Agentic AI, characterized by its autonomous decision-making capabilities, transforms industries by executing tasks without direct human intervention. While this advancement brings efficiency and innovation, it also presents significant cybersecurity challenges. From adversarial attacks to data breaches, organizations must address these threats to ensure the secure deployment of agentic AI systems.
This blog explores agentic AI's key cybersecurity challenges, real-world implications, and effective mitigation strategies.
Understanding Agentic AI and Its Security Implications
Agentic AI refers to AI systems that operate independently, making decisions and taking actions based on predefined goals and learned experiences. Unlike traditional AI models that rely on human supervision, agentic AI systems can interact dynamically with their environment, adapt to changes, and make autonomous choices.
This independence, however, exposes agentic AI to unique security risks, including:
Adversarial Attacks – Malicious inputs designed to deceive AI models.
Data Poisoning – Manipulation of training data to corrupt AI decision-making.
Model Theft and Reverse Engineering – Unauthorized access to AI architectures.
Bias Exploitation – Manipulation of AI bias to generate undesirable outcomes.
Autonomy Exploitation – Hacking AI’s autonomous decision-making processes.
Supply Chain Attacks – Targeting AI components and data sources before deployment.
Cybersecurity Challenges in Agentic AI
1. Adversarial Attacks
Adversarial attacks involve subtly altering inputs to trick AI models into making incorrect predictions. Attackers leverage small perturbations that humans cannot detect but AI misinterprets, leading to severe consequences in security-sensitive applications like autonomous vehicles, surveillance systems, and fraud detection systems.
Real-World Example:
-
Researchers demonstrated how slight alterations in stop signs could make autonomous vehicles misinterpret them as speed limits, leading to potential road hazards.
Solution:
-
Implement adversarial training to expose AI to potential attacks.
-
Use robust anomaly detection techniques to identify suspicious inputs.
-
Deploy model ensembling to make AI more resilient to adversarial perturbations.
-
Integrate AI-powered cybersecurity solutions to detect adversarial attacks in real-time.
2. Data Poisoning Attacks
In data poisoning, attackers inject malicious data into AI training datasets, altering the model’s behaviour. This can lead to biased decision-making, reduced accuracy, or intentional vulnerabilities within AI systems.
Real-World Example:
-
Poisoning an AI-powered email filter to incorrectly classify phishing emails as safe leads to widespread security breaches.
Solution:
-
Ensure data integrity by employing blockchain and decentralized verification.
-
Continuously audit datasets for anomalies and inconsistencies.
-
Use differential privacy to minimize exposure of training data to potential adversaries.
-
Develop AI models with self-healing capabilities that recognize and correct poisoned data.
3. AI Model Theft and Reverse Engineering
As AI models become valuable assets, attackers attempt to steal proprietary models through side-channel attacks, model extraction techniques, and API exploitation. This theft allows adversaries to maliciously deploy similar AI models or use them to find vulnerabilities.
Solution:
-
Implement API rate limiting and access control measures.
-
Utilize encryption techniques such as homomorphic encryption to secure AI computations.
-
Apply watermarking techniques to identify unauthorized usage of proprietary models.
-
Store AI models in secure enclaves that prevent unauthorized access.
4. Bias and Ethical Manipulation
Agentic AI models are prone to inherent biases that, when exploited, can lead to unfair or unethical decisions. Attackers can intentionally manipulate biases in AI to serve malicious objectives, leading to reputational and financial damage.
Real-World Example:
-
AI-driven hiring tools discriminate against specific demographic groups due to biased training data.
Solution:
-
Regularly audit AI models for biases and fairness.
-
Deploy explainable AI (XAI) to make AI decision-making more transparent.
-
Establish regulatory compliance frameworks to prevent biased AI exploitation.
-
Implement diversity-driven training datasets to mitigate AI bias from the outset.
5. Autonomy Exploitation and AI Manipulation
Autonomous AI systems, particularly those operating in mission-critical sectors, can be manipulated to make harmful decisions. Attackers can hijack AI agents by injecting malicious commands or modifying their decision-making algorithms.
Real-World Example:
-
Attackers exploit vulnerabilities in AI-powered drones to change flight paths or disrupt operations.
Solution:
-
Implement multi-layer authentication for AI command execution.
-
Use reinforcement learning with human oversight to prevent unintended AI actions.
-
Develop AI fail-safe mechanisms to halt operations in case of anomalies.
-
Introduce runtime monitoring to detect and counteract AI manipulation in real time.
6. Supply Chain Attacks on AI Systems
AI systems rely on extensive supply chains, including data sources, model training environments, and hardware components. Attackers can introduce vulnerabilities at any stage, compromising AI systems before deployment.
Solution:
-
Verify the integrity of AI supply chain components.
-
Employ end-to-end encryption for AI model updates and data transfers.
-
Conduct thorough vetting of third-party AI providers to ensure security compliance.
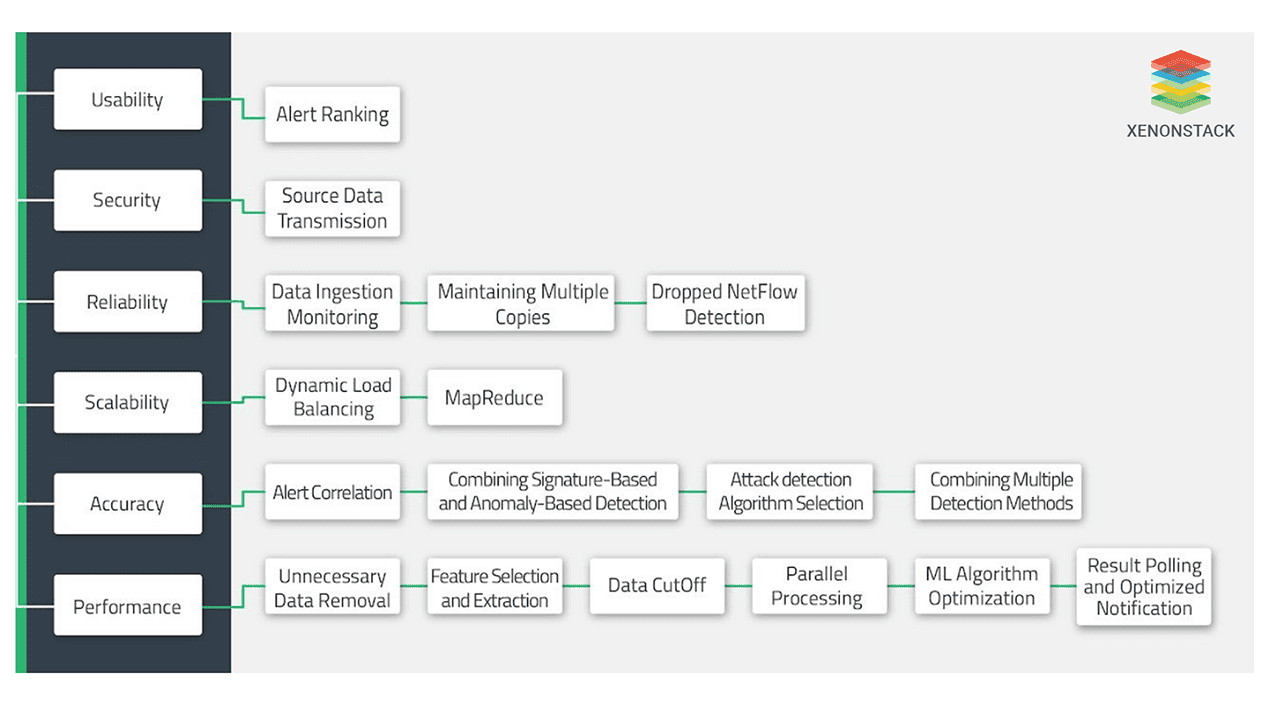
Strategies for Strengthening Cybersecurity in Agentic AI
1. Zero Trust Security Model
Adopt a zero-trust architecture where no entity (user, device, or AI model) is inherently trusted. Continuous verification and strict access control prevent unauthorized AI interactions.
2. Federated Learning for Secure AI Training
Federated learning allows AI models to be trained on decentralized data without centralizing sensitive information, reducing the risk of data breaches.
3. AI Explainability and Transparency
Enhancing AI interpretability ensures that security teams can track AI decision-making patterns and detect abnormalities before they escalate into security threats.
4. Regular Security Audits and Red Teaming
Conduct penetration testing, security audits, and red teaming exercises to identify vulnerabilities in agentic AI systems proactively.
5. Regulatory Compliance and Ethical Governance
Establish governance policies that ensure AI systems comply with global cybersecurity standards such as GDPR, NIST, and ISO 27001.
6. AI Model Resilience Techniques
-
Employ self-learning AI models that continuously update their security mechanisms.
-
Integrate cyber deception techniques to mislead attackers attempting to compromise AI systems.
-
Deploy AI-enhanced threat intelligence to predict and mitigate cyber risks proactively.
Conclusion of Cybersecurity Challenges in Agentic AI
Agentic AI introduces groundbreaking advancements and presents new cybersecurity challenges that organizations must address. Implementing robust security frameworks, adversarial resilience techniques, and ethical AI governance can ensure the safe deployment of agentic AI. As AI evolves, a proactive approach to cybersecurity will mitigate risks and securely unlock AI’s full potential. Organizations must invest in AI security research, collaboration, and threat intelligence sharing to build a future where AI operates securely and ethically.
Next Steps with Agentic AI
Talk to our experts about implementing compound AI system, How Industries and different departments use Agentic Workflows and Decision Intelligence to Become Decision Centric. Utilizes AI to automate and optimize IT support and operations, improving efficiency and responsiveness.