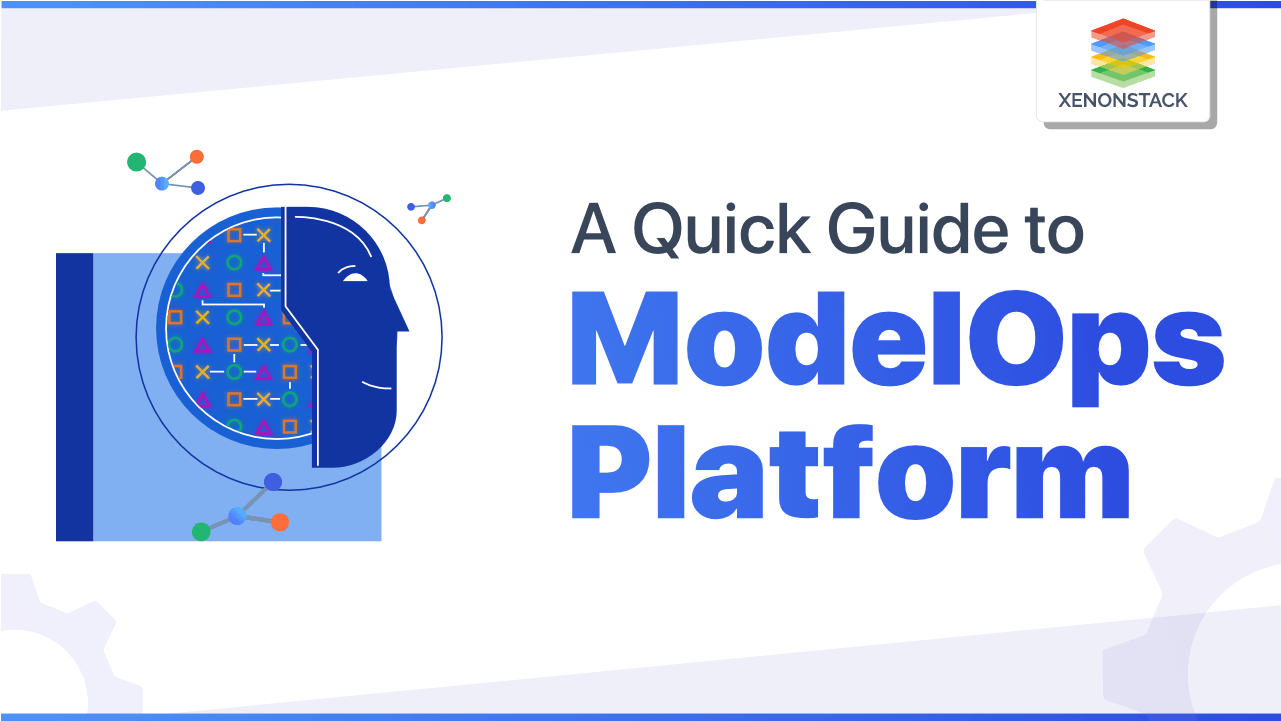
What is ModelOps?
Model Operations are at the center of every organization's enterprise AI approach. It enables technology to converge multiple AI objects, solutions, and AI frameworks while maintaining scalability and governance. A diverse set of Operationalized AI (Artificial Intelligence) and decision models, including machine learning, knowledge graphs, rules, optimization, and linguistic and agent-based models, are mostly focused on governance and life cycle management (or AI platform operationalization).
What are the types of ModelOps Processes?
Enterprises should ensure that they do the following to take benefit of ModelOps and drive transformative leadership.
Intelligent use of AI and DevOps Automation
Adopting AI life cycle management automation will help streamline the method of handling applications and models. Review or redesign an end-to-end process to drive continuous learning and handle models with a particular focus on training and output data. Utilizing AI will help automate core DevOps processes, such as automatic infrastructure maintenance and control, AI-driven automated testing and process enhancement, and AI-driven quality assurance.
DevOps and ModelOps synchronization
Synchronized application development and model life cycles will also help ensure that models are efficiently implemented in operating applications and that outputs get back to the retraining of models and the development of application upgrades.
What are the Technologies Involved in ModelOps?
Multi-Cloud Data and AI Architecture
Investing in data-driven, cloud-based infrastructure, and microservices will help the enterprises to enable the accelerated growth and rollout of software, as well as workload and model portability through several clouds.
Think Beyond Machine Learning Models
To achieve the full benefits of a model-based approach, make use of AI models outside machine learning, such as organizational models, optimization models, and transformational models, that will be helpful for enterprises.
AI Governance at Scale
It will help to ensure that models are governed, trusted, and explainable and contribute to the creation of implementations that are consistent with market priorities and legislation relevant to compliance and are scalable to adapt to evolving business needs on a scale. As enterprises scale up their AI initiatives to become a true Enterprise AI organizations, having full operationalized analytics capability puts ModelOps in the center
As enterprises scale up their AI initiatives to become a true Enterprise AI organization, having full operationalized analytics capability puts ModelOps in the center. Click to explore our, connecting both DataOps and DevOps.- Forbes
How to operationalize ModelOps?
Enterprises need to monitor the performance of the ModelOps software for the first phase of a ModelOps process. Since ModelOps is a growth, testing, rollout, and monitoring cycle, it will only be successful if it progresses against delivering the organization's size and accuracy. Then need to track each model's success at the organizational stage.
- For models (or model classes), set accuracy goals and monitor them for dimensions such as drift and degradation through development, validation, and deployment.
- Identify market indicators that are influenced in service by the model. Is a model designed to raise users, for instance, having a beneficial impact on subscription rates?
- Track metrics such as the size of data and update frequency, positions, groups, and forms. Model performance concerns are often attributable to changes in the data and its sources, and these metrics can assist in your investigation.
- Track the volume used by processing power or memory models.
Why is ModelOps Important?
Organizations also struggle with analytics operationalization because they lack a formal system to organize resources through analytics, IT, and the organization. Since data alone doesn't drive the business, decisions do. They are making decisions that impact organizations each day. Since analytically driven decisions are smarter choices, it helps them make the right choices every time, even while making thousands or millions of them every day, by integrating analytics into the decision-making processes. This includes the operationalization of scale analytics.
- The number of models - To account for business process variations, personalization, and specific customer groups, each organization will need to handle hundreds of models.
- Technology Complexity - The fast and continuing innovation in data & analytics contributes to unmanageable difficulty for even the most expert IT teams.
- Regulatory Compliance - As the use of AI spreads across markets, adhering to strict and ever-increasing models, regulatory criteria become more challenging.
- Organization Silos - Scaling AI in trends that are in businesses needs industry, technology, and data to come together. To ensure its free flow through the enterprise, the organizational data needs to be opened. This cannot occur in a siloed work culture, and organizations must develop an interdisciplinary team to push AI in the organization.
ModelOps (or AI model operationalization) is focused primarily on the governance and life cycle management of a wide range of operationalized artificial intelligence. Click to explore our, AI model operationalization - Gartner
How do industry leaders drive AI at scale?
Enterprises drive AI into core processes at scale by focusing on three areas:
- Model Centric Approach - Leaders manage models as first-class business properties, enabled by a model-centric infrastructure that facilitates long-term continuous implementation performance independent of data science workbench and execution platforms.
- Operations First Mentality - Leaders recognize that deploying models to operation systems allows them to run 24×7 without delay, using the same operating controls, software, and automation that enable other technologies.
- Automation - Leaders completely automate ModelOps processes, from implementation to monitoring and governance, to efficiently handle business model SLAs, eliminate manual execution, and mitigate risk, cost, and model time for business.
ModelOps vs MlOps
Model Operations performs model implementation and management during the application creation and deployment lifecycle to operate models in production.
In comparison to MLOps, which focuses exclusively on ML models' operationalization, and AIOps, which is AI for IT operations, It focuses on the operationalization of both AI and decision models.
In the past few years, we have seen how AI shifts from Data Science to building powerful AI models to figure out how to deploy models on production at scale. Many powerful tools can help train the models and use the right model for the specific use case. MLOps tools reduce the time to deploy models from months to a matter of hours. It provides powerful tools to monitor how the AI models perform in real time, but there is still a missing link between the teams in building and deploying AI. It provides transparency into how teams are deploying and using AI anywhere in the enterprise. This technology truly changes the current state of enterprises and provides explainability into AI-enabled outcomes.
Click to explore MLOps Challenges and Solutions while building and deploying Model
ModelOps Solutions that address challenges to help large enterprises
Major business companies have been speeding up their artificial intelligence and machine learning activities for the past few years with ModelOps. Organizations need to run their machine learning models around the enterprise to adapt models to hundreds of use cases.
Gartner researched that enterprises were planning to deploy AI twenty-three percent in their systems two years back, but they found that only five percent were deployed.
The companies were bringing an accumulating amount of "model debt" instead of real 24/7 production implementations of Enterprise AI adoptions. These are undeployed and unrefreshed models that leave businesses with millions on the table. Some investments are unrealized. If the business dynamics shift, they can never realize their returns if they don't move on these investments.
To compound the issue, as new capabilities such as AutoML arise, and industry demands further as market trends change, citizen data scientists have begun to implement bottom-line applications without enterprise IT controls.
The lack of accountability in these systems will harm governance, not to mention costs. Large-scale models also need governance during implementation and close oversight to ensure that they comply with the regulations.
ModelOps aims to delineate these problems. The ModelOps Life Cycle can be a data science partner working with various technologies in specific business divisions to tap into specific data repositories to address business issues better. Models are mostly generated from historical knowledge and then deployed on production data to work. For the full data science project team, ModelOp aims to operationalize the analysis performed in all contexts through the ModelOps Lifecycle.
ModelOps in the Insurance industry for decision-making?
In the insurance industry, algorithms are supervised to ensure that no redlining is happening. That can be complicated and add more uncertainty to the processes of decision-making. To ensure compliance, audit trails, scores, and monitoring reports must be produced. ITOps can use the CommandCenter during the whole model's life cycle to respond quickly to troubleshooting as required and rely on various resources to solve them.
After implementing ModelOps Life Cycle with ModelOps, some large enterprise customers are finding some new possibilities. A major multinational insurance corporation has recently arrived at ModelOp after successfully developing data science and computer learning technologies to upgrade their legacy models to machine learning models. To deploy their capabilities of data science and machine learning, they used ModelOps. They found that with this process, they could deploy a lot more models after using ModelOps. They improved the overall insurance policy decisioning by creating a full end-to-end policy adjustment process. Before that, they had no idea that it was feasible to establish a full end-to-end policy adjustment process. They got an opportunity for an Enterprise AI solution to boost their current business workflow until they used ModelOps.
How can ModelOps be beneficial for given use cases in the Insurance Industry?
- Insurance Advice: From handling the customer's first interaction to the completion of service, which includes the customer requirements made possible by machine learning algorithms that will review their profiles and recommend personalized products.
- Claim Processing: To automate and improve operational efficiency from the registration to the settlement of claims and enhance the customer experience.
- Fraud Prevention: To identify the claims faster and accurately, which are fraudulent. This will improve the productivity of the claim settlement process and improve fraud prevention.
- Risk management: To find out the losses and premiums for the policies, early identification of risks in the process helps insurers make better use of underwriters' time and provides them with a significant competitive advantage.
Conclusion
The ModelOps Life Cycle is relevant as more organizations are expanding their Enterprise AI initiatives. Probably soon, in the next few years, as more businesses use ModelOp to ramp up their Enterprise AI systems, companies will gradually see a major reduction in "model debt" across organizations.
What we do - To help you leverage the power of AI/ML on a scale that will improve your core processes, we have the expertise and solution. With ModelOps, we help our clients scale and control their enterprise AI initiatives.
-
Explore more about Machine Learning Observability and Monitoring
-
Learn more about MLOps Roadmap for Model's Interpretability