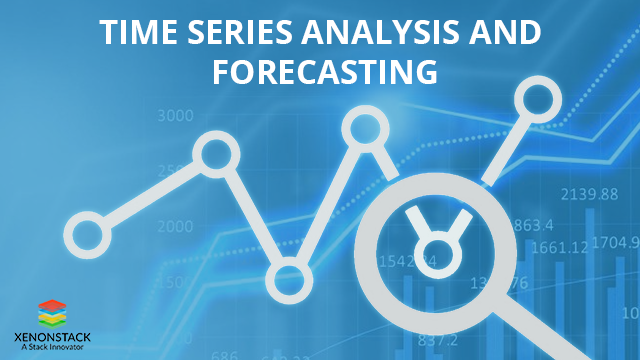
Introduction to Time Series Analysis
Time-Series refers to data recording at regular intervals of time. Time-Series components involve -- Trend - It increases, decreases or remains at a constant level with respect to time.
- Seasonality - It is the property of Time -Series to display periodical patterns which repeats at a constant frequency.
- Cycles - They do not repeat at regular time intervals and occur even if the frequency is 1.
Challenges to Time Series Forecasting
- Sensor Data or Stock Market data consists of ~250 variables ‘col1’,’col2’...etc. and the data size is 15 gb.
- Besides this, there are two critical categorical variables named ‘symbol,' ‘categ’ and another variable ‘time.'
- There are 22 unique symbols, and seven unique cats's in the whole dataset recorded for every minute.
- The target is to forecast ten future values of a column named ‘val’ for each symbol-categ pair.
Solution Offered for Time Series Forecasting
Approaches for Time Series Analysis
Approach 1 - Convert Time Series Problem to Supervised Learning Problem.- Convert Time-Series data to Supervised Learning data.
- Supervised Learning requires the values for all the independent variables.
- It is an extension of the one dimensional Autoregressive Method. The advantage of this method is, no need to convert the Time Series Data to Supervised Learning Data.
- Get the predictions for future values from the model itself. The model considers the interdependencies in the data.
Understanding Pattern Analysis and its Components
Pattern Analysis with Time Series Data involves nature identification represented by a sequence of observations and forecasting including prediction of future values Time- Series variable. Time Series Pattern Analysis consists of systematic pattern data called as a set of identifiable components and random noise error which makes pattern identification difficult.Components of Pattern Analysis
- Trend Analysis
- Seasonality Analysis