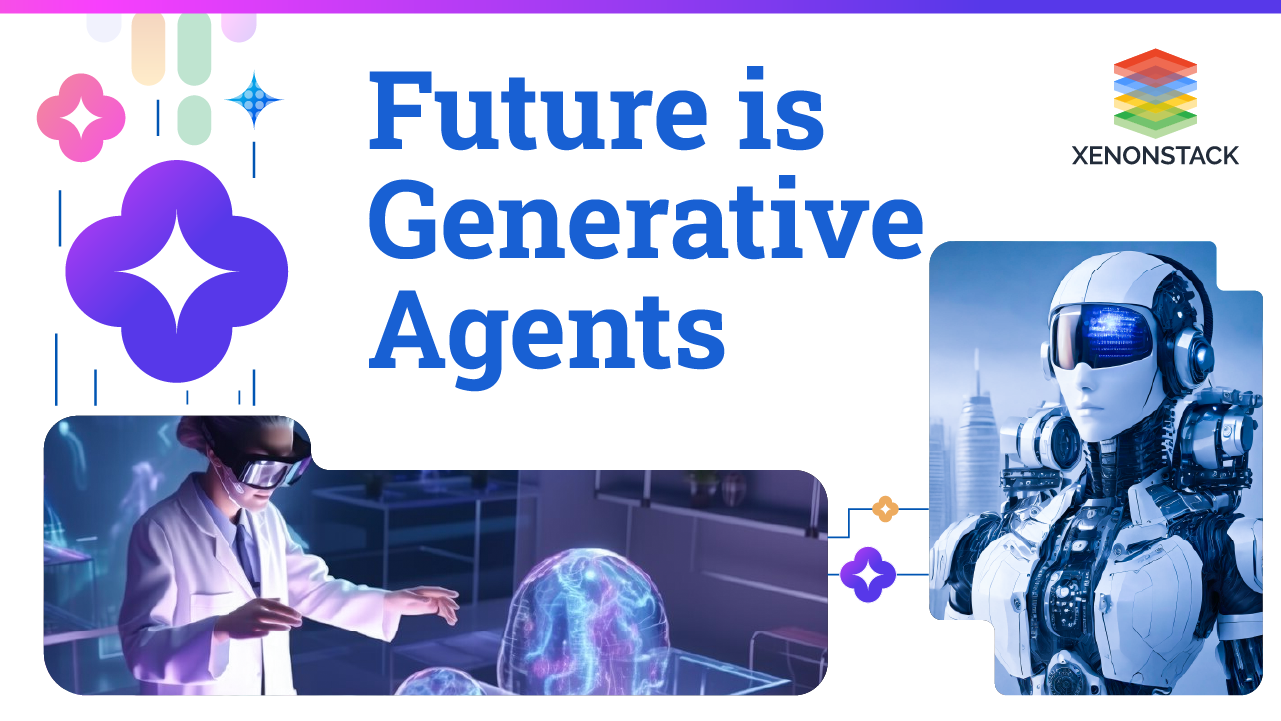
Applications Across Industries
The potential applications of generative agents span across various industries, each leveraging their unique capabilities to drive progress and innovation.
-
Art and Entertainment: Generative agents are revolutionizing the way we create and consume content in the world of art and entertainment. From creating life-like paintings and original music to creating immersive virtual worlds, creative agents are pushing the limits of creativity and providing new opportunities for artistic expression.
-
Healthcare: Generative agents are changing the way we view medical imaging and drug discovery, as well as patient care. Generative agents analyze enormous amounts of medical information to help physicians diagnose diseases, predict treatment results, and even create personalized treatment plans for specific patients.
-
Gaming: Generative agents are also making an impact in the gaming industry. Generative agents can be used to create realistic environments and NPCs and engaging gameplay mechanics. Generative agents are not only improving the gaming experience, but they are also providing game developers with new ways to innovate.
-
Content Creation: Generative agents have the capability to automate content creation across various platforms, including social media, marketing, and journalism channels. Generative agents can analyze user preferences and trends to create personalized content at scale. This allows businesses to better engage with their audience and drive growth.
-
Education: Generative agents (GAs) are changing the way students learn and interact with course material. GAs builds interactive simulations and provide virtual tutors that provide personalized learning experiences based on learner learning styles and help students gain a better understanding of complex topics.
Ethical and Societal Implications
While the potential of generative agents is vast, their proliferation also raises important ethical and societal considerations that must be addressed.
-
Bias and Fairness: Like all AI systems, generative agents are susceptible to bias, which can perpetuate existing inequalities and discrimination. It is crucial to ensure that these agents are trained on diverse and representative datasets to mitigate bias and promote fairness in their output.
-
Privacy and Security: Generative agents raise concerns about privacy and security, especially when they are used to generate sensitive information or manipulate digital content. Safeguards must be put in place to protect against misuse and unauthorized access to these agents.
-
Intellectual Property: Generative agents also raise questions about intellectual property (IP) rights and who owns the content created. Clear guidelines and regulations are needed to govern the ownership and use of content produced by these agents to prevent disputes and ensure fair compensation for creators.
-
Impact on Employment: As generative agents become more prevalent, there is growing concern about their impact on employment, particularly in industries where manual labor or creative work is involved. It is essential to consider the potential displacement of workers and explore ways to retrain and upskill individuals for new roles in the AI-driven economy.
The Path Forward
Despite these challenges, the future of generative agents holds immense promise for advancing technology and shaping the world we live in. To realize this potential, it is essential to adopt a collaborative and responsible approach to AI development and deployment.
-
Ethical AI Design: Developers must prioritize ethical considerations in the design and implementation of generative agents, ensuring transparency, accountability, and fairness in their operation.
-
Education and Awareness: There is a need for greater education and awareness about AI and its implications among the public, policymakers, and industry stakeholders. By fostering a better understanding of AI technologies, we can navigate the ethical and societal challenges they present more effectively.
-
Regulation and Governance: Governments and regulatory bodies are pivotal in shaping the ethical and legal landscape of AI. Strong regulations and governance structures are essential to responsibly develop and deploy generative agents, while mitigating potential risks.
-
Collaboration and Innovation: Collaboration between academia, industry, and civil society is essential for driving innovation and addressing the complex challenges associated with generative agents. By working together, we can harness the full potential of AI to create a better future for all.
Conclusion
In conclusion, the future is indeed generative agents, AI systems capable of creating new and valuable content across a wide range of domains. With their ability to generate diverse and realistic output, these agents have the potential to revolutionize industries, drive innovation, and shape the world we live in. Yet, unlocking this potential demands a united commitment to tackle ethical, societal, and technical hurdles, ensuring the responsible development and deployment of generative agents. Embracing opportunities and surmounting challenges, we can unleash the power of generative agents, shaping a future that is inclusive, equitable, and prosperous for humanity.
-
Click to explore about Intelligent Robotic Process Automation
- Explore more about How to Build a Generative AI Model for Image Synthesis