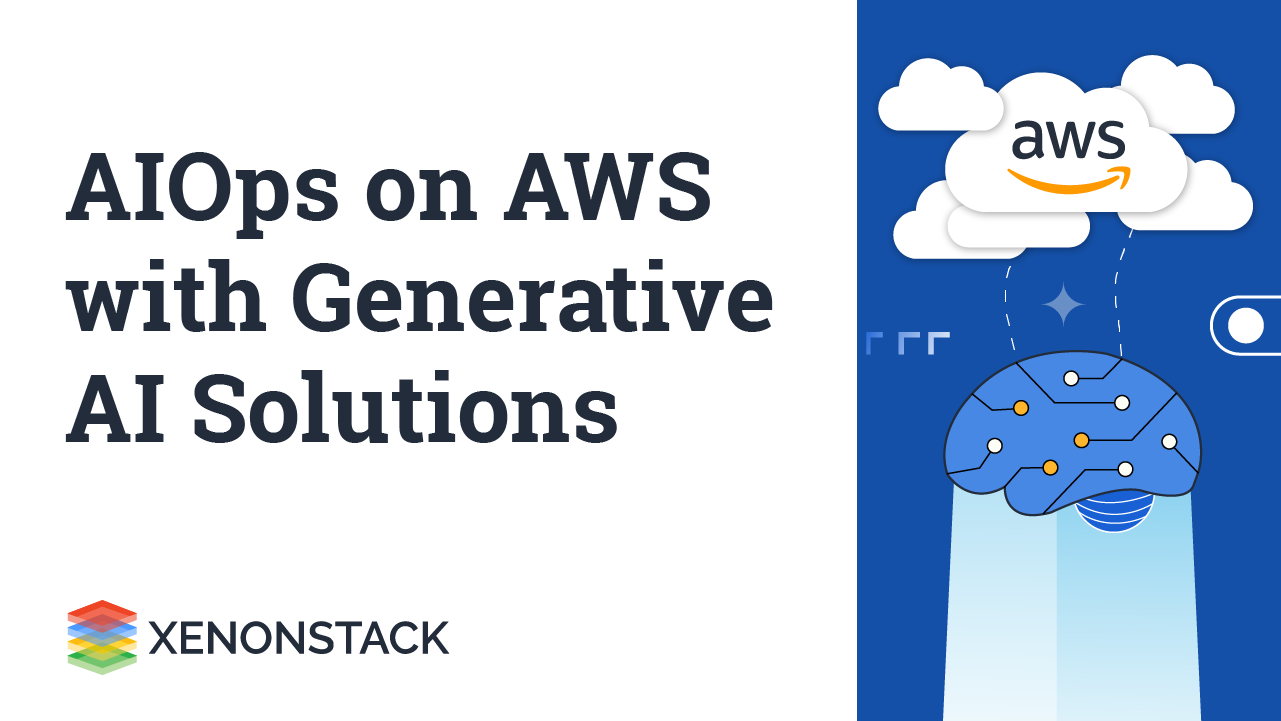
Introduction to AIOps and Anomaly Detection
The combination of artificial intelligence with IT management, or AIOps, represents a breakthrough paradigm in the field of contemporary IT operations. To start our investigation, we first examine the fundamental idea of AIOps, defining it as the incorporation of cutting-edge AI and machine learning technologies into the complex web of IT operations. This all-encompassing strategy promises increased effectiveness and dependability by automating, streamlining, and optimising the numerous tasks involved in IT management.
AIOps ecosystem component anomaly detection stands out as a key sentinel in this environment. Its importance is derived from its capacity to identify anomalies—differences from typical system behaviour. As we proceed through this blog, we highlight the crucial part that anomaly detection plays in protecting IT systems as well as spotting performance issues, potential security risks, and other things. We also emphasise the urgent need for improvements in anomaly detection strategies, emphasising the shortcomings of conventional methods considering the complex and dynamic IT environments of today.
What do you understand by AIOps?
AIOps is a set of practices combining artificial intelligence and machine learning with IT operations to enhance how organizations manage their IT systems. It leverages large datasets, real-time analysis, and automation to detect and resolve issues proactively, predict future problems, and optimize IT infrastructure. AIOps aims to reduce human intervention in the IT operations processes.
Foundations of Anomaly Detection
The basis of anomaly detection is the discovery of anomalies, which are data items or occurrences that dramatically depart from what is expected or "normal" behaviour in a certain environment. These anomalies can appear in a variety of ways, from quiet outliers to obvious deviations. The foundation for separating anomalous events from routine processes within complicated data sets is laid by having a clear understanding of what an anomaly is.
Anomalies themselves are not uniform, it becomes clear when exploring the field of anomaly detection. They take many different forms, therefore correctly classifying them is crucial for efficient identification. Point anomalies, contextual anomalies, and collective anomalies are the three primary categories of anomalies. Individual data points that considerably depart from the predicted range are referred to as point anomalies.
Contrarily, contextual anomalies are anomalies that are only regarded as anomalous when considering a larger context or set of circumstances. Finally, collective anomalies refer to a set of data points that, although they individually appear normal, behave abnormally when seen collectively. Being aware of these differences enables anomaly detection systems to adapt to different situations and data kinds.
While anomaly detection is a useful tool, conventional approaches frequently face serious obstacles. The imbalance between normal and anomalous data is one of the main problems. Anomalies are infrequent occurrences in many real-world circumstances, making it challenging to successfully train models.
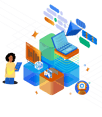
The purpose of Generative AI is to create content, as opposed to other forms of AI, which might be used for different purposes, such as analyzing data or helping to control a self-driving car.
Key Technologies in AIOps Anomaly Detection
The use of cutting-edge technology is crucial for the development of anomaly detection within the context of AIOps.
Machine Learning and Deep Learning
The use of Machine Learning (ML) and deep learning (DL) techniques is foremost among these. By recognising differences from established standards, these technologies enable AIOps systems to learn patterns and relationships across massive and complicated datasets, enabling them to detect anomalies. The accuracy of anomaly detection is further improved
by deep learning, in particular because of how well it handles complex, high-dimensional data thanks to its neural networks and hierarchical feature learning.
Unsupervised Learning Techniques
Techniques for unsupervised learning are a vital component of AIOps anomaly detection. In instances involving anomaly detection, labelled data, which are necessary for traditional supervised learning, may be limited. This problem is solved by unsupervised learning, which enables algorithms to recognise patterns and anomalies on their own without any prior instruction. In order to more effectively discover anomalies, techniques like clustering and dimensionality reduction are crucial in revealing hidden structures within data.
Time Series Analysis
Given its abundance in IT data, time series analysis emerges as a crucial tool in AIOps. Time series data, which records data over time, is a popular format for keeping track of user behaviour, network activity, and system performance. Algorithms must be able to represent temporal dependencies, trends, and seasonality to detect anomalies effectively. AIOps uses time series analysis methods including autoregressive models, moving averages, and recurrent neural networks to examine time-stamped data for deviations and identify them quickly so that anomalies may be addressed.
Natural Language Processing (NLP) for Log Analysis
AIOps encompasses both organised and unstructured data sources, such as logs produced by IT systems. When analysing these logs, Natural Language Processing (NLP) is essential. NLP algorithms interpret error messages, event summaries, and other log entries to extract useful information from textual input. AIOps systems can connect log events with system behaviours and perform more thorough and contextual anomaly detection by parsing and understanding this unstructured data.
These essential technologies are incorporated into AIOps anomaly detection, improving the system's ability to spot abnormalities and preparing it to adjust to changing IT environment complexities. AIOps is able to provide more precise, pro-active, and effective anomaly detection, which is essential for preserving the stability and security of contemporary IT systems. This is made possible by the synergy of Machine Learning, Unsupervised Learning, Time Series Analysis, and Natural Language Processing.
Enhancing Anomaly Detection with AIOps
Applying AIOps to improve anomaly detection requires a multifaceted strategy that goes beyond algorithmic selections.
Feature Engineering for Anomaly Detection
The process' crucial step is feature engineering. It involves choosing and manipulating pertinent data qualities to feed into algorithms for anomaly detection. Feature engineering in the context of AIOps could entail developing new features that accurately depict the subtleties of IT system behaviour. For instance, elements like packet loss rates, traffic peaks,
or latency patterns may be engineered in network monitoring to provide a more thorough perspective of network health. The discriminative ability of anomaly detection models is considerably increased through effective feature engineering, allowing them to identify even the smallest abnormalities with greater accuracy.
Model Selection and Hyperparameter Tuning
AIOps-driven anomaly detection relies heavily on both model selection and hyperparameter tuning. The best results come from models that are specifically designed for the various anomalies and data distributions. Depending on the type of data and the unique anomaly detection task, AIOps practitioners must carefully choose the appropriate algorithms, such as decision trees, support vector machines, or deep neural networks. Additionally, hyperparameter tuning entails perfecting these models' settings for optimum performance. This procedure is streamlined by automated tools and methods like grid search and Bayesian optimisation, which enable AIOps systems to dynamically adjust to shifting data patterns and enhance their anomaly detection skills.
Ensemble Techniques for Improved Accuracy
Anomaly detection models can be made more accurate and robust by using ensemble techniques. Ensembles reduce the chance of false positives and enhance overall detection performance by combining the predictions of various models. AIOps makes use of ensemble approaches, such as Random Forests, Gradient Boosting, or stacking, which combine the results of various models to provide a more thorough and trustworthy anomaly detection system. Ensembles are a useful tool in the AIOps toolbox since they are particularly successful in scenarios where multiple models excel at capturing different sorts of anomalies or where the data is highly unbalanced.
By incorporating these techniques, AIOps-driven anomaly detection improves its capacity to find anomalies in IT systems. Feature engineering makes sure that the right data is recorded, model selection and hyperparameter tuning optimise the algorithmic decisions, and ensemble techniques improve the detection system's overall accuracy and robustness. AIOps are better able to handle the complexity of contemporary IT environments thanks to this comprehensive methodology, which also produces more accurate and usable anomaly detection results.
Real-time Anomaly Detection
Real-time Anomaly Detection is a crucial AIOps feature because it enables quick detection of and reaction to anomalies as they happen, minimising potential disruptions and damages. Real-time detection does, however, provide a special set of difficulties. The sheer amount and speed of data that modern IT systems produce is one of the main problems. The speed at which data is created makes traditional batch processing approaches inadequate. Furthermore, compared to historical data analysis, anomalies frequently appear in real-time data streams as small deviations, making their discovery more difficult.
AIOps uses Stream Processing and Event Correlation to handle these problems. AIOps systems can ingest and analyse data as it flows in real-time thanks to stream processing technologies. These systems use algorithms that have incredibly fast data processing and decision-making capabilities. Using event correlation algorithms, the system can make connections between occurrences that at first glance seem unconnected, possibly revealing intricate abnormalities that could otherwise go undetected. AIOps can immediately identify anomalies in the data stream by continually monitoring and correlating events in real-time.
Rapid real-time analysis is made possible by in-memory computing. Faster processing and access to data are made possible by storing it in memory rather than on disc. This is essential for real-time anomaly detection since it gives the system the ability to handle high data ingestion rates and carry out intricate calculations instantly. When working with massive data streams, in-memory computing is very useful because it reduces latency and makes sure that any potential anomalies are found and addressed right away.
In summary, a key component of AIOps is real-time anomaly detection, which enables prompt reactions to new problems. The speed and volume of data present problems, but AIOps uses event correlation, stream processing, and in-memory computing to overcome them. AIOps gives businesses the tools they need to quickly spot and address anomalies in their IT systems, enhancing system resilience and reducing interruptions.
Network Anomalies Detection
The concept of AIOps has become a major factor in network management. AIOps technologies excel in crucial applications like Network Anomalies Detection. AIOps systems can spot out of the ordinary patterns in network traffic that can indicate network problems or security breaches. abnormalities include things like abrupt increases in traffic, odd data packet patterns, and abnormalities in communication protocols. A case study may demonstrate how an enormous company used AIOps to quickly recognise and fix a serious network issue that could have resulted in pricey downtime. The organisation was able to significantly reduce network-related problems and enhance overall network performance by employing machine learning and real-time analysis.
Application Performance Monitoring
AIOps has completely changed how businesses manage and enhance their software systems in the area of Application Performance Monitoring (APM). Case studies can highlight how AIOps technologies can uncover performance bottlenecks automatically, identifying problems like poor response times, memory leaks, or inefficient database queries. Machine learning techniques and real-time monitoring can detect future performance degradation before it influences end users. Companies can discuss their experiences and how AIOps technologies have helped them maintain high application availability and make sure that their software services continue to function without interruption even during periods of heavy demand. These case studies highlight the importance of AIOps in sustaining business continuity and providing great user experiences.
Security and Threat Detection
In today's connected world, security is critical, and AIOps is essential to bolstering an organization's defences. Case studies in the security and threat detection space can demonstrate how AIOps can quickly recognise and address security events. For instance, a case study might show how, even before conventional security technologies had identified the breach, an AIOps-driven security system discovered a sophisticated cyber attack by examining patterns of odd behaviour. AIOps can also aid in the hunt for hidden dangers by sifting through vast amounts of log and event data. These case studies highlight AIOps as a pro-active security partner who speeds up threat detection and response while strengthening organisations' cyber-security posture.
Overall, these case studies demonstrate concrete examples of how AIOps is applied in real-world situations and highlight the effects it has on network management, application performance, and security. They act as effective examples of how AIOps may improve IT operations and guarantee the dependability, performance, and security of contemporary digital ecosystems.
Generative AI Solutions
Generative AI, a subset of AI, focuses on creating data rather than just processing it. It has applications in various fields, including natural language processing, image generation, and even code generation. In the context of AIOps, Generative AI can help generate synthetic data, analyze complex patterns, and predict future system behaviors.
Benefits of Implementing AIOps on AWS with Generative AI Solutions
Generative AI is a new and rapidly evolving technology that significantly impacts the field of AIOps. Artificial intelligence (AI) models trained on large data sets of IT data to learn the expected behavior of IT systems and infrastructure. This knowledge can then be used to identify anomalies, predict failures, and generate insights that can help IT teams improve their operations.
There are some significant benefits to implementing AIOps on AWS with generative AI solutions. One of the most important benefits is that it can help to improve IT efficiency and productivity. Generative AI can automate many repetitive and time-consuming tasks involved in AIOps, such as monitoring logs, detecting anomalies, and correlating events. This can free up IT staff to focus on more strategic and essential tasks, such as improving the customer experience and developing new products and services. Some of them are discussed below:
Proactive Issue Resolution
AIOps, powered by Generative AI, can identify potential issues before they impact system performance. Analyzing historical data and patterns can suggest preventive actions, reducing downtime and ensuring a seamless user experience.
Cost Optimization:
AWS provides a pay-as-you-go model, and AIOps can help organisations optimize their cloud spending by identifying underutilised resources, suggesting rightsizing, and automating resource allocation.
Efficient Resource Management
Generative AI can analyze resource usage patterns and recommend adjustments to resource allocation in real-time, ensuring that you're only paying for what you need.
Improved Security
AIOps can enhance security by continuously monitoring network traffic and identifying potential security breaches or anomalies. Generative AI can also generate synthetic data for security testing, helping to fortify your defences.
Predictive Maintenance
AIOps can predict when hardware components will likely fail based on historical data and usage patterns, allowing for proactive maintenance and minimizing unplanned downtime.
Overcoming Challenges in AIOps Anomaly Detection
The quality and readiness of the data is one of the key issues with AIOps anomaly detection. As a result, it can be challenging for AIOps systems to distinguish true anomalies from data artefacts. Real-world data is frequently noisy, fragmentary, or inconsistent. To solve these problems, data preparation techniques are needed.
To prepare the data for analysis, this stage entails cleaning, converting, and normalising it. For instance, standardising and parsing logs from diverse sources may be necessary for log-based anomaly detection to extract useful data. Case studies in this field can show how businesses have dealt with problems with data quality and emphasise the value of data preparation for accurate anomaly identification.
Class imbalance, when regular instances vastly outnumber anomalous ones, is another ongoing problem. This could result in a biased model that does poorly with rare anomalies and is biased towards normal behaviour. This is addressed by AIOps using methods like oversampling, undersampling, or specialised algorithms made for datasets with imbalances.
Case studies could show how businesses effectively overcame problems with class imbalance in their anomaly detection initiatives, leading to increased accuracy in spotting both frequent and uncommon anomalies. These illustrations highlight how easily AIOps techniques may be adjusted to various data distributions.
AIOps anomaly detection may face extra challenges from seasonality and data trends. IT systems, for example, frequently display cyclical patterns, such as daily or weekly consumption trends. Systems for AIOps must distinguish between normal seasonal changes and actual abnormalities.
Case studies can highlight how businesses successfully modelled and accounted for seasonality and trends by utilising advanced time series analysis techniques. These findings may shed light on how AIOps systems may differentiate between normal oscillations and abnormal behaviours, ultimately resulting in more precise anomaly identification and fewer false alarms.
In a nutshell while challenging, these anomaly detection challenges in AIOps are not insurmountable. Case studies that illustrate effective tactics and methods for overcoming these difficulties not only show the effectiveness of AIOps but also offer insightful information for businesses seeking to integrate anomaly detection into their IT operations.
They emphasise the significance of handling data properties including seasonality and trends, class balance, and data quality to accomplish robust and accurate anomaly detection in dynamic IT settings.
Ethical and Privacy Considerations
As AIOps continues to change IT operations, it also raises several ethical and privacy issues that require careful consideration. The primary issue with these worries is data privacy. AIOps significantly relies on enormous volumes of data, which frequently contain sensitive data about specific people or organisations. It is crucial to ensure the proper handling and protection of this data.
To protect individual privacy rights and stop unauthorised access or data breaches, organisations employing AIOps must abide by strict data privacy laws and best practices, such as GDPR or HIPAA.
Additionally, important ethical factors in anomaly identification include bias and fairness. AIOps models gain knowledge from biased past data. Biased models can reinforce unfair treatment by marking particular groups or people more frequently than others as abnormal, for example. This may result in unfair results, which is not only unethical but may also have legal repercussions.
Fairness in anomaly detection must be ensured by addressing bias in AIOps models through thorough data curation, algorithmic fairness strategies, and constant monitoring.
Organisations must uphold the core values of accountability and transparency in their AIOps practices. It is imperative to comprehend how AIOps systems make decisions for both moral and useful reasons. Better audibility is made possible by transparent models and processes, which guarantees that system decisions are explicable and warranted.
Furthermore, for responsible AIOps adoption inside an organisation, defining clear lines of accountability is essential. AIOps systems should be designed, deployed, and overseen by teams and individuals who are prepared to address any potential ethical or privacy-related issues.
Organisations can use the power of AIOps while upholding moral principles and protecting privacy rights by proactively addressing these issues.
AIOps on AWS with Generative AI
Data Collection and Integration
Start by collecting and centralizing all relevant data sources, such as logs, metrics, and performance data, into AWS storage solutions like Amazon S3 or Amazon RDS.
Integrate monitoring tools like Amazon CloudWatch to provide real-time data on your AWS resources.
Data Preprocessing
Use Generative AI solutions to preprocess data, clean it, and prepare it for analysis. This step is crucial for accurate insights.
Pattern Recognition
Employ machine learning algorithms to recognize patterns and anomalies in your data. Generative AI can help uncover hidden patterns that may not be apparent through traditional analysis.
Predictive Analytics
Develop predictive models that can forecast potential issues or resource requirements. AWS offers services like SageMaker for building and deploying machine learning models.
Synthetic Data Generation
Utilize Generative AI to create synthetic data for testing and development purposes. This is particularly valuable for security testing and ensuring your AIOps system remains robust.
Continuous Monitoring and Improvement
AIOps is an ongoing process. Continuously monitor the performance of your AIOps system and refine it as needed to adapt to changing conditions and new data patterns.
Future Trends in AIOps and Anomaly Detection
AIOps and Anomaly Detection are expected to continue to develop and innovate in the future. We may anticipate more advanced algorithms and models as AIOps technologies develop, providing even deeper insights into the behaviours of IT systems. These developments will make AIOps systems more dynamically adapt to shifting IT environments, improving their ability to detect abnormalities and manage IT operations.
For instance, the incorporation of reinforcement learning strategies, which enable systems to learn from their experiences and advance, could result in AIOps solutions that are smarter and more independent.
The seamless integration of AIOps with both DevOps and SecOps practices is a big trend that is on the horizon. While SecOps deals with security, DevOps focuses on simplifying development and operations.
By offering a holistic picture of IT operations, development, and security, AIOps connects these disciplines. Through this connection, anomaly detection is made to become more than just a stopgap solution in the development and security lifecycles.
This development is essential for assuring early anomaly identification and prevention, improving system dependability, and supporting cyber security initiatives.
Autonomous incident management and remediation are AIOps' ultimate objectives. This means that AIOps systems won't just find anomalies; they'll also deal with them automatically, without help from people. For instance, the system might automatically assign more resources to ensure optimal performance when a performance anomaly is discovered.
AIOps might also isolate compromised systems and launch incident response methods in the case of security risks. Rapider incident response, decreased downtime, and improved system resilience will result from autonomous remediation.
AIOps and anomaly detection have tremendous prospects for the future, to sum up. Organisations may anticipate more proactive, effective, and dependable IT operations thanks to the development of AIOps technologies, greater integration with DevOps and SecOps practises, and the shift towards autonomous remediation. In addition to improving anomaly detection skills, these trends also help modern IT environments be more agile and secure.