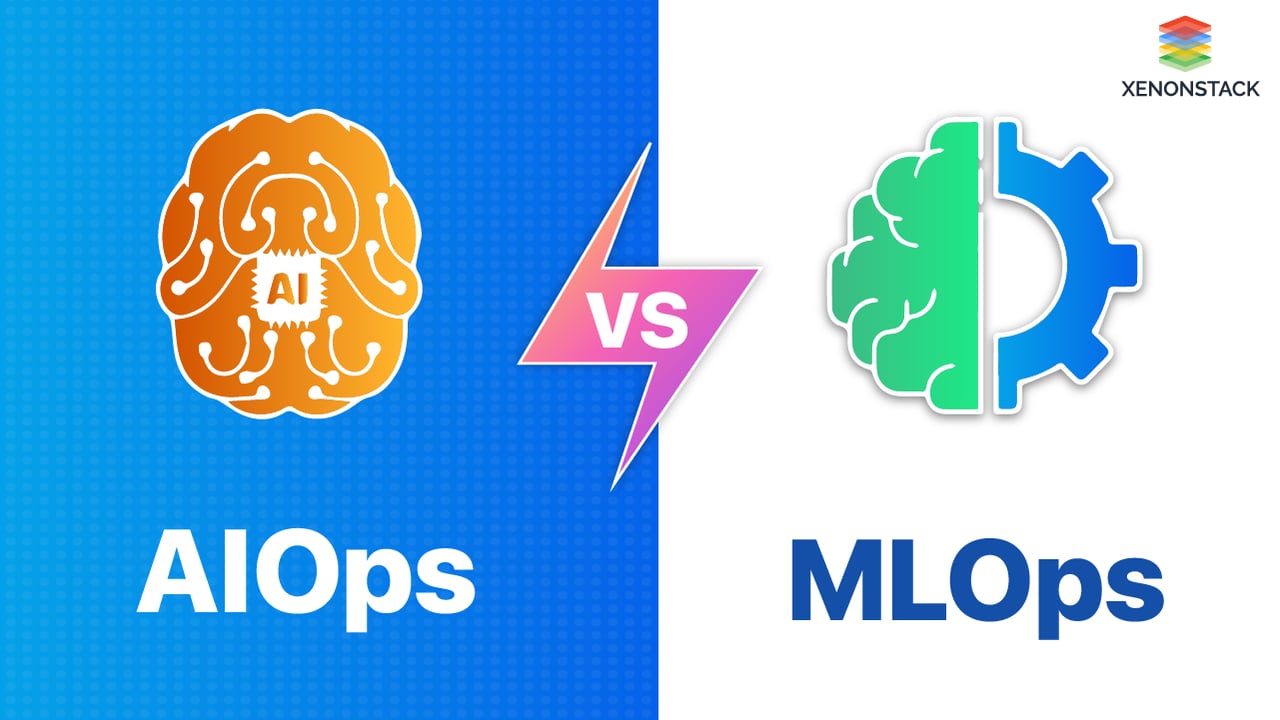
Introduction to AIOps and MLOps
The goals of MLOps (Machine Learning Operations) and AIOps (Artificial Intelligence for IT Operations), two similar but separate technologies, are to improve the effectiveness and efficiency of the processes involved in developing machine learning and IT operations, respectively.
AIOps is a field that automates and optimizes IT operations processes, including managing risk, event correlation, and root cause analysis using Artificial Intelligence (AI) and Machine Learning (ML) techniques. It aim to reduce the time and effort needed for manual IT processes while increasing the precision and speed of problem-solving.
MLOps, on the other hand, is a set of practices and tools for managing the entire lifecycle of machine learning models, from development to deployment to production. This includes data preparation, model training, validation, monitoring, and managing model versioning and deployment. It aims to speed up the time-to-market for machine learning models and ensure that they are reliable and scalable in production environments.
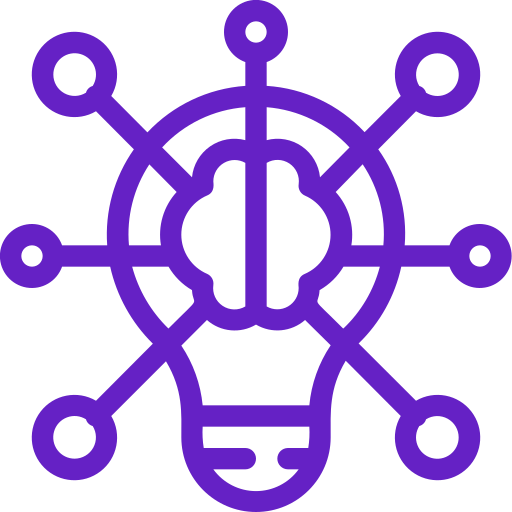
AIOPS aim to reduce the time and effort needed for manual IT processes while increasing the precision and speed of problem-solving
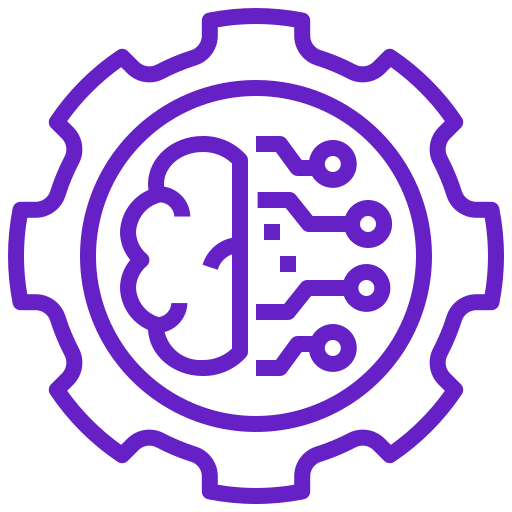
MLOPS aims to speed up the time-to-market for machine learning models and ensure that they are reliable and scalable in production environments
In summary, AIOps focuses on improving IT operations' efficiency through AI and ML. In contrast, MLOps focuses on improving the efficiency of machine learning development processes using DevOps principles and practices.
Leverage AIOps for enhanced automation and faster execution of processes in enterprises. Click to explore about our, Artificial Intelligence for IT Operations
What is AIOps?
AI for IT operations, or AIOps, is a field of study that automates and improves IT operations processes using machine learning (ML) and artificial intelligence (AI) approaches. Its purpose is to decrease the time and effort needed for manual IT operations while increasing IT operations' efficiency and efficacy, including incident management, event correlation, and root cause analysis.
In real life, AIOps involve collecting, analyzing, and applying AI and ML algorithms to find patterns and trends, automate manual operations, and improve the efficiency and accuracy of issue-solving. To aid IT operations teams in making wise decisions and resolving problems more rapidly, systems also offer real-time insights and suggestions.
Automating and optimizing IT operations procedures may assist enterprises in reducing downtime, enhancing service quality, and increasing the efficiency and effectiveness of their IT operations.
The Goal of AIOps
The goal is to provide continuous insight across IT operations, including the following:
- Anomaly detection
- Outlier detection
- Malware traffic detection
- Vulnerability detection
- Historical analysis
- Performance analysis
- Root cause analysis
- Remediation
The next generation of IT operation analytics ot integrates a huge amount of data and uses machine learning to automate IT processes. Taken From Article, How to Integrate AIOps in DevOps?
What is MLOps?
MLOps is "a method for data scientists and operations experts to collaborate and communicate to manage the production ML (or deep learning) lifecycle. It aims to improve automation and raise the quality of production machine learning while simultaneously focusing on business and regulatory needs, much like the DevOps or DataOps methodologies.
It is the collection of engineering components that regularly work together to deploy, run, and train AI models. Using that, we can see that it consists of three ability to enable parts:
- Machine Learning
- DevOps (IT)
- Data Engineering
Each component plays a crucial role in helping an organization complete the ML lifecycle. Initially, MLOps emerged to improve communication between data scientists and DevOps teams working with machine learning. It began as simple workflows and procedures aimed at managing the challenges encountered during ML implementations. Over time, it has evolved into a comprehensive framework for overseeing the entire lifecycle, covering stages such as data collection, model building (integrating the software development lifecycle and continuous integration/delivery), orchestration, deployment, health monitoring, diagnostics, governance, and business KPIs
The key phases of MLOps start with Data gathering, analysis, transformation/preparation, Model training and development, validation, serving, monitoring, and re-training.
It provides a clear direction and focuses on corporate interests for data scientists with measurable benchmarks. Some of the critical capabilities that enable machine learning in production include:
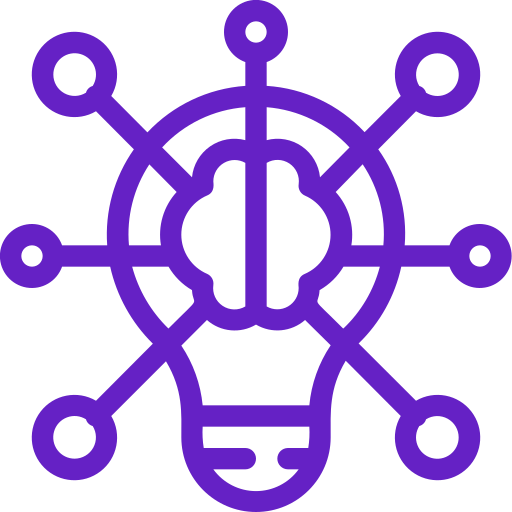
Simplified Deployment
The deployment process can become intricate when data scientists use various modeling frameworks, languages, and tools. MLOps enables IT operations teams to deploy models from diverse frameworks and languages in production environments effectively
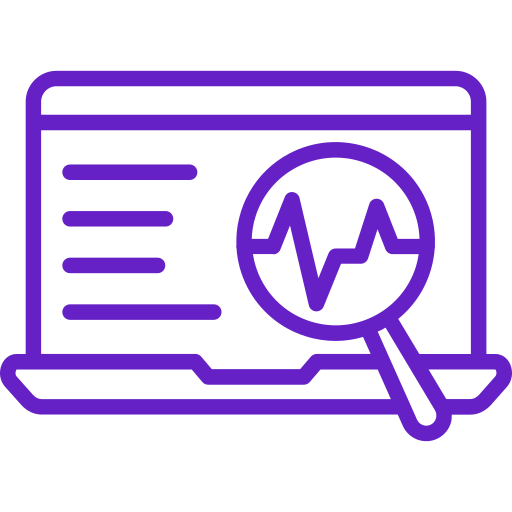
ML Monitoring
Traditional software monitoring tools are inadequate for machine learning monitoring. MLOps offers specialized monitoring, providing metrics tailored to each model, identifying data drift in critical features, and including other essential functionalities
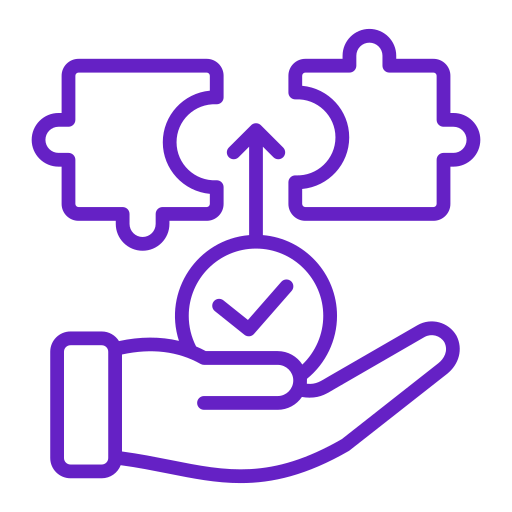
Lifecycle Management
Deployment marks only the initial phase of a lengthy update lifecycle. To maintain an operational machine learning model, teams must test the model and its updates without disrupting business applications, which falls within the MLOps domain
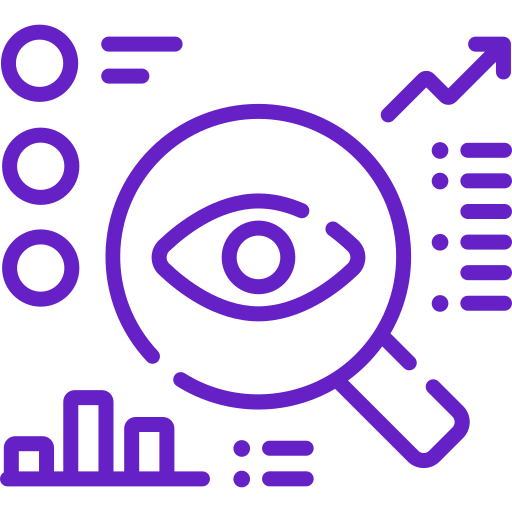
Compliance
MLOps promotes compliance by offering traceability, access control, and audit trails. This framework reduces risks, prevents unauthorized changes, and ensures adherence to regulatory standards, ultimately enhancing the integrity and security of machine learning operations
Automating Machine Learning Operations means automation and monitoring at all ML system construction steps. Taken From Article, MLOps Challenges and Solutions
Differences Between AIOps and MLOps
The areas of Artificial Intelligence Operations and Machine Learning Operations, which concentrate on the operational elements of AI/ML systems, are similar but separate. The main difference between AIOps and MLOps is the scope of focus:
AIOps |
MLOps |
Focus on infrastructure, data management, model deployment, monitoring, and maintenance |
MLOps focuses exclusively on the operational elements of machine learning models |
Uses big data and ML to automate IT activities |
Produces machine learning pipelines, also known as DevOps for ML. |
Allow for more rapid and accurate root cause analysis |
Enabling strong governance and ongoing machine learning model improvement |
Enabling preventative maintenance, automating issue resolution and providing proactive alerts. |
Model validation reduces bias and ensures fairness |
Manages vulnerability risks continually |
Manages ML lifecycle |
Insights into factors other than the obvious |
Enables collaboration between data engineering and data science teams |
Use Cases of AIOps and MLOps
Real-world examples of organizations using AIOps and MLOps to solve IT and ML-related problems.
Real-life use cases of MLOps
- Web Analytics – Coinbase, AT&T
- Systems for providing recommendations - OTT and e-commerce platforms - a recommendation engine that derives from user actions and reproduces those actions. In this situation, keeping an eye on the forecasts is crucial to preventing a domino effect.
- Share Market Analysis – Bloomberg
- Sports Analysis – ESPN, Sky Sports
Real-life use cases of AIOps
- Predictive Alerting: Place Park Technologies and TDC NetDesign
- Avoiding Service Disruptions: Schaeffler Group
- Proper Monitoring of System: Enablis
- Blueprinting and Triaging of Incidents: PostNord AB
An ML engineering practice that aims to unite Machin Learning system development and ML system operation. Taken From Article, Challenges of Productionizing ML models
What are the Advantages of MLOps?
MLOps is focused on developing scalable ML systems, as was already indicated. Let's discuss how it differs from the conventional method of constructing ML Systems and the significance of MLOps.
Orchestration of multiple pipelines
Developing a machine learning model combines several processes (pre-processing, feature engineering model, model validation, etc.). You may orchestrate these workflows with the help of MLOps to update the model automatically.
Managing ML Lifecycle
Model development has several stages, and traditional DevOps might require a lot of work to manage and maintain. However, it gives you the advantage of deploying and quickly improving ML models in production.
Scale ML Applications
The real problem arises as use and significant data increase and ML applications fail. Scaling ML applications is possible with MLOps as and when demand rises.
Monitor ML systems
Monitoring the system's performance is essential following the deployment of machine learning models. By enabling the detection of model and data drifts, MLOps offer approaches.
Continuous Integration and Deployment
Continuous integration and deployment are techniques DevOps use in the software development process, but applying them to the creation of ML systems is challenging. Machine learning systems may be effectively deployed using CI and CD thanks to the many tools and methodologies presented by MLOps.
Developing a machine learning mode and deploying it fast and cheaply but maintaining it over time becomes difficult. Click to explore about our, What are the MLOps best practices?
What are the Advantages of AIOps?
AIOps has different use cases and benefits from MLOps as it leverages Machine learning techniques to improve IT Operations.
Proactive IT Operations
Customer satisfaction is crucial for the success of products and services in a market that is competitive. It is essential to anticipate if a failure will occur rather than just reacting to a problem. The ability of IT Operations to foresee and address problems with applications, systems, and infrastructure is crucial.
Data-driven decision-making
Pattern Matching, Historical Data Analysis, and Predictive Analysis are ML approaches used in AIOps in IT Operations. These machine-learning approaches will eliminate human mistakes by making decisions based only on data. IT operations can concentrate on resolution rather than root cause analysis with the help of such an automated reaction.
Detecting anomalies and deviation from baseline
IT Operations can identify odd behavior using ML approaches like clustering. These monitoring methods, which may be used to change firewall rules and detect anomalies in network traffic automatically, are developed with the help of AIOps.
Enterprises need compositional AI with Explainability for Data-Centric transformation. Click to explore about our, Artificial Intelligence Services Development Company
Conclusion
Two essential strategies—AIOps and MLops—may be used to manage the development and deployment of AI and machine learning systems. MLops focuses on improving and controlling the entire machine learning life cycle, while AIOps focuses on automating the operations of AI systems. Both strategies must be incorporated into an organization's AI and machine learning development and deployment process to produce dependable, scalable, and effective AI and machine learning systems.
Read here to Streamline ML Projects with MLOPs and Azure ML Know more about Top 7 Layers of MLOps Security Explore here Best Open Source AIOps Platforms