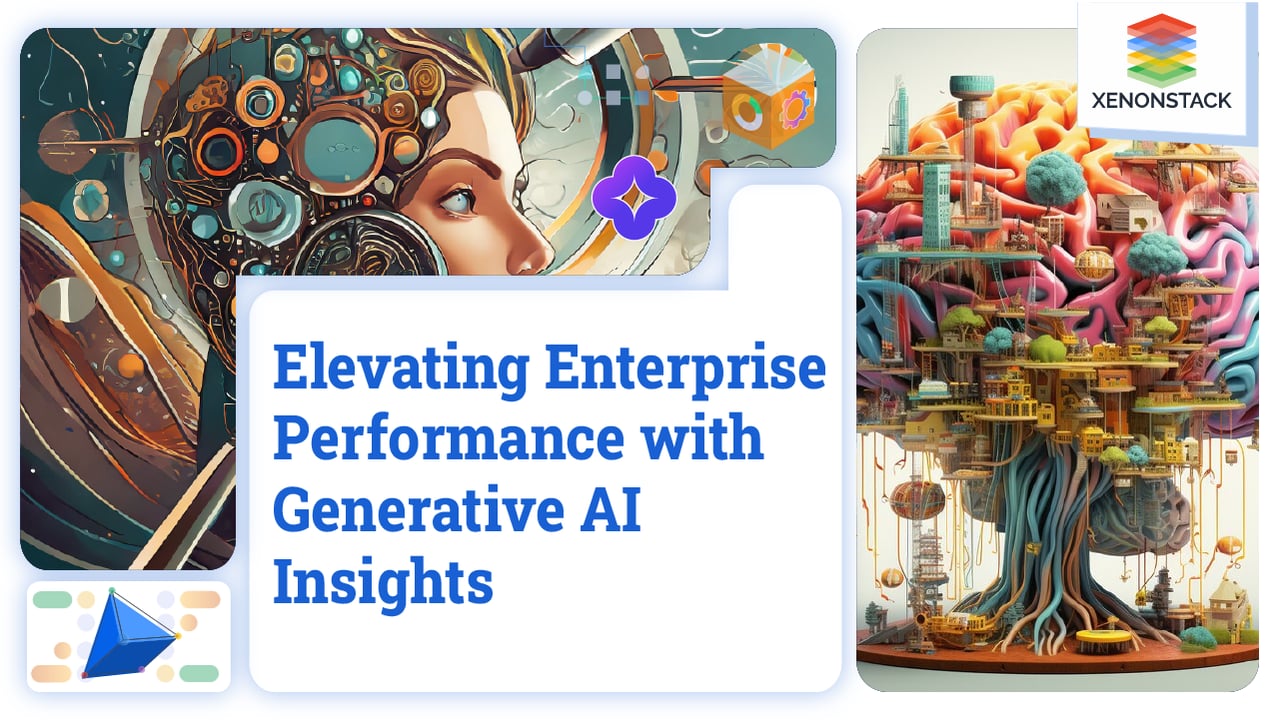
Introduction
Generative AI has numerous benefits. It enables the generation of realistic images, videos, and audio. This technology can also help create innovative designs and ideas by analyzing existing patterns and developing new ones. Generative AI is useful in various fields, including art, music, fashion, and video game development. Additionally, it can automate specific tasks, improving efficiency and reducing costs. Despite these benefits, there must be more enterprise use cases as companies are still exploring ways to utilize emerging technologies to enhance their operations. However, as more businesses recognize the potential benefits of AI, Machine Learning (ML), and other advanced technologies, we may see more enterprise use cases emerge.
Challenges of Implementing Generative AI in Enterprise
The following are the main issues that organizations often face:
-
Slow decision-making process
-
Poor quality data
-
Misaligned incentives
-
Hesitancy to touch complex ERP applications
Enterprises, or large-scale organizations, often have complex structures and decision-making processes. This can result in slow progress, with even simple decisions taking 6-9 months to make. Moreover, enterprise data can be fragmented, scattered across different systems and departments, and difficult to integrate, making it challenging to execute GenAI initiatives. Implementing artificial intelligence solutions often requires significant data preparation and training, which can be time-consuming and costly.
To make matters worse, incentives can be misaligned within enterprises. Data centres, for example, may hold significant power and resist change, hindering the widespread adoption of AI solutions. However, AI has the potential to democratize the entire process by cutting through silos and aligning incentives. By using AI to integrate data across departments and systems, organizations can break down silos and create a shared understanding of their data. This can lead to more efficient decision-making processes and better alignment of incentives across different departments.
Potential of Generative AI in Enterprise
To execute GenAI initiatives, good data is crucial, and preparing and training the data is a complex task that requires collaboration among data centres. AI has the potential to democratize the entire process by removing silos and aligning incentives to improve the decision-making process. By leveraging AI, enterprises can expedite their decision-making processes and gain accurate insights and analysis.
To overcome the hesitancy to touch complex ERP applications, employees can be trained to use the applications more efficiently, and companies can invest in tools and training to streamline the processes. Furthermore, aligning incentives can create a sense of ownership among employees, increasing their motivation to work collaboratively and improve their performance.
Enterprises can overcome challenges and improve decision-making processes, data quality, and performance by adopting constructive approaches like peripheral applications, AI, training, and aligning incentives.
Flaws in current Generative AI technology
Numerous challenges and concerns are associated with current Generative AI technology. One issue is the potential for hallucination, where the AI generates content that is not accurate or representative of reality. Another concern is prompt injections, which can occur when individuals reverse engineer prompts and manipulate the model to produce desired results. Privacy and security issues are also a concern, as sensitive information could be inadvertently revealed through the AI's output. Finally, the cost and return on investment for long gestation applications can be a barrier to adoption for some organizations. These are all critical factors to consider when working with Generative AI technology.
Adoption Curve for Generative AI
Here is the technological adoption and innovation timeline, starting with early adopters like Klarna and predicting that B2B consumer tech companies will follow in their footsteps. The factors that impact technology adoption include accuracy, cost, privacy and security, data preparation, and enterprise focus.
The "Crossing the Chasm" model illustrates the different categories of adopters, including Innovators, Startups, Early Majority, Late Majority, and Laggards. The timeline presented in the model predicts that Innovators and Startups will lead the way until 2023-2024, followed by the Early Majority. Late Majority will adopt the technology by late 2025 or early 2026, and finally, Laggards will adopt the technology, marking the end of the adoption phase.
Challenges to Mainstream Enterprise Adoption
Several challenges must be addressed to achieve mainstream enterprise adoption. Accuracy is critical, as businesses require reliable and trustworthy data to make informed decisions. Cost is also a concern, as implementing new technologies and processes can be expensive. Privacy and security are also critical, as companies must protect sensitive data from unauthorized access or theft. Finally, data preparation can be a significant challenge, as it requires cleaning, formatting, and organizing data to make it usable for analysis. By addressing these challenges, businesses can improve the accuracy and reliability of their data, reduce costs, protect sensitive information, and ensure that their data is ready for analysis and decision-making.
Generative AI can break into mainstream enterprises by addressing organizations' key challenges when implementing new technologies. To achieve mainstream adoption, Generative AI must improve accuracy, reduce costs, ensure privacy and security, simplify data preparation, and align with enterprise goals.
To improve accuracy, Generative AI must deliver reliable and trustworthy data to support informed decision-making. This can be achieved through rigorous testing, training, and validation processes. To reduce costs, Generative AI must offer scalable and cost-effective solutions that deliver a significant return on investment.
In order to safeguard privacy and security, Generative AI should incorporate strong security measures, including encryption and access controls, to safeguard sensitive data. Generative AI must simplify data preparation by automating data cleaning, formatting, and organization processes. Finally, Generative AI must align with enterprise goals by focusing on specific use cases that deliver measurable business value.
Addressing these challenges, Generative AI can break into mainstream enterprises and revolutionize various industries.
Conclusion
Generative AI has enormous potential in various fields, including art, music, fashion, and video game development. It can automate tasks, improve efficiency, and reduce costs, making it a valuable technology for enterprises. However, organizations must overcome several challenges before mainstream enterprise adoption can occur. These challenges include accuracy, cost, privacy and security, data preparation, and enterprise focus. By addressing these issues and leveraging AI to integrate data across departments and systems, organizations can break down silos, create a shared understanding of their data, and expedite decision-making processes. With the democratization of the entire process, enterprises can gain accurate insights and analysis and improve their performance.
-
Read more about Generative AI for Infrastructure Management
-
Know more about Generative Adversarial Network Architecture
-
Explore more about How to Build a Generative AI Model for Image Synthesis